Semi-Autonomous Teleoperation via Learning Non-Prehensile Manipulation Skills
IEEE International Conference on Robotics and Automation(2022)
摘要
In this paper, we present a semi-autonomous teleoperation framework for a pick-and-place task using an RGB-D sensor. In particular, we assume that the target object is located in a cluttered environment where both prehensile grasping and non-prehensile manipulation are combined for efficient teleoperation. A trajectory-based reinforcement learning is utilized for learning the non-prehensile manipulation to rearrange the objects for enabling direct grasping. From the depth image of the cluttered environment and the location of the goal object, the learned policy can provide multiple options of non-prehensile manipulation to the human operator. We carefully design a reward function for the rearranging task where the policy is trained in a simulational environment. Then, the trained policy is transferred to a real-world and evaluated in a number of real-world experiments with the varying number of objects where we show that the proposed method outperforms manual keyboard control in terms of the time duration for the grasping.
更多查看译文
关键词
nonprehensile manipulation skills,semiautonomous teleoperation framework,-place task,target object,cluttered environment,prehensile grasping,efficient teleoperation,trajectory-based reinforcement learning,learned policy
AI 理解论文
溯源树
样例
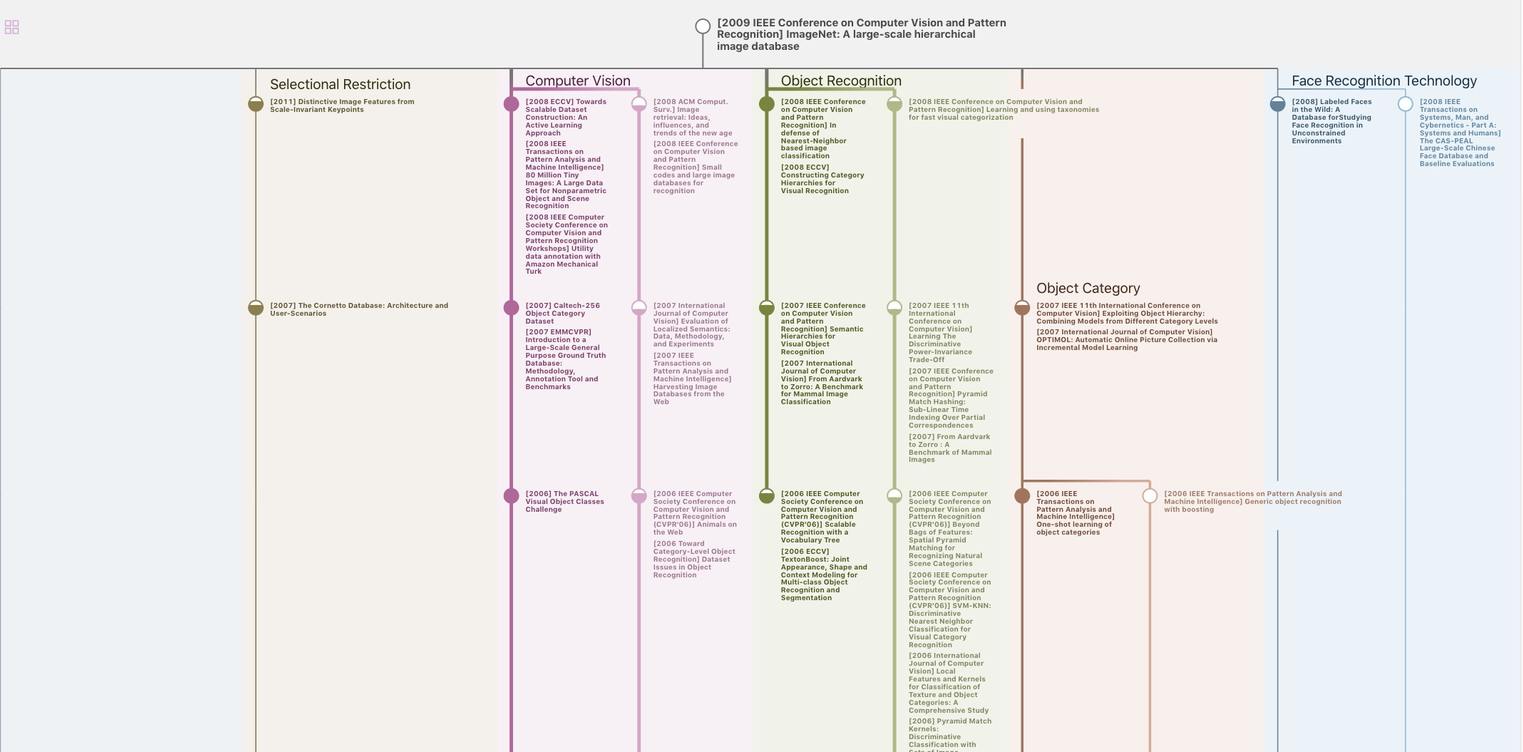
生成溯源树,研究论文发展脉络
Chat Paper
正在生成论文摘要