Collaborative clustering parallel reinforcement learning for edge-cloud digital twins manufacturing system
China Communications(2022)
摘要
To realize high-accuracy physical-cyber digital twin (DT) mapping in a manufacturing system, a huge amount of data need to be collected and analyzed in real-time. Traditional DTs systems are deployed in cloud or edge servers independently, whilst it is hard to apply in real production systems due to the high interaction or execution delay. This results in a low consistency in the temporal dimension of the physical-cyber model. In this work, we propose a novel efficient edge-cloud DT manufacturing system, which is inspired by resource scheduling technology. Specifically, an edge-cloud collaborative DTs system deployment architecture is first constructed. Then, deterministic and uncertainty optimization adaptive strategies are presented to choose a more powerful server for running DT-based applications. We model the adaptive optimization problems as dynamic programming problems and propose a novel collaborative clustering parallel Q-learning (CCPQL) algorithm and prediction-based CCPQL to solve the problems. The proposed approach reduces the total delay with a higher convergence rate. Numerical simulation results are provided to validate the approach, which would have great potential in dynamic and complex industrial internet environments.
更多查看译文
关键词
edge-cloud collaboration,digital twins,job shop scheduling,parallel reinforcement learning
AI 理解论文
溯源树
样例
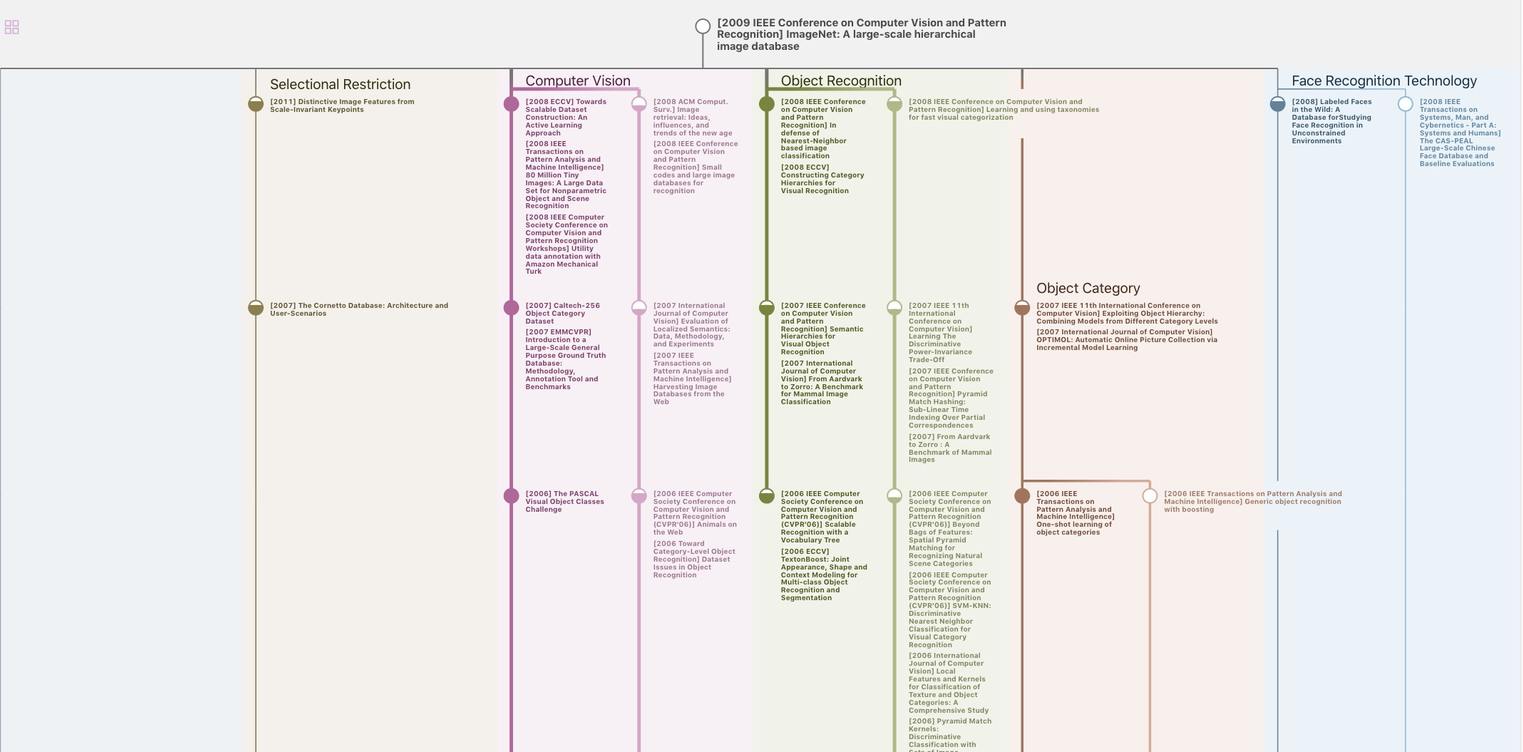
生成溯源树,研究论文发展脉络
Chat Paper
正在生成论文摘要