Weighted Verification Tools to Evaluate Univariate and Multivariate Probabilistic Forecasts for High-Impact Weather Events
arxiv(2023)
摘要
To mitigate the impacts associated with adverse weather conditions, meteorological services issue weather warnings to the general public. These warnings rely heavily on forecasts issued by underlying prediction systems. When de-ciding which prediction system(s) to utilize when constructing warnings, it is important to compare systems in their ability to forecast the occurrence and severity of high-impact weather events. However, evaluating forecasts for particular out-comes is known to be a challenging task. This is exacerbated further by the fact that high-impact weather often manifests as a result of several confounding features, a realization that has led to considerable research on so-called compound weather events. Both univariate and multivariate methods are therefore required to evaluate forecasts for high-impact weather. In this paper, we discuss weighted verification tools, which allow particular outcomes to be emphasized during forecast evaluation. We review and compare different approaches to construct weighted scoring rules, both in a univariate and multivariate setting, and we leverage existing results on weighted scores to introduce conditional probability integral transform (PIT) histograms, allowing forecast calibration to be assessed conditionally on particular outcomes having oc-curred. To illustrate the practical benefit afforded by these weighted verification tools, they are employed in a case study to evaluate probabilistic forecasts for extreme heat events issued by the Swiss Federal Office of Meteorology and Climatology (MeteoSwiss).
更多查看译文
关键词
Forecast verification, skill, Probability forecasts, models, distribution, Statistical forecasting, Model comparison, Postprocessing
AI 理解论文
溯源树
样例
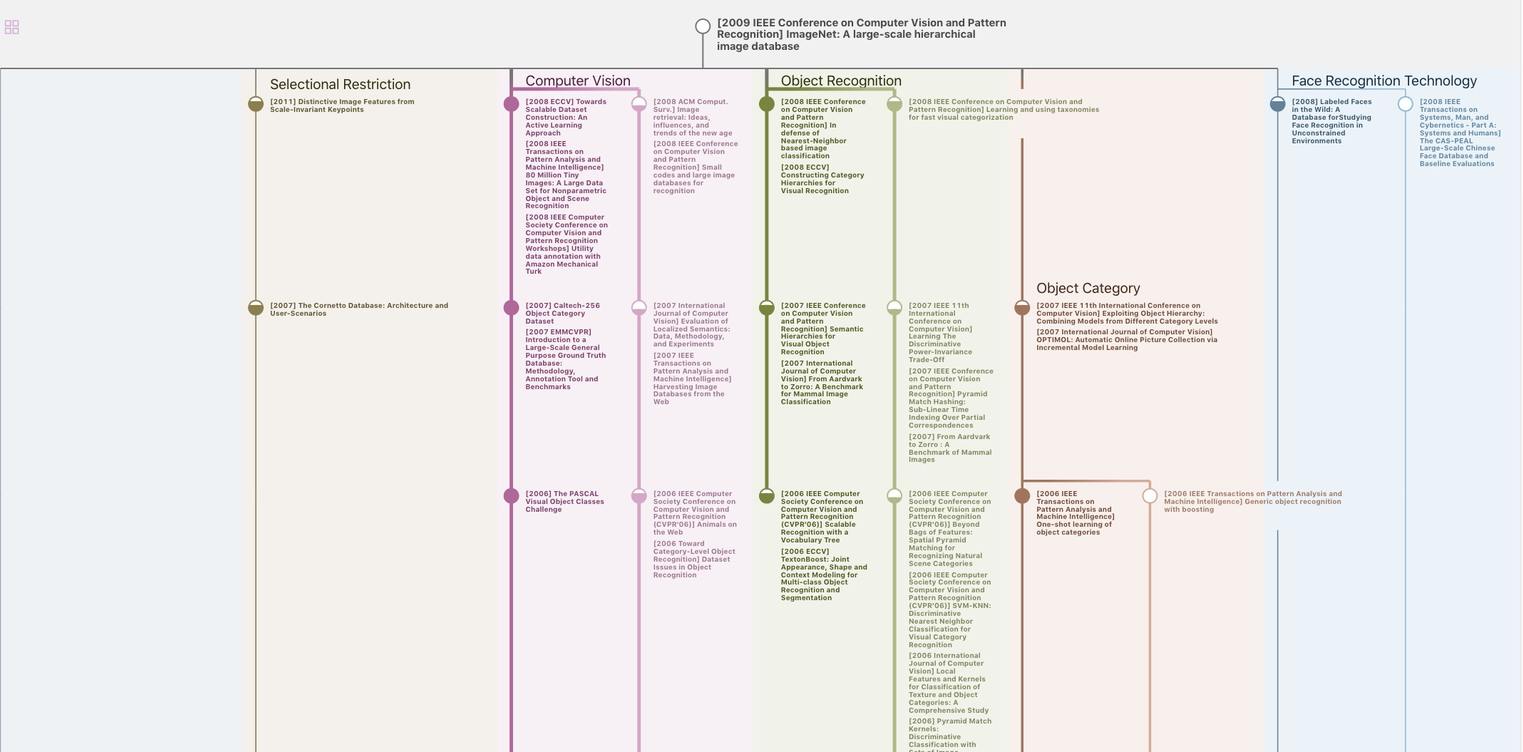
生成溯源树,研究论文发展脉络
Chat Paper
正在生成论文摘要