SAFE: Sensitivity-Aware Features for Out-of-Distribution Object Detection
arxiv(2023)
摘要
We address the problem of out-of-distribution (OOD) detection for the task of object detection. We show that residual convolutional layers with batch normalisation produce Sensitivity-Aware FEatures (SAFE) that are consistently powerful for distinguishing in-distribution from out-of-distribution detections. By extracting SAFE vectors for every detected object, and training a multilayer perceptron on the surrogate task of distinguishing adversarially perturbed from clean in-distribution examples, we circumvent the need for realistic OOD training data, computationally expensive generative models, or retraining of the base object detector. SAFE outperforms the state-of-the-art OOD object detectors on multiple benchmarks by large margins, e.g. reducing the FPR95 by an absolute 30.6% from 48.3% to 17.7% on the OpenImages dataset.
更多查看译文
关键词
detection,features,sensitivity-aware,out-of-distribution
AI 理解论文
溯源树
样例
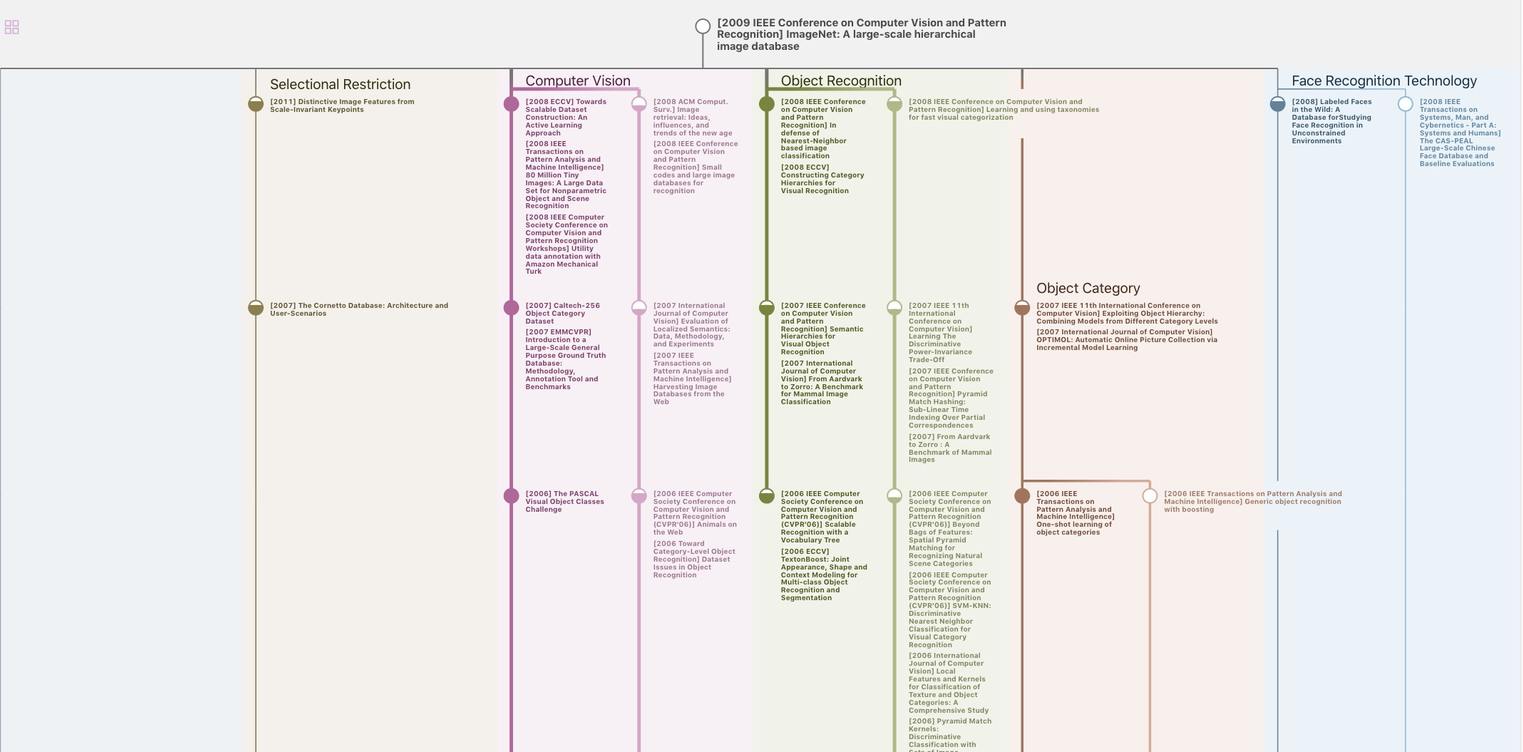
生成溯源树,研究论文发展脉络
Chat Paper
正在生成论文摘要