A Meta-Transfer Learning Approach to ECG Arrhythmia Detection.
Annual International Conference of the IEEE Engineering in Medicine and Biology Society (EMBC)(2022)
摘要
Automatic classification of cardiac abnormalities is becoming increasingly popular with the prevalence of ECG recordings. Many signal processing and machine learning algorithms have shown the potential to identify cardiac ab-normalities accurately. However, most of these methods heavily rely on a large amount of relatively homogeneous datasets. In real life, chances are that there is not enough data for a specific category, and regular deep learning may fail in this scenario. A straightforward intuition is to use the knowledge learned from previous data to solve the problem. This idea leads to learning-to-learn: extrapolating the knowledge accumulated from the old dataset and using it in a different but somewhat related dataset. In this way, we do not need to have considerable data to learn the new task because the underlying features of the old and new datasets resemble one another. In this paper, a novel machine learning method is introduced to solve the ECG arrhythmia detection problem with a limited amount of data. The proposed method combines the popular techniques of meta-learning and transfer learning. It is shown that our method achieves much higher accuracy in ECG arrhythmia classification with a few data and learns the new task faster than regular deep learning.
更多查看译文
关键词
learning,meta-transfer
AI 理解论文
溯源树
样例
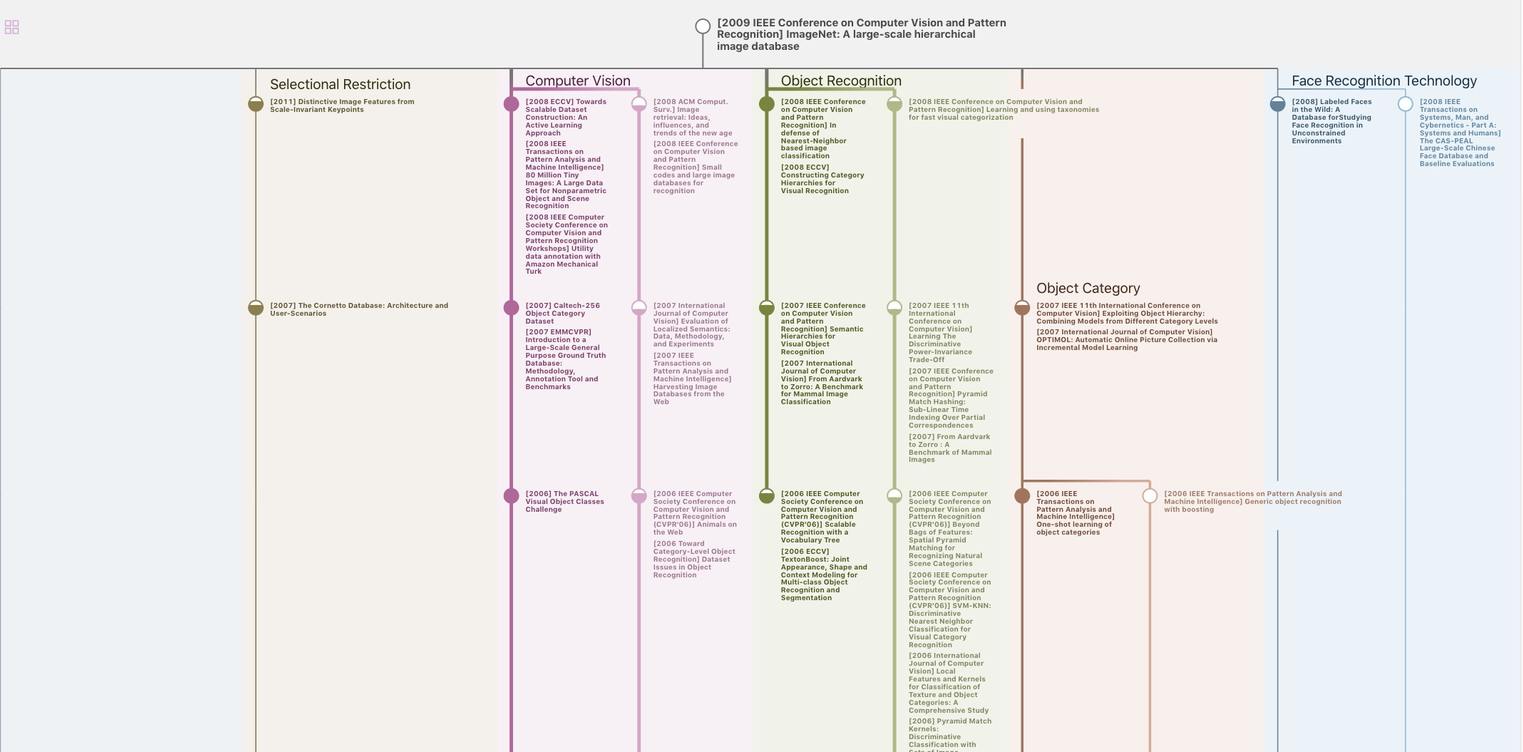
生成溯源树,研究论文发展脉络
Chat Paper
正在生成论文摘要