Distributional Correlation-Aware Knowledge Distillation for Stock Trading Volume Prediction
arxiv(2023)
摘要
Traditional knowledge distillation in classification problems transfers the knowledge via class correlations in the soft label produced by teacher models, which are not available in regression problems like stock trading volume prediction. To remedy this, we present a novel distillation framework for training a light-weight student model to perform trading volume prediction given historical transaction data. Specifically, we turn the regression model into a probabilistic forecasting model, by training models to predict a Gaussian distribution to which the trading volume belongs. The student model can thus learn from the teacher at a more informative distributional level, by matching its predicted distributions to that of the teacher. Two correlational distillation objectives are further introduced to encourage the student to produce consistent pairwise relationships with the teacher model. We evaluate the framework on a real-world stock volume dataset with two different time window settings. Experiments demonstrate that our framework is superior to strong baseline models, compressing the model size by 5x while maintaining 99.6% prediction accuracy. The extensive analysis further reveals that our framework is more effective than vanilla distillation methods under low-resource scenarios. Our code and data are available at https:// github.com/lancopku/DCKD.
更多查看译文
关键词
stock trading volume prediction,correlation–aware knowledge distillation
AI 理解论文
溯源树
样例
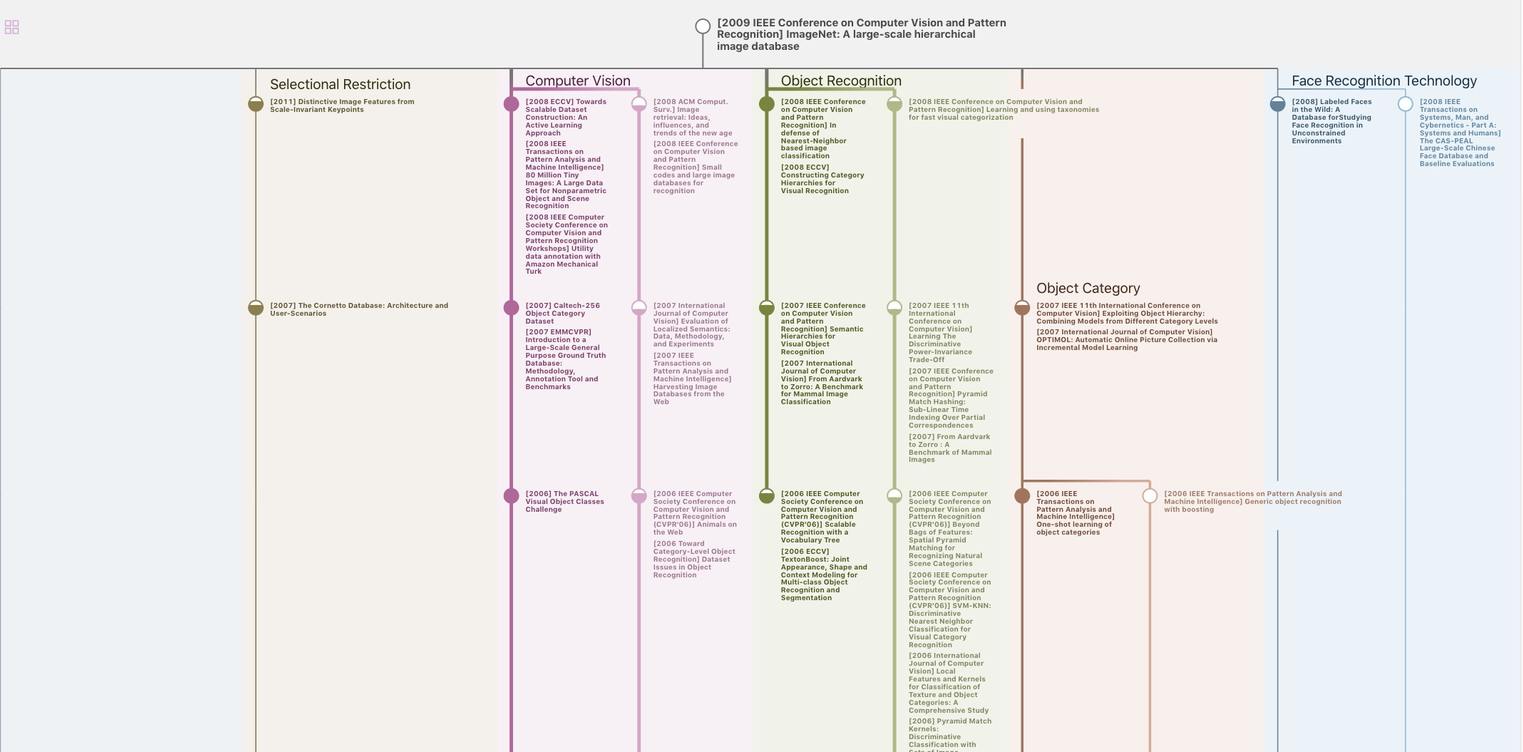
生成溯源树,研究论文发展脉络
Chat Paper
正在生成论文摘要