Stacked auto-encoders based visual features for speech/music classification
Expert Systems with Applications(2022)
摘要
With the rapid rise of online available content, multimedia signal processing has become an important area of research. The output of the speech/music classifier (SMC) is further used for both speech and music signal processing. Most of the works in the past two decades are focused on conventional classifiers mostly using shallow structures. This work evaluates the performance of stacked auto-encoders based deep neural network for designing a speech/music classifier on S&S and GTZAN dataset using visual features. The hidden layers of the neural network are initially trained in unsupervised manner using auto-encoders and are stacked with the final softmax layer. Different experiments were conducted on time-frequency features derived from Spectrogram and Chromagram. Performances of the combination of stacked auto-encoder and softmax classifier was further compared with traditional classifiers and different deep learning techniques. Best classification accuracy of 93.05% and 94.73% is observed for fused features for S&S and GTZAN datasets respectively.
更多查看译文
关键词
Speech/Music classifier,Auto-encoders,Time-frequency Visual features,Deep-learning
AI 理解论文
溯源树
样例
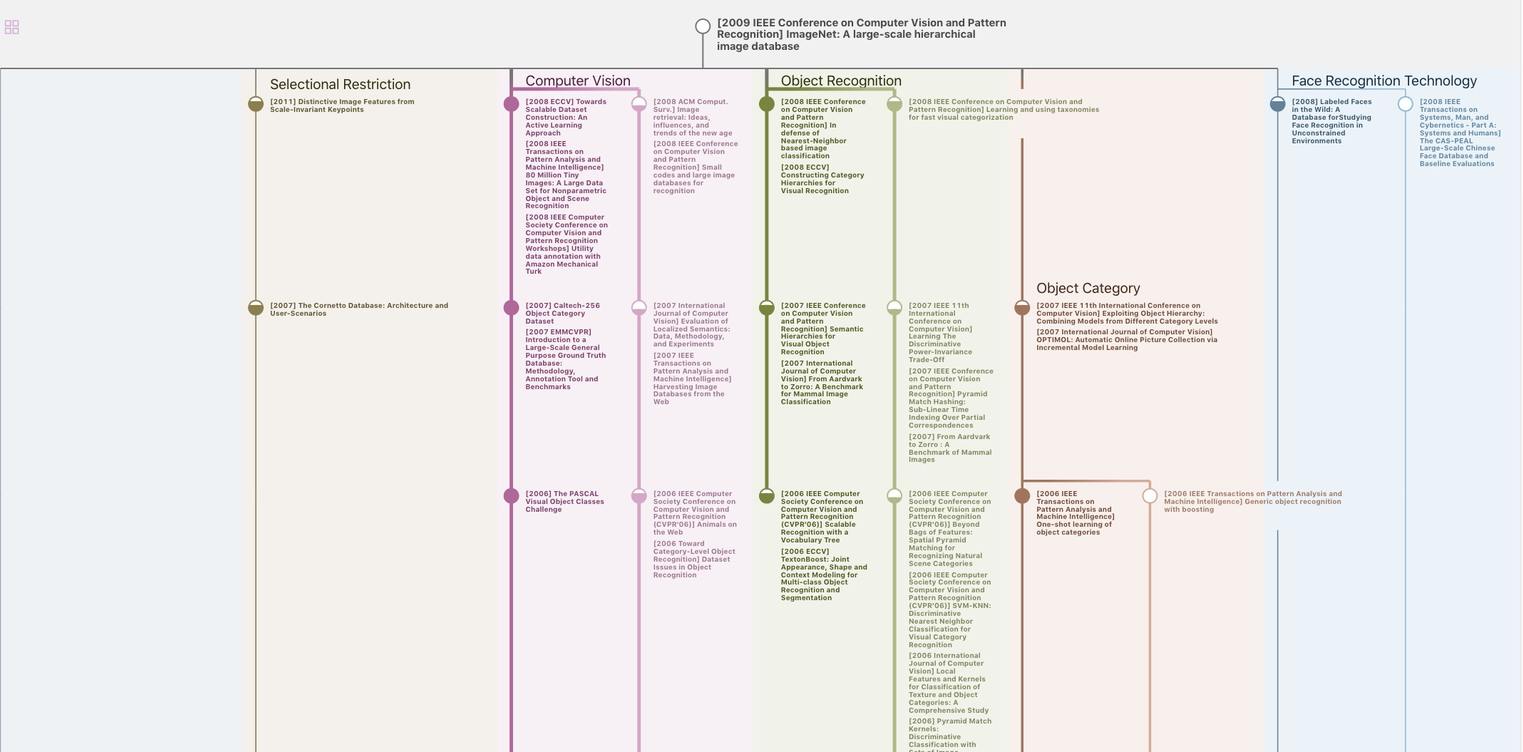
生成溯源树,研究论文发展脉络
Chat Paper
正在生成论文摘要