Adapting Distilled Knowledge for Few-shot Relation Reasoning over Knowledge Graphs
SIAM International Conference on Data Mining (SDM)(2022)
摘要
Knowledge graphs (KGs) are serving as important resources for many applications, such as semantic search, question answering, or dialogue generation. As one of the fundamental tasks, multi-hop KG reasoning aims at generating effective and explainable relation prediction through reasoning paths. The current methods often require sufficient amount of training data (i.e., fact triples) for each query relation, impairing their applicabilities and performances over few-shot relations (with limited data) which are common in KGs. Despite that some few-shot relation reasoning methods have been proposed, their effectiveness and efficiency remain to be improved. To address these challenges, we propose a novel model called ADK-KG for multi-hop few-shot relation reasoning over KGs. In ADK-KG, we introduce a reinforcement learning framework to model the sequential reasoning process. We further develop a text-enhanced heterogeneous graph neural network to encode node embeddings, where entity and relation embeddings are pre-trained using content information. Later, we employ a task-aware meta-learning algorithm to optimize the model parameters that could be fast adapted for few-shot relations. A knowledge distillation module is further designed to make use of unlabeled data for improving model training. Extensive experiments on three benchmark datasets demonstrate that ADK-KG has satisfactory efficiency and outperforms state-of-the-art approaches.MSC codesKnowledge graphsRelation reasoningFew-shot learningGraph neural network
更多查看译文
关键词
distilled knowledge,relation,reasoning,few-shot
AI 理解论文
溯源树
样例
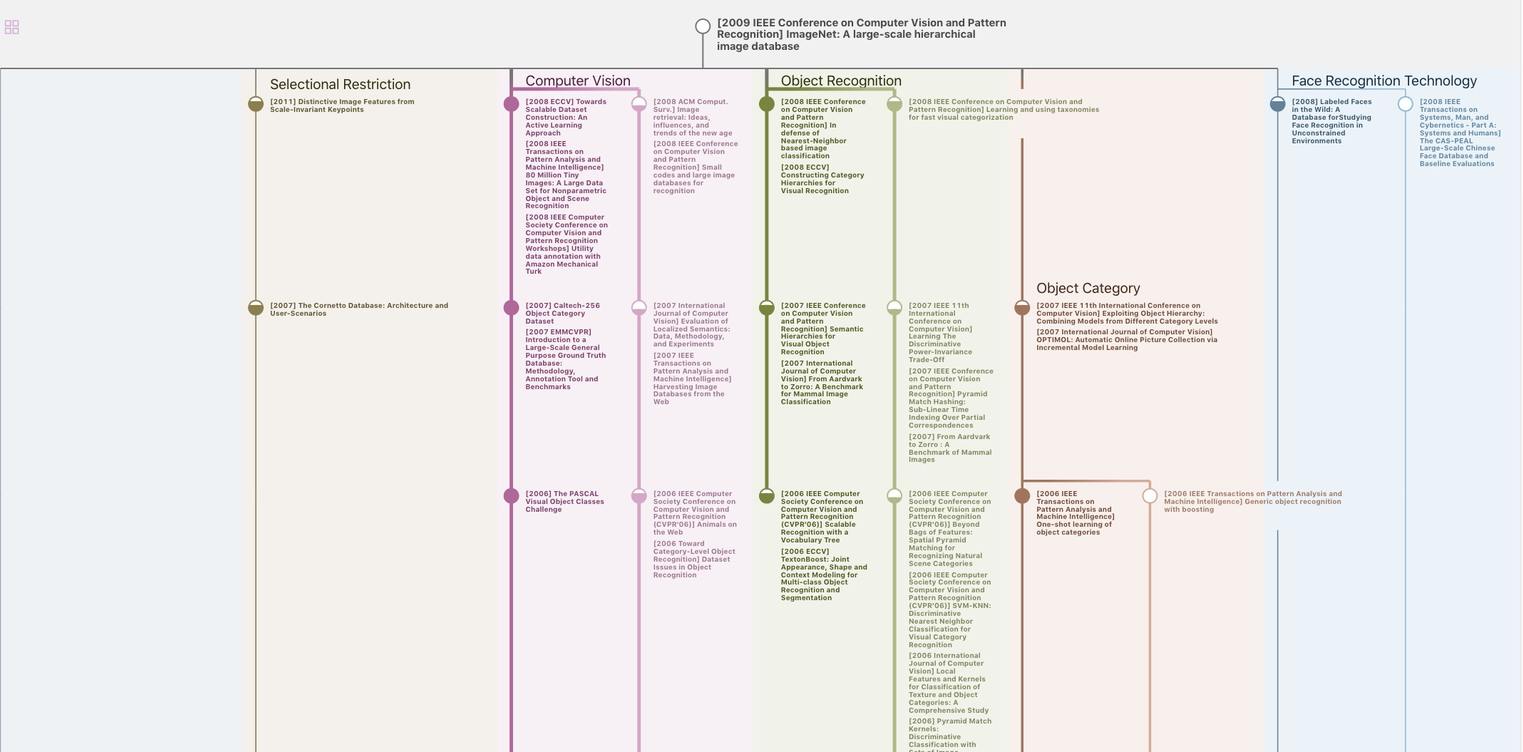
生成溯源树,研究论文发展脉络
Chat Paper
正在生成论文摘要