Spatial-Spectral Constrained Adaptive Graph for Hyperspectral Image Clustering.
SENSORS(2022)
摘要
Hyperspectral image (HSI) clustering is a challenging task, whose purpose is to assign each pixel to a corresponding cluster. The high-dimensionality and noise corruption are two main problems that limit the performance of HSI clustering. To address those problems, this paper proposes a projected clustering with a spatial-spectral constrained adaptive graph (PCSSCAG) method for HSI clustering. PCSSCAG first constructs an adaptive adjacency graph to capture the accurate local geometric structure of HSI data adaptively. Then, a spatial-spectral constraint is employed to simultaneously explore the spatial and spectral information for reducing the negative influence on graph construction caused by noise. Finally, projection learning is integrated into the spatial-spectral constrained adaptive graph construction for reducing the redundancy and alleviating the computational cost. In addition, an alternating iteration algorithm is designed to solve the proposed model, and its computational complexity is theoretically analyzed. Experiments on two different scales of HSI datasets are conducted to evaluate the performance of PCSSCAG. The associated experimental results demonstrate the superiority of the proposed method for HSI clustering.
更多查看译文
关键词
clustering, adaptive graph, spatial-spectral constraint, hyperspectral image
AI 理解论文
溯源树
样例
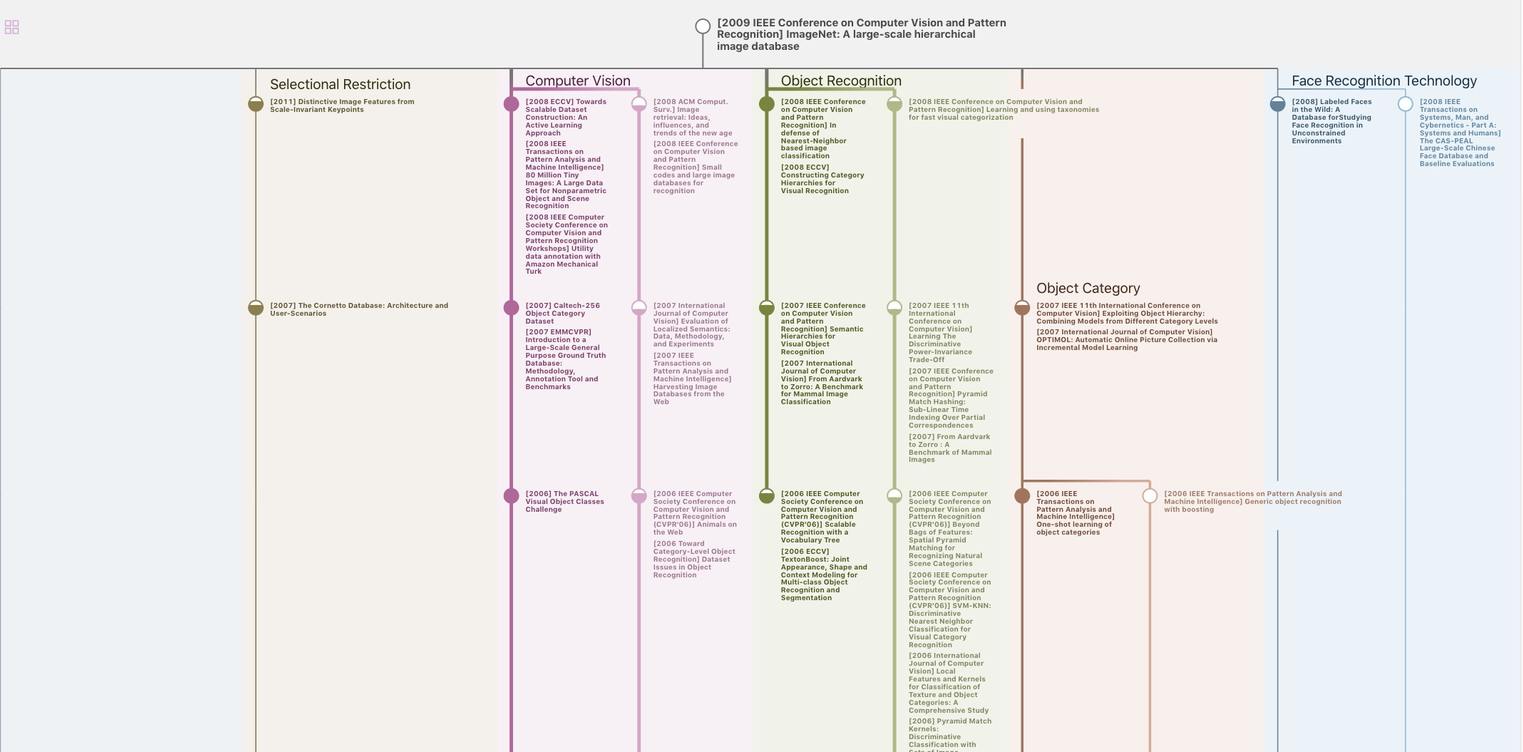
生成溯源树,研究论文发展脉络
Chat Paper
正在生成论文摘要