Multiple Consistency Supervision based Semi-supervised OCT Segmentation using Very Limited Annotations.
IEEE International Conference on Robotics and Automation(2022)
摘要
Optical Coherence Tomography (OCT) is a rapidly growing and promising imaging technique, enabling non-invasive high-resolution visualization of biological tissues. Segmentation of tissue structures from OCT scans is essen-tial for disease diagnosis but remains challenging for the blurry boundaries and large volumes. Deep learning-based OCT segmentation algorithms always require large numbers of annotations for satisfying performance, which is hard to meet since manually labeling is time-consuming and labor-intensive. Therefore, we propose a novel semi-supervised OCT segmentation framework utilizing very few labeled scans, i.e., 5 samples, and abundant unlabeled data. Specifically, our framework con-sists of one shared encoder and two different decoder branches. For the two branches, we design a strong augmentation-consistent supervision module and a scaling transformation-consistent supervision module respectively to improve their generalization ability. Besides, cross consistency supervision with feature perturbations between two branches is proposed to incorporate their advantages for further regularization. With such multiple consistency supervision, we aim to enrich the diversity of unsupervised information so as to make full use of labeled and unlabeled data. Experimental results on a public retinal OCT dataset demonstrate the effectiveness of our method, achieving an average dice score of 87.25% in the case of only 5 labeled samples used. It outperforms the supervised baseline by 3.46% and the best semi-supervised model by 1.42% in our experiments.
更多查看译文
关键词
multiple consistency supervision,segmentation,semi-supervised
AI 理解论文
溯源树
样例
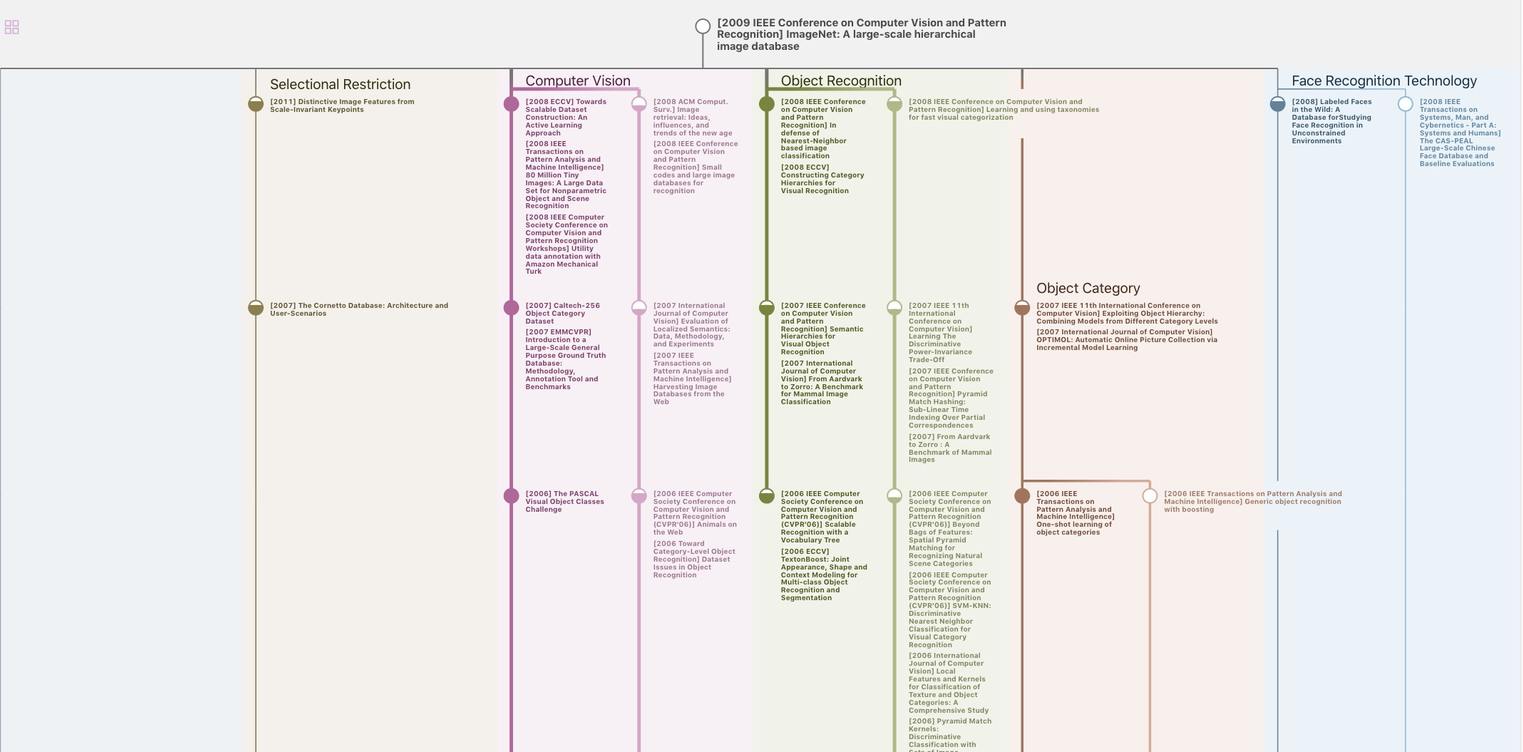
生成溯源树,研究论文发展脉络
Chat Paper
正在生成论文摘要