Exploring the role of the long short‐term memory model in improving multi‐step ahead reservoir inflow forecasting
Journal of Flood Risk Management(2022)
摘要
Daily inflow forecasting is of vital importance in reservoir economic operation. In the context of hydrometeorological forecasting, the effectiveness of the data-driven models has been demonstrated as bias correctors for physically-based models or direct forecasting models. However, existing studies only highlight the performance improvements provided by the data-driven model, lacking a comprehensive investigation on whether the data-driven model should be used as bias correctors or direct forecasting models. This study constructs long short-term memory (LSTM)-based preprocessing and postprocessing techniques for a hydrological model, which are tested by linear scaling preprocessing and autoregressive (AR) postprocessing models. The integrated model is compared with the LSTM-only model. The Shuibuya and Zuojiang reservoirs in China are selected as case studies. Results indicate that: (1) LSTM-based bias correctors are effective in both preprocessing and postprocessing and (2) the integrated model is comparable to the LSTM-only model when trained with four or more years of data, while it is better than the LSTM-only model when trained with less data. These findings demonstrate that data-driven methods can effectively correct the bias in physically-based model output, and integrating the physical and data-driven models is useful in improving multi-step ahead reservoir inflow forecasting if limited data can be obtained.
更多查看译文
关键词
hydrological modeling, long short-term memory, postprocessing, preprocessing, reservoir inflow forecasting
AI 理解论文
溯源树
样例
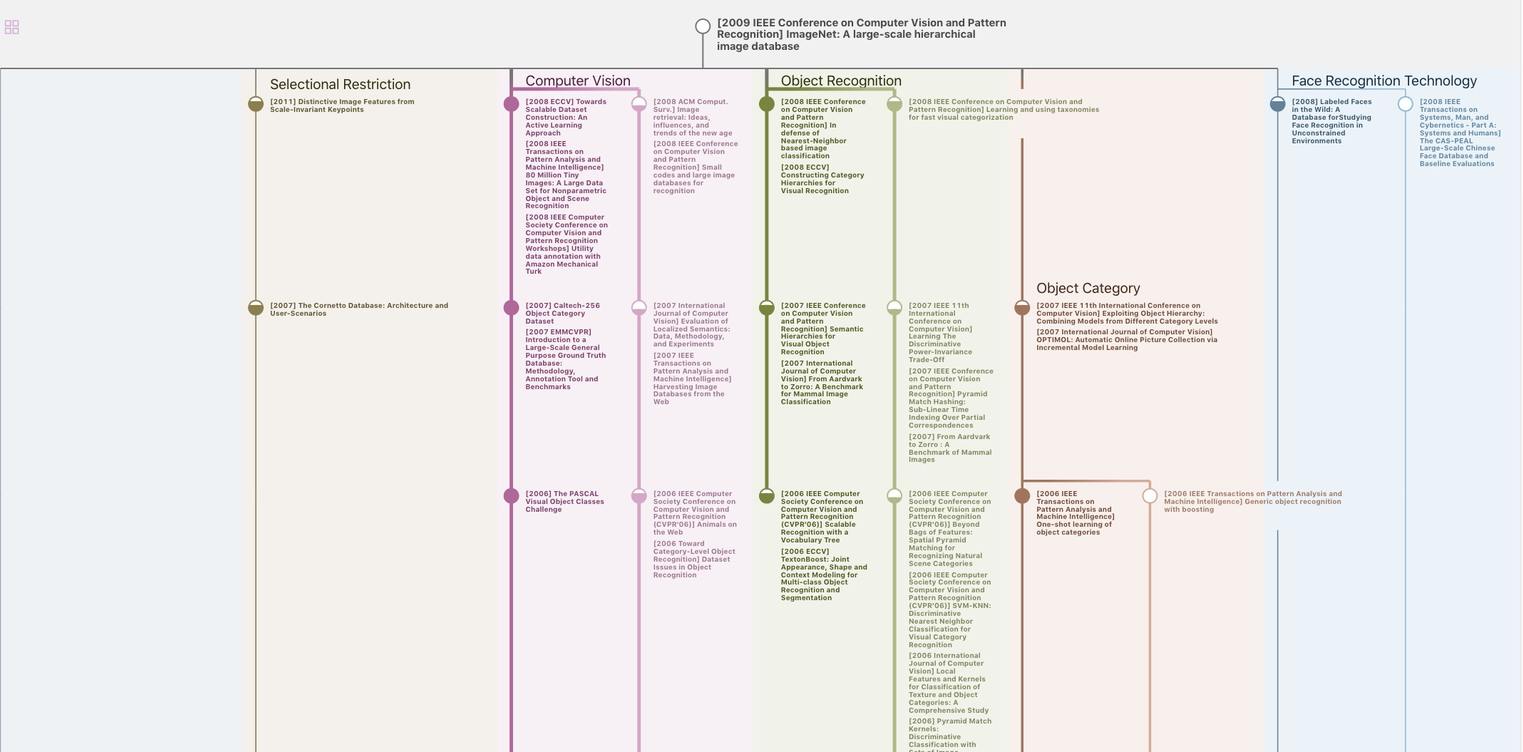
生成溯源树,研究论文发展脉络
Chat Paper
正在生成论文摘要