On the Opportunity of Causal Learning in Recommendation Systems: Foundation, Estimation, Prediction and Challenges
European Conference on Artificial Intelligence (ECAI)(2022)
摘要
Recently, recommender system (RS) based on causal inference has gained much attention in the industrial community, as well as the states of the art performance in many prediction and debiasing tasks. Nevertheless, a unified causal analysis framework has not been established yet. Many causal-based prediction and debiasing studies rarely discuss the causal interpretation of various biases and the rationality of the corresponding causal assumptions. In this paper, we first provide a formal causal analysis framework to survey and unify the existing causal-inspired recommendation methods, which can accommodate different scenarios in RS. Then we propose a new taxonomy and give formal causal definitions of various biases in RS from the perspective of violating the assumptions adopted in causal analysis. Finally, we formalize many debiasing and prediction tasks in RS, and summarize the statistical and machine learning-based causal estimation methods, expecting to provide new research opportunities and perspectives to the causal RS community.
更多查看译文
关键词
causal learning,recommendation systems
AI 理解论文
溯源树
样例
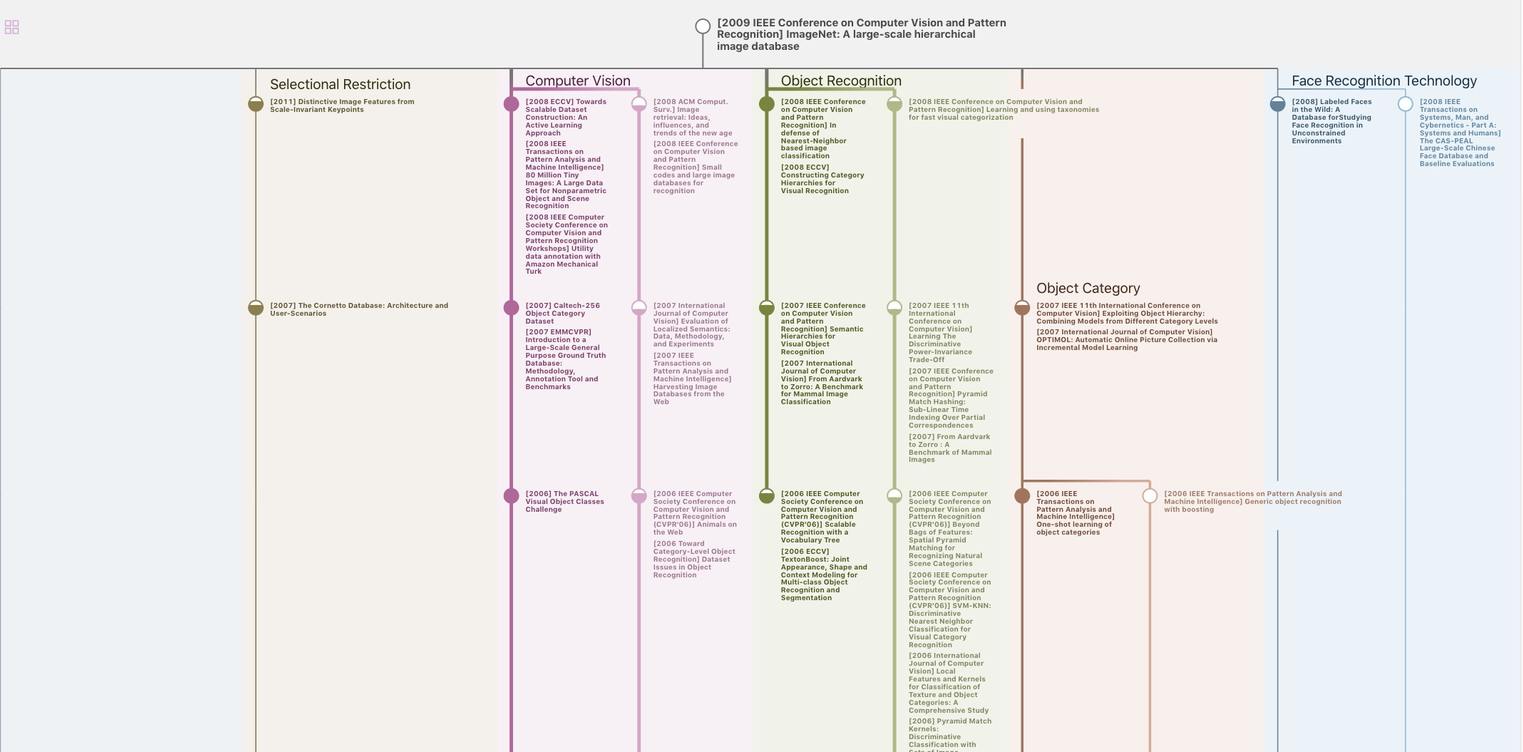
生成溯源树,研究论文发展脉络
Chat Paper
正在生成论文摘要