An Ameliorated Denoising Scheme Based on Deep Learning for Φ-OTDR System With 41 km Detection Range
IEEE Sensors Journal(2022)
摘要
In recent years, denoising methods for improving the performance of the phase-sensitive optical time-domain reflectometry (
$\Phi $
-OTDR) system have been restricted by the deficiencies of time-consuming and limited denoising effect. In this work, a trained convolutional neural network (CNN)-based image denoising model is proposed to greatly eliminate the unwanted noises in the
$\Phi $
-OTDR-based sensing system. First, the given Rayleigh backscattering traces are acquired and preprocessed through adjacent differentiation and two-dimensionalization. Second, the 2-D preprocessed data are converted into a noisy gray-scale image and sent into the CNN model for training and testing. Third, the CNN model outputs a corresponding denoised gray-scale image, which can be further analyzed by reconverting it into a series of denoised Rayleigh backscattering traces. Finally, a series of experiments are carried out to demonstrate the effectiveness of the proposed denoising scheme. Experimental results show that, in allusion to the vibration signal with different intensities along the 41-km optical sensing fiber, the trained CNN model achieves a signal-to-noise ratio (SNR) enhancement of about 20 dB. Compared with the conventional methods based on wavelet and empirical mode decomposition (EMD), the proposed denoising scheme demonstrates characteristics of robustness, well spatial resolution reservation, and high efficiency. It is believed that the trained CNN model has great potential to be deployed on the
$\Phi $
-OTDR system for real-time denoising.
更多查看译文
关键词
Convolutional neural network (CNN),distributed fiber optic vibration sensing,image denoising,long-range detection,perimeter security,phase-sensitive optical time-domain reflectometry (Φ-OTDR),signal processing
AI 理解论文
溯源树
样例
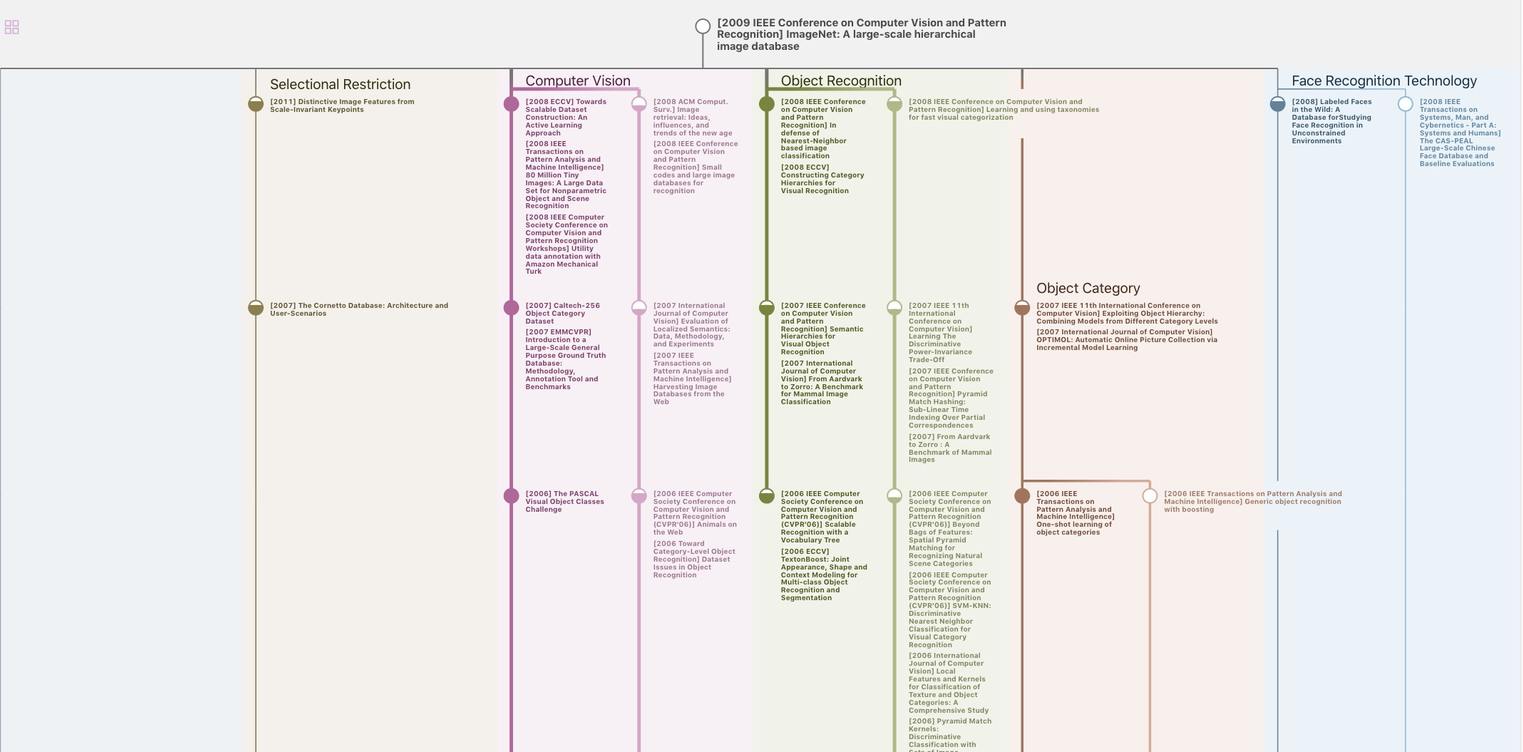
生成溯源树,研究论文发展脉络
Chat Paper
正在生成论文摘要