Improved Unet in Lithology Identification of Coal Measure Strata
Lithosphere(2022)
摘要
Abstract The lithology of underground formations can be determined using logging data, which is important for a variety of subsurface geoscience and industrial applications. Deep learning technology offers the advantage of discovering a potential relationship between input and output variables, making it a great choice for generating fast and cost-effective lithology classification models. To automatically characterize lithologies, a multiclass image segmentation problem is considered and an improved Unet as a solution is adopted. The model’s input data is two-dimensional images composed of rock feature data at different depths, and the outcome is a result of one-dimensional rock lithology classification. The algorithm’s practicality was tested using the logging data set from the Xinjing mining area in Shanxi Province, in north-central China, and an open-source data set of Canadian strata. Our model is tested against the 1D-convolutional neural network (CNN) and XGBoost algorithms using a good logging data set of the same depth and different depths for testing. The results show that the improved Unet method outperforms the 1D-CNN and XGBoost algorithms in the classification of rock lithologies. This algorithm has high application potential in the automatic interpretation of rock lithologies.
更多查看译文
关键词
lithology identification,coal
AI 理解论文
溯源树
样例
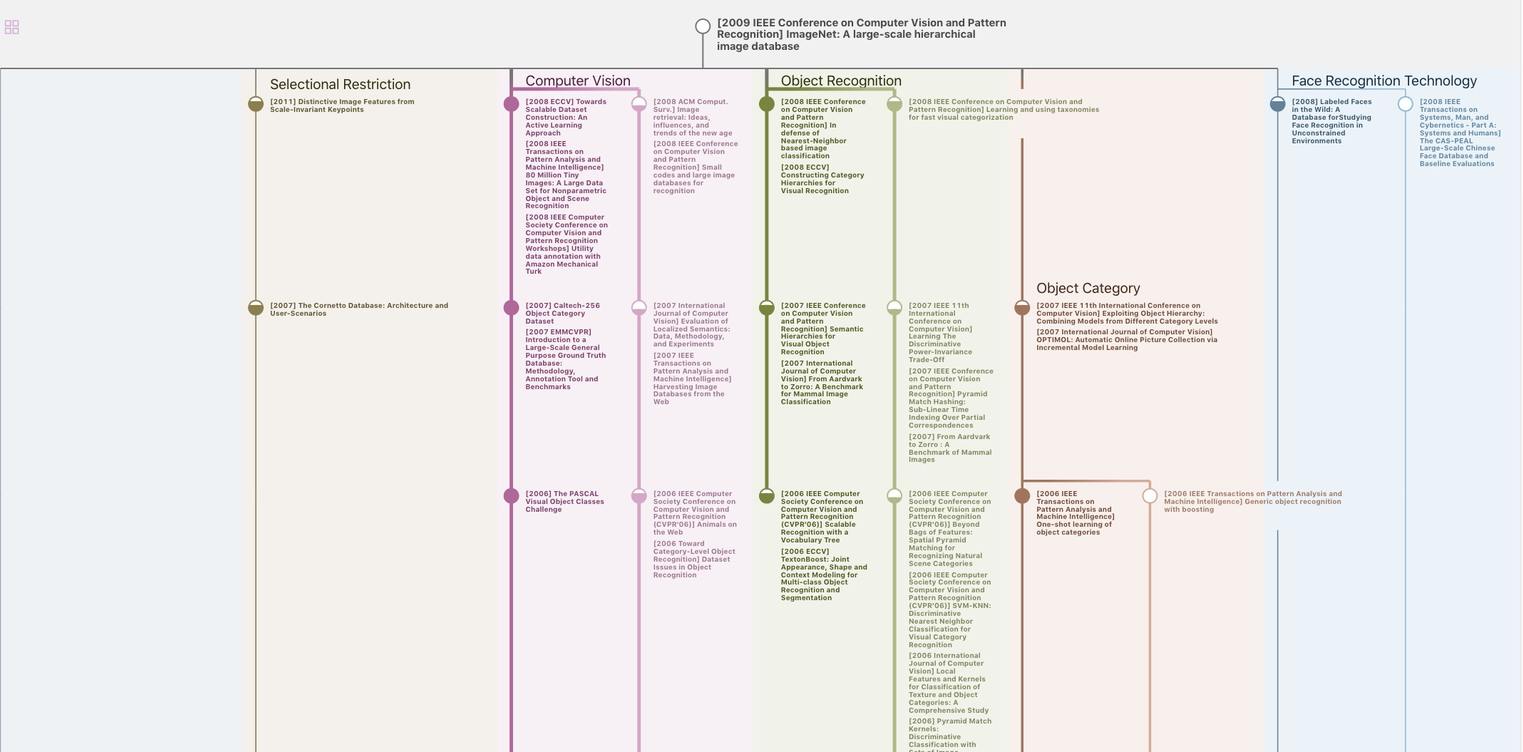
生成溯源树,研究论文发展脉络
Chat Paper
正在生成论文摘要