Understanding the stochastic dynamics of sequential decision-making processes: A path-integral analysis of Multi-armed Bandits
CoRR(2022)
摘要
The multi-armed bandit (MAB) model is one of the most classical models to study decision-making in an uncertain environment. In this model, a player needs to choose one of K possible arms of a bandit machine to play at each time step, where the corresponding arm returns a random reward to the player, potentially from a specific unknown distribution. The target of the player is to collect as much rewards as possible during the process. Despite its simplicity, the MAB model offers an excellent playground for studying the trade-off between exploration versus exploitation and designing effective algorithms for sequential decision-making under uncertainty. Although many asymptotically optimal algorithms have been established, the finite-time behaviours of the stochastic dynamics of the MAB model appears much more difficult to analyze, due to the intertwining between the decision-making and the rewards being collected. In this paper, we employ techniques in statistical physics to analyze the MAB model, which facilitates to characterize the distribution of cumulative regrets at a finite short time, the central quantity of interest in an MAB algorithm, as well as the intricate dynamical behaviours of the model.
更多查看译文
关键词
stochastic dynamics,decision-making decision-making,processes,path-integral,multi-armed
AI 理解论文
溯源树
样例
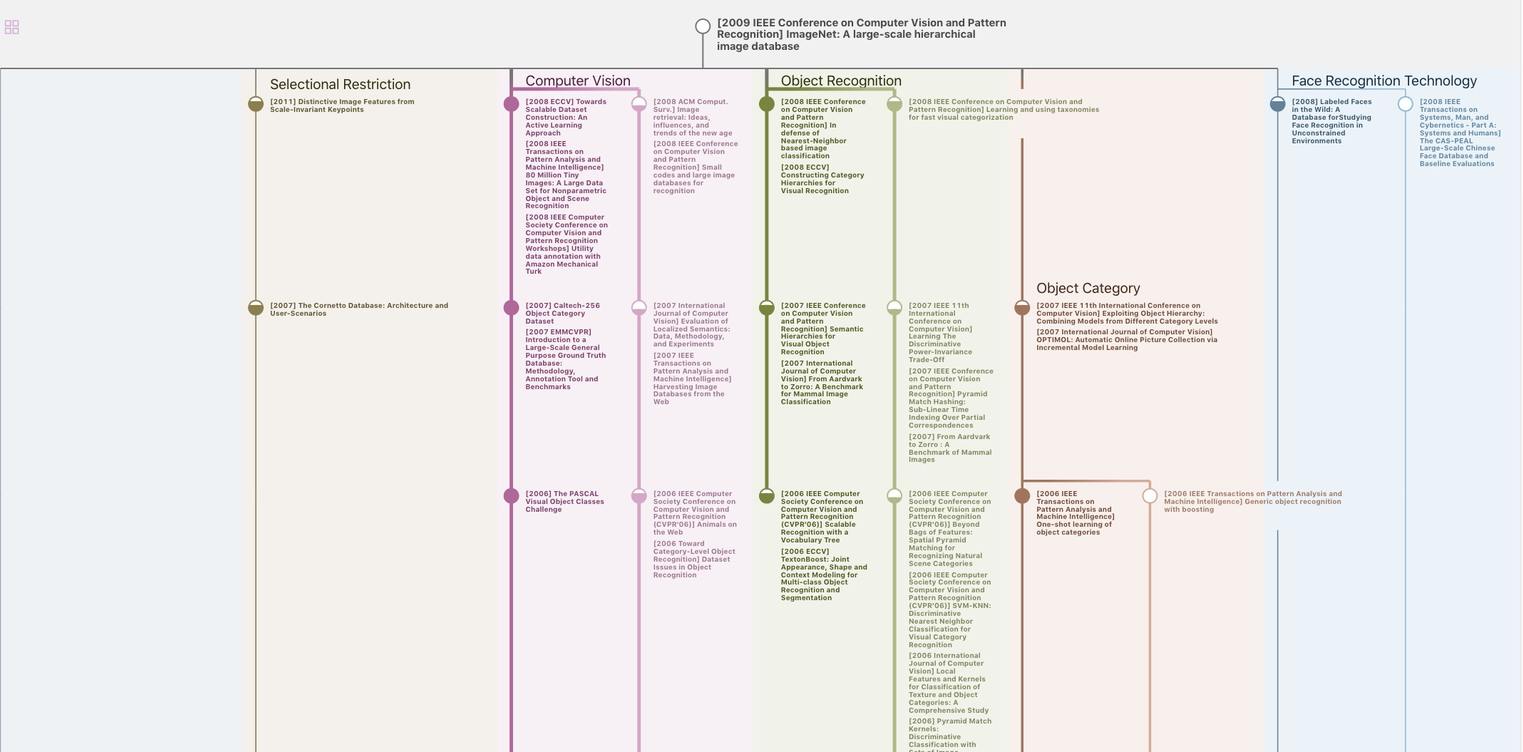
生成溯源树,研究论文发展脉络
Chat Paper
正在生成论文摘要