Invited: Improving GNN-Based Accelerator Design Automation with Meta Learning
Design Automation Conference (DAC)(2022)
Abstract
Recently, there is a growing interest in developing learning-based models as a surrogate of the High-Level Synthesis (HLS) tools, where the key objective is rapid prediction of the quality of a candidate HLS design for automated design space exploration (DSE). Training is usually conducted on a given set of computation kernels (or kernels in short) needed for hardware acceleration. However, the model must also perform well on new kernels. The discrepancy between the training set and new kernels, called domain shift, frequently leads to model accuracy drop which in turn negatively impact the DSE performance. In this paper, we investigate the possibility of adapting an existing meta-learning approach, named MAML, to the task of design quality prediction. Experiments show the MAML-enhanced model outperforms a simple baseline based on fine tuning in terms of both offline evaluation on
MoreTranslated text
Key words
accelerator design automation,meta learning,gnn-based
AI Read Science
Must-Reading Tree
Example
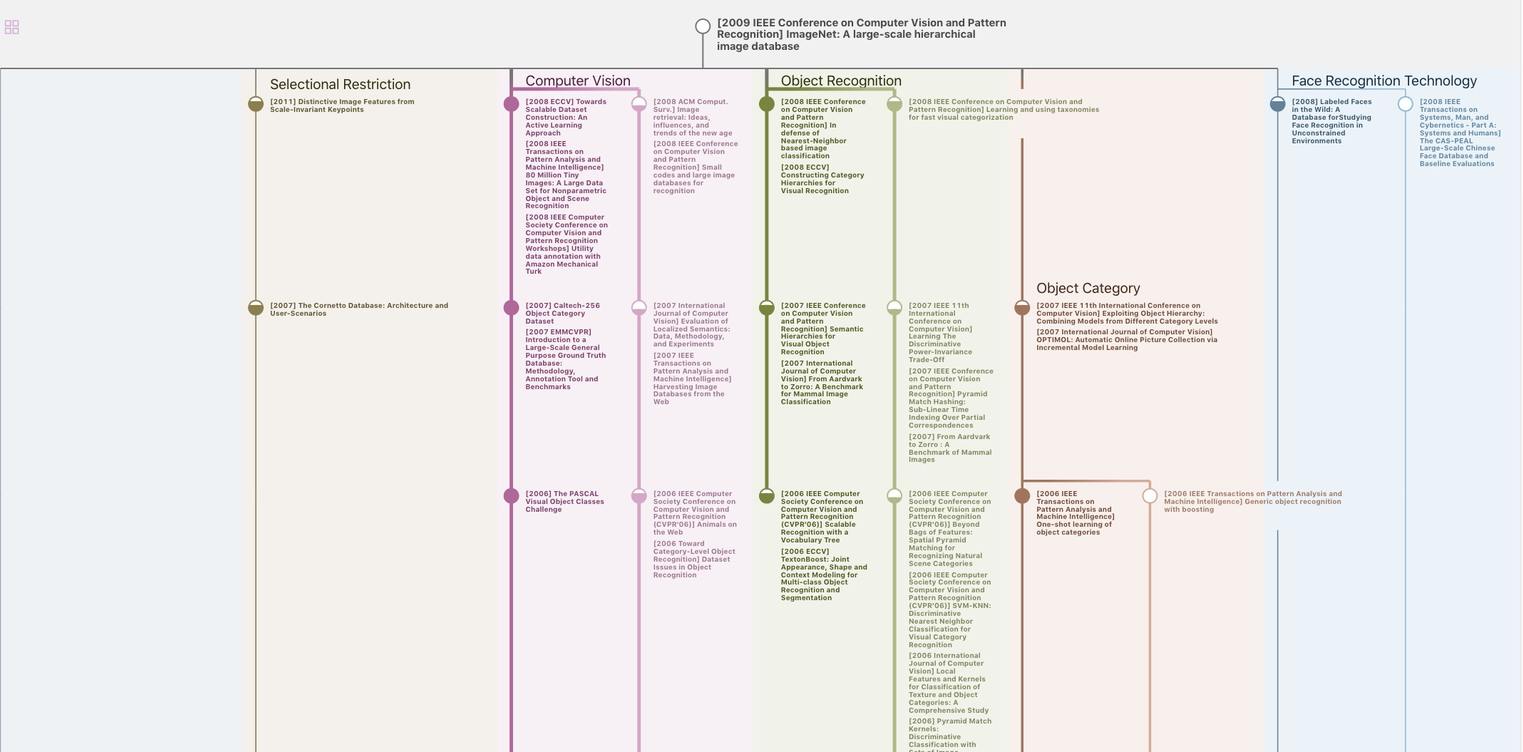
Generate MRT to find the research sequence of this paper
Chat Paper
Summary is being generated by the instructions you defined