Architecture Growth of Dynamic Feedforward Neural Network Based on the Growth Rate Function
2022 IEEE 11th Data Driven Control and Learning Systems Conference (DDCLS)(2022)
摘要
At present, when artificial neural network is widely used to deal with practical problems, there is no theoretical choice on network scale guidance. Users often use empirical or trial-and-error methods to find an appropriate scale of the network. When the scale is too large, the neural network will need to calculate a large number of parameters when carrying out forward and back propagation, which will cause a waste of resources and time, as well as overfitting. If the scale is too small, underfitting will happen. To solve this problem, a simple, effective and easy method that to realize network growth is proposed in this paper. A growth function is designed to continuously adjust the growth of the network architecture, it takes into account multiple factors which can reflect network performance, including the current recognition rate and the changing rate of the loss of error before and after growth. Experiments show that the architecture of network can grow to a suitable scale for solving problems.
更多查看译文
关键词
dynamic feedforward neural network,neural network,growth
AI 理解论文
溯源树
样例
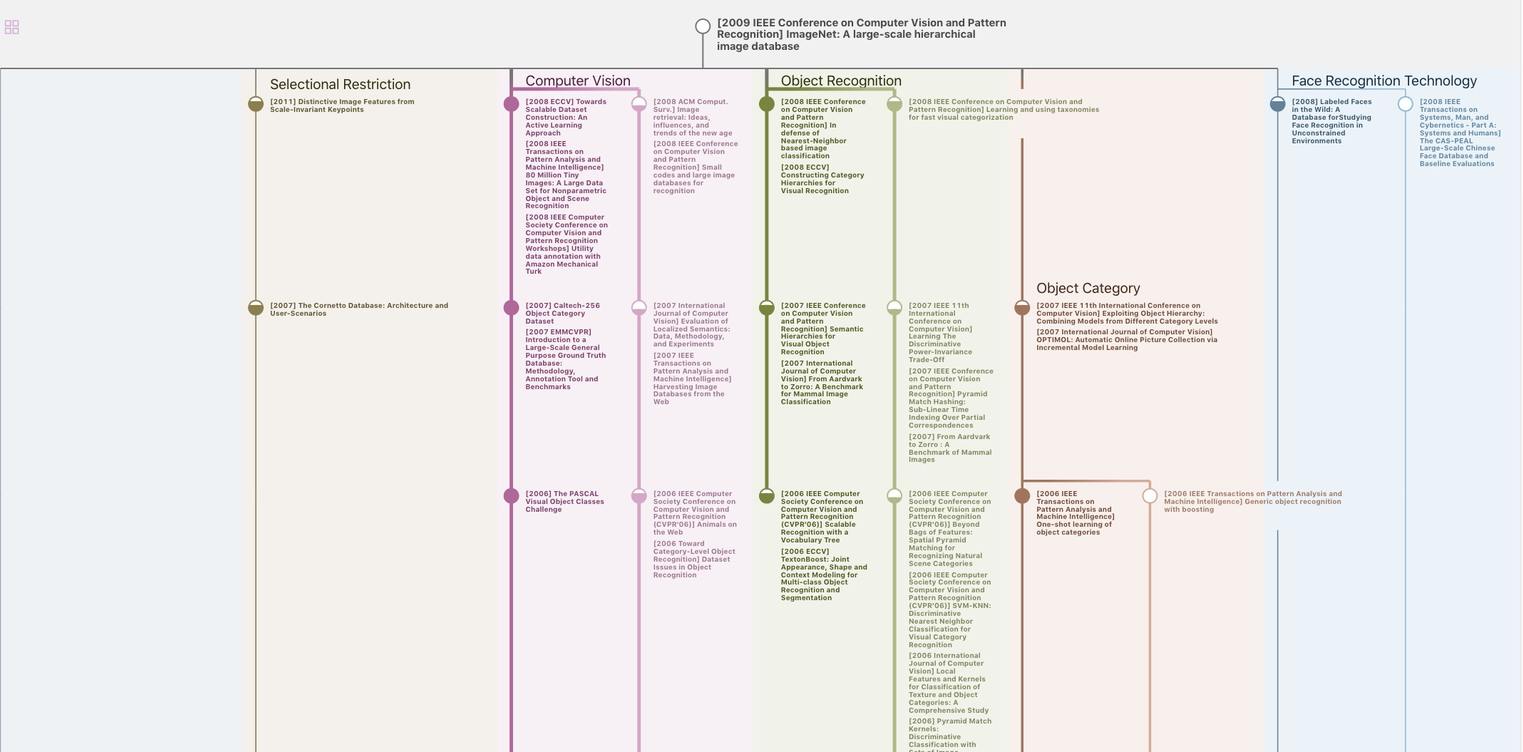
生成溯源树,研究论文发展脉络
Chat Paper
正在生成论文摘要