A new intrusion detection system based on using non-linear statistical analysis and features selection techniques
Computers & Security(2022)
摘要
The increase in the number of connected devices to the Internet and Internet of Things (IoT) development accompanied a massive increase in the number and types of attacks. Most IoT devices have security vulnerabilities due to their limited computing and storage capabilities and specific protocols. Thus, there is essential to build Intrusion Detection Systems (IDSs) that can detect these threats that affect smart applications. Researchers examined different data mining techniques, statistical analysis techniques, and machine learning (ML) algorithms. In this paper, we propose a novel approach for building an anomaly-based intrusion detection system based on using a non-linear statistical analysis technique called recurrence quantification analysis (RQA). Our approach uses RQA to identify abnormal behavior in an individual feature extracted from a series of packets rather than inspecting the packet's payload. The proposed procedure implies finding the minimum number of features, applying the RQA technique to each effective feature separately, and applying different ML algorithms to classify the RQA measurements resulting from each effective feature. The system's performance was evaluated based on the accuracy and F-score using the UNSW-NB15 dataset. Results show our proposed approach's effectiveness in discovering hidden characteristics in the underlying series of an individual feature that leads to identifying different attacks. Besides, the proposed approach outperforms most previous works using one feature.
更多查看译文
关键词
Intrusion detection system,Network threats,IoT security,UNSW-NB15 dataset,Recurrence quantification analysis,Machine learning algorithms
AI 理解论文
溯源树
样例
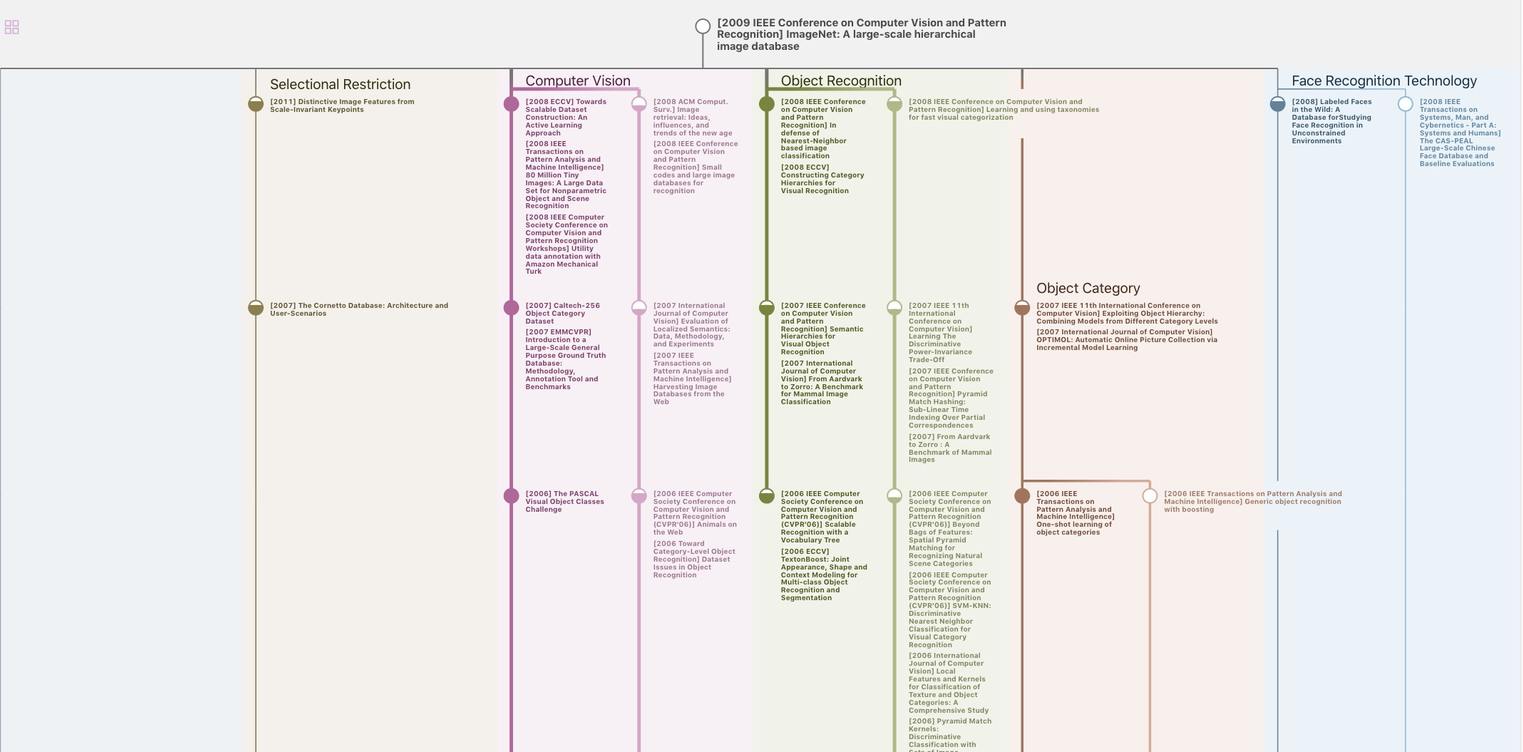
生成溯源树,研究论文发展脉络
Chat Paper
正在生成论文摘要