Taxonomical classification of reef fish with broadband backscattering models and machine learning approaches.
The Journal of the Acoustical Society of America(2022)
摘要
Commercially available broadband echosounders have the potential to classify acoustic targets based on their scattering responses, which are a function of their species-specific morphological and physiological properties. This is particularly important in complex environments with biologically diverse fish assemblages. Using theoretical acoustic scattering models among 130 fishes across six species, we examine the potential to classify reef fish based on the fine-scale gas-bearing swim bladder morphology quantified from three-dimensional computed-tomography models. Modeled echoes of the swim bladder for an incident broadband sound source (30-200 kHz) and across a range of orientation angles (±44°) are acoustically simulated using the boundary element method. Backscatter models present characteristics that are consistent within species and distinguishable among them. Broadband and multifrequency echoes are classified and compared with Bayesian, support vector machine, k-nearest neighbor, and convolutional neural network estimators. Classifiers have higher accuracies (>70%) when noise is not present and perform better when applied to broadband spectra than multifrequency data (42, 70, 100, 132, 160, 184 kHz). The modeling and classification approaches presented indicate that a taxonomic distinction based on morphologically dependent scattering responses is possible and may provide the capacity to acoustically discriminate among fish species.
更多查看译文
关键词
reef fish,taxonomical classification,broadband backscattering models
AI 理解论文
溯源树
样例
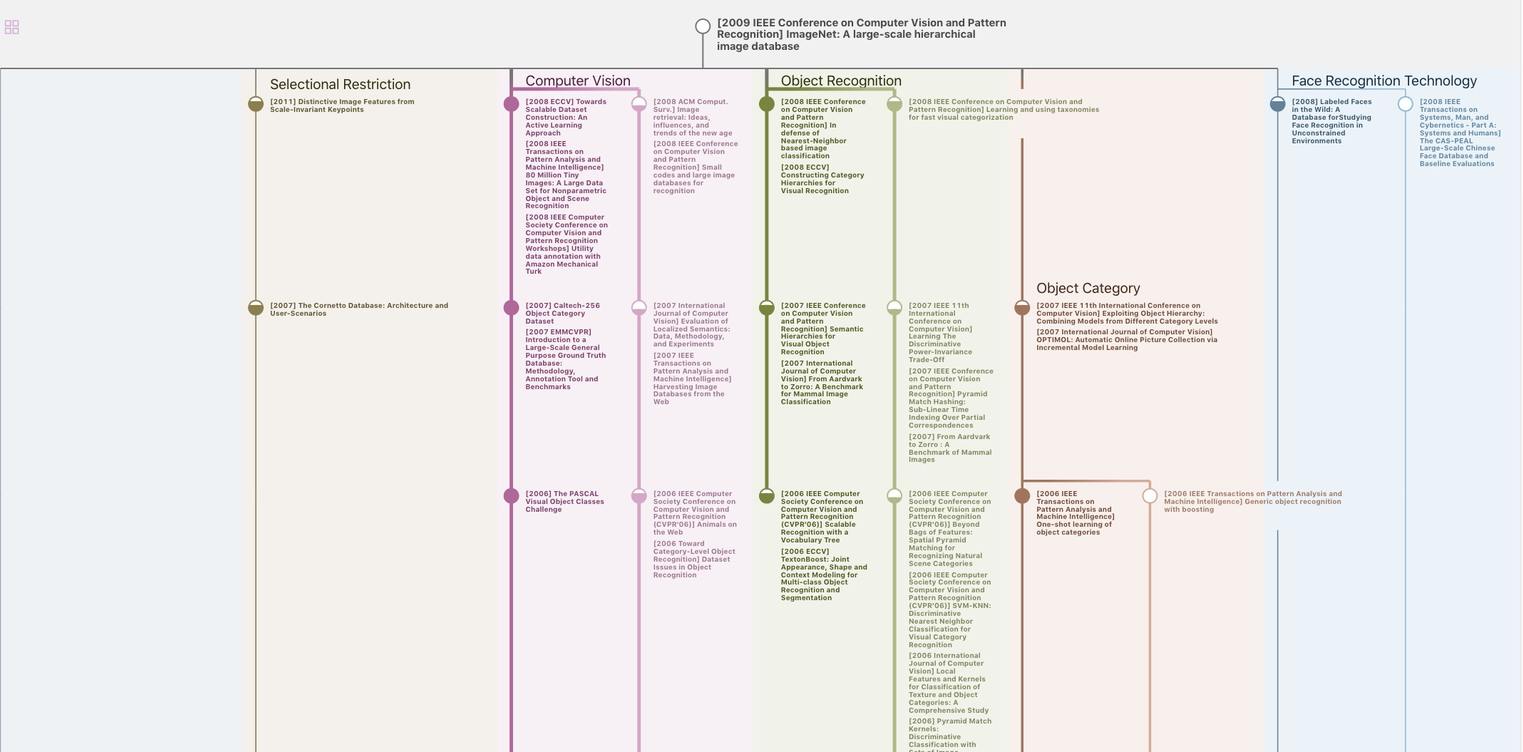
生成溯源树,研究论文发展脉络
Chat Paper
正在生成论文摘要