On differential privacy for federated learning in wireless systems with multiple base stations
IET COMMUNICATIONS(2024)
摘要
In this work, we consider a federated learning model in a wireless system with multiple base stations and inter-cell interference. We apply a differentially private scheme to transmit information from users to their corresponding base station during the learning phase. We show the convergence behavior of the learning process by deriving an upper bound on its optimality gap. Furthermore, we define an optimization problem to reduce this upper bound and the total privacy leakage. To find the locally optimal solutions of this problem, we first propose an algorithm that schedules the resource blocks and users. We then extend this scheme to reduce the total privacy leakage by optimizing the differential privacy artificial noise. We apply the solutions of these two procedures as parameters of a federated learning system where each user is equipped with a classifier and communication cells have mostly fewer resource blocks than numbers of users. The simulation results show that our proposed scheduler improves the average accuracy of the predictions compared with a random scheduler. In particular, the results show an improvement of over 6%. Furthermore, its extended version with noise optimizer significantly reduces the amount of privacy leakage. Here, we consider a federated learning model in a wireless system with multiple base stations and inter-cell interference. We apply a differentially private scheme to transmit information from users to their corresponding base station during the learning phase and analyse the performance of such a system.image
更多查看译文
关键词
6G,data privacy,federated learning,optimization,scheduling,wireless channels
AI 理解论文
溯源树
样例
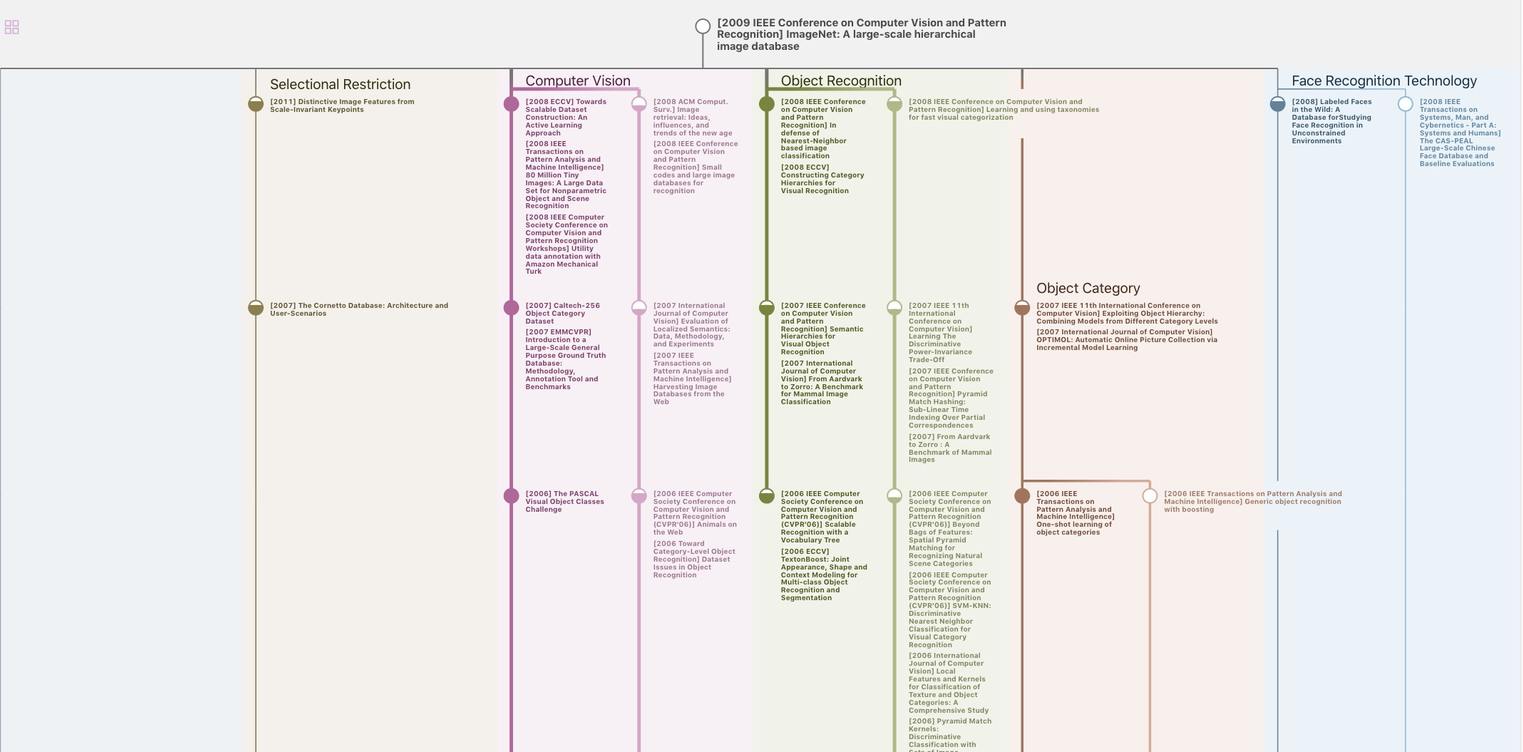
生成溯源树,研究论文发展脉络
Chat Paper
正在生成论文摘要