Extracting Relations Between Sectors
2022 IEEE/ACM International Conference on Big Data Computing, Applications and Technologies (BDCAT)(2022)
摘要
The term "sector" in professional business life is a vague concept since companies tend to identify themselves as operating in multiple sectors simultaneously. This ambiguity poses problems in recommending jobs to job seekers or finding suitable candidates for open positions. The latter holds significant importance when available candidates in a specific sector are also scarce; hence, finding candidates from similar sectors becomes crucial. This work focuses on discovering possible sector similarities through relational analysis. We employ several algorithms from the frequent pattern mining and collaborative filtering domains, namely negFIN, Alternating Least Squares, Bilateral Variational Autoencoder, and Collaborative Filtering based on Pearson’s Correlation, Kendall and Spearman’s Rank Correlation coefficients. The algorithms are compared on a real-world dataset supplied by a major recruitment company, Kariyer.net, from Turkey. The insights and methods gained through this work are expected to increase the efficiency and accuracy of various methods, such as recommending jobs or finding suitable candidates for open positions.
更多查看译文
关键词
Frequent Itemset Mining,Collaborative Filtering,Ranking,Matrix Factorization,Recruitment Recommendation
AI 理解论文
溯源树
样例
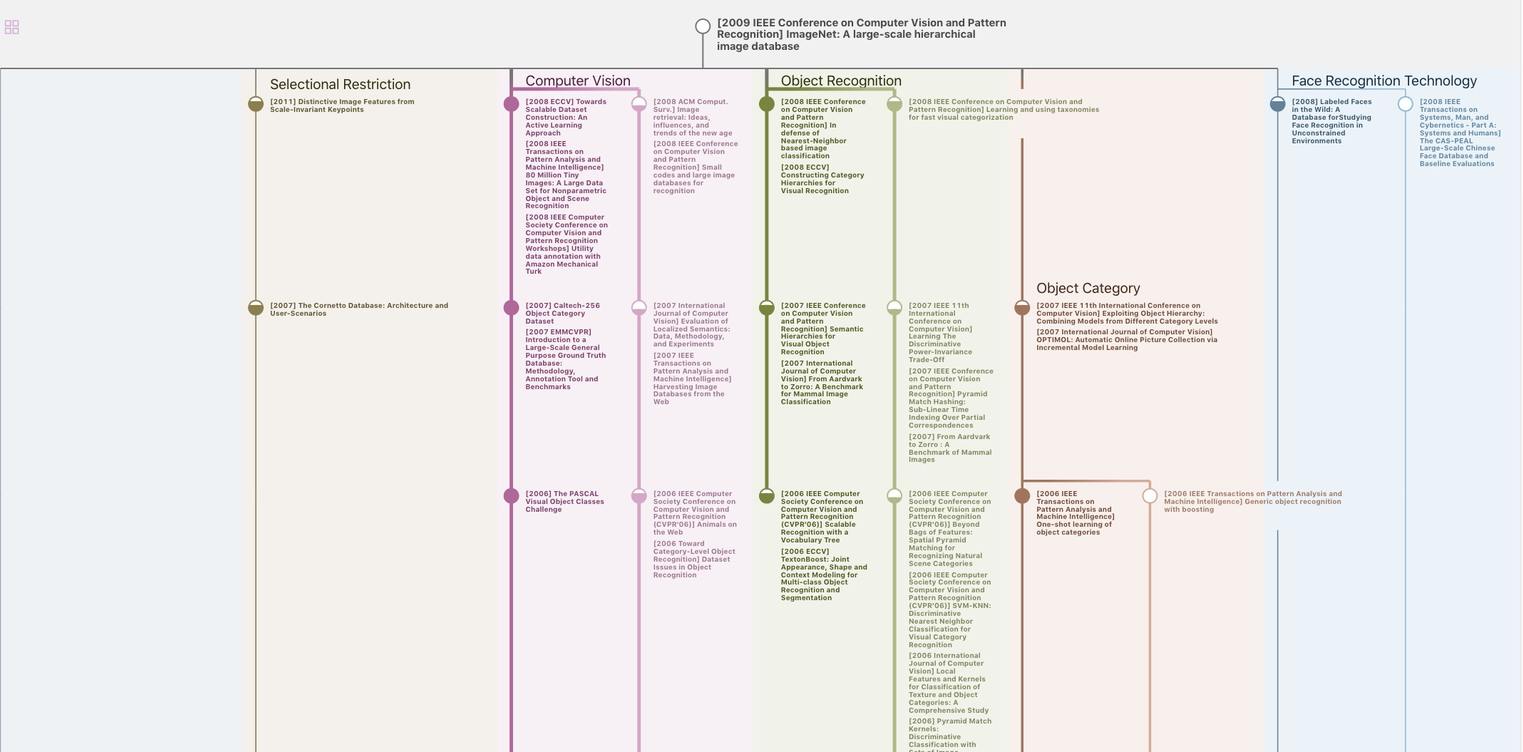
生成溯源树,研究论文发展脉络
Chat Paper
正在生成论文摘要