Learnable Human Mesh Triangulation for 3D Human Pose and Shape Estimation
WACV(2023)
摘要
Compared to joint position, the accuracy of joint rotation and shape estimation has received relatively little attention in the skinned multi-person linear model (SMPL)based human mesh reconstruction from multi-view images. The work in this field is broadly classified into two categories. The first approach performs joint estimation and then produces SMPL parameters by fitting SMPL to resultant joints. The second approach regresses SMPL parameters directly from the input images through a convolutional neural network (CNN)-based model. However, these approaches suffer from the lack of information for resolving the ambiguity of joint rotation and shape reconstruction and the difficulty of network learning. To solve the aforementioned problems, we propose a two-stage method. The proposed method first estimates the coordinates of mesh vertices through a CNN-based model from input images, and acquires SMPL parameters by fitting the SMPL model to the estimated vertices. Estimated mesh vertices provide sufficient information for determining joint rotation and shape, and are easier to learn than SMPL parameters. According to experiments using Human3.6M and MPI-INF-3DHP datasets, the proposed method significantly outperforms the previous works in terms of joint rotation and shape estimation, and achieves competitive performance in terms of joint location estimation.
更多查看译文
关键词
learnable human mesh triangulation,3d human pose,shape estimation
AI 理解论文
溯源树
样例
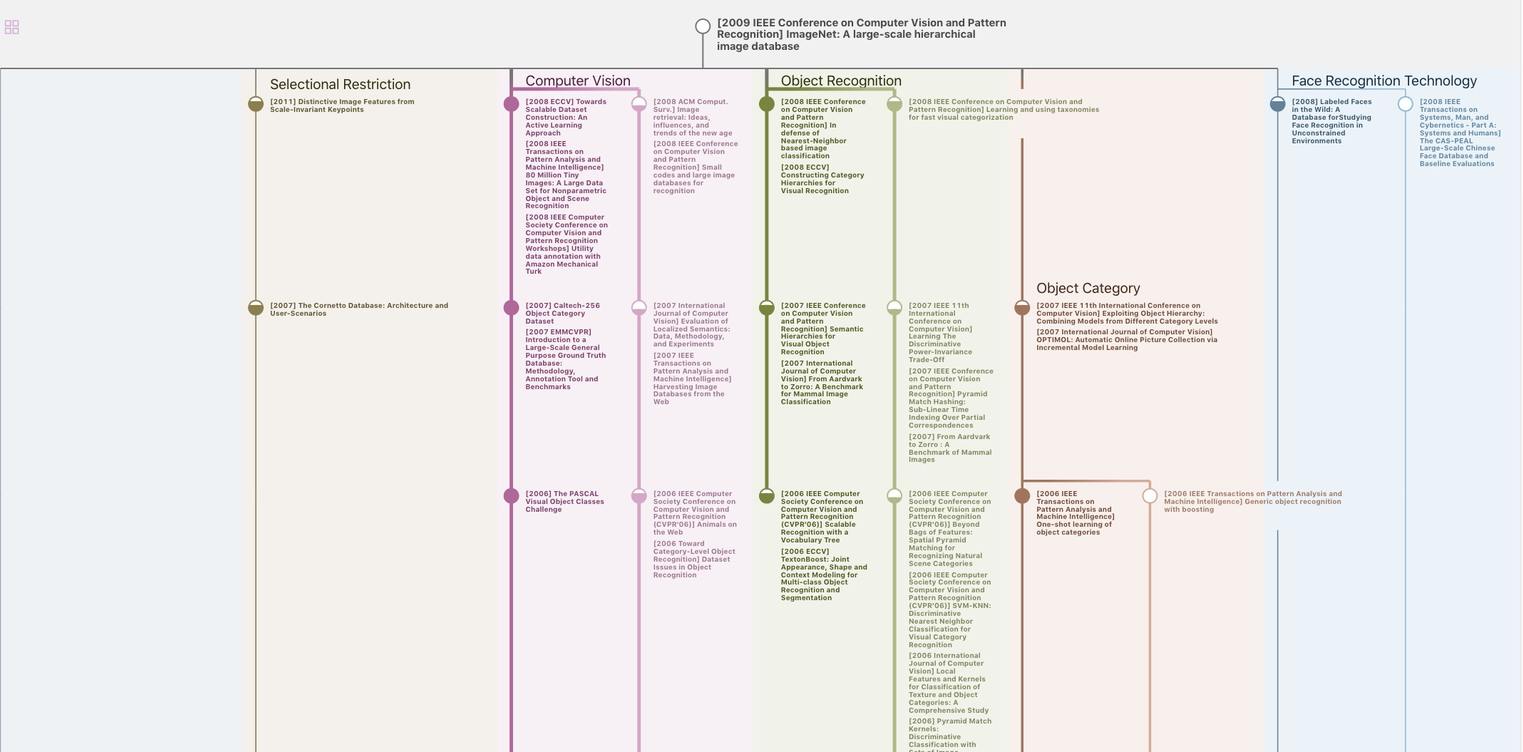
生成溯源树,研究论文发展脉络
Chat Paper
正在生成论文摘要