Prospective Explanations: An Interactive Mechanism for Model Understanding.
Annual Conference on Neural Information Processing Systems(2021)
摘要
We demonstrate a system for prospective explanations of black box models for regression and classification tasks with structured data. Prospective explanations are aimed at showing how models function by highlighting likely changes in model outcomes under changes in input. This in contrast to most post-hoc explanability methods, that aim to provide a justification for a decision retrospectively. To do so, we employ a surrogate Bayesian network model and learn dependencies through a structure learning task. Our system is designed to provide fast estimates of changes in outcomes for any arbitrary exploratory query from users. Such queries are typical partial, i.e. involve only a selected number of features, the outcomes labels are shown therefore as likelihoods. Repeated queries can indicate which aspects of the feature space are more likely to influence the target variable. We demonstrate the system from a real-world application from the humanitarian sector and show the value of bayesian network surrogates.
更多查看译文
AI 理解论文
溯源树
样例
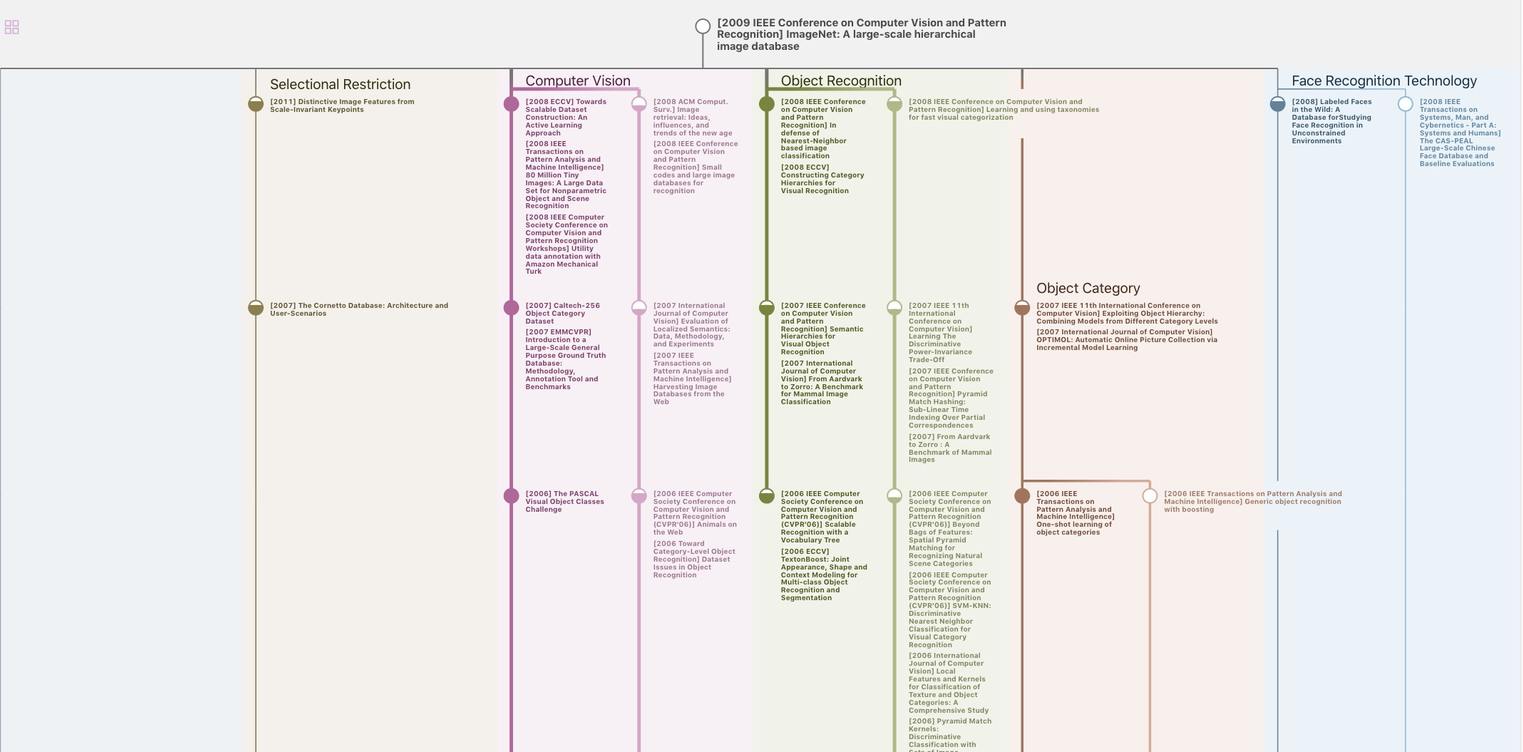
生成溯源树,研究论文发展脉络
Chat Paper
正在生成论文摘要