Solar power time series forecasting utilising wavelet coefficients
Neurocomputing(2022)
摘要
Accurate and reliable prediction of photovoltaic (PV) power output is critical to electricity grid stability and power dispatching capabilities. However, PV power generation is highly volatile and unstable due to different reasons. The wavelet transform (WT) has been utilised in time series applications, such as PV power prediction, to model the stochastic volatility and reduce prediction errors. Yet the existing WT approach has a limitation in terms of time complexity. It requires reconstructing the decomposed components and modelling them separately and thus needs more time for reconstruction, model configuration and training. The aim of this study is to improve the efficiency of applying WT by proposing a new method that uses a single simplified model. Given a time series and its WT coefficients, it trains one model with the coefficients as features and the original time series as labels. This eliminates the need for component reconstruction and training numerous models. This work contributes to the day-ahead aggregated solar PV power time series prediction problem by proposing and comprehensively evaluating a new approach of employing WT. The proposed approach is evaluated using 17 months of aggregated solar PV power data from two real-world datasets. The evaluation includes the use of a variety of prediction models, including Linear Regression, Random Forest, Support Vector Regression, and Convolutional Neural Networks. The results indicate that using a coefficients-based strategy can give predictions that are comparable to those obtained using the components-based approach while requiring fewer models and less computational time.
更多查看译文
关键词
Time series,Photovoltaic power,Forecasting,Wavelet transform,Machine learning
AI 理解论文
溯源树
样例
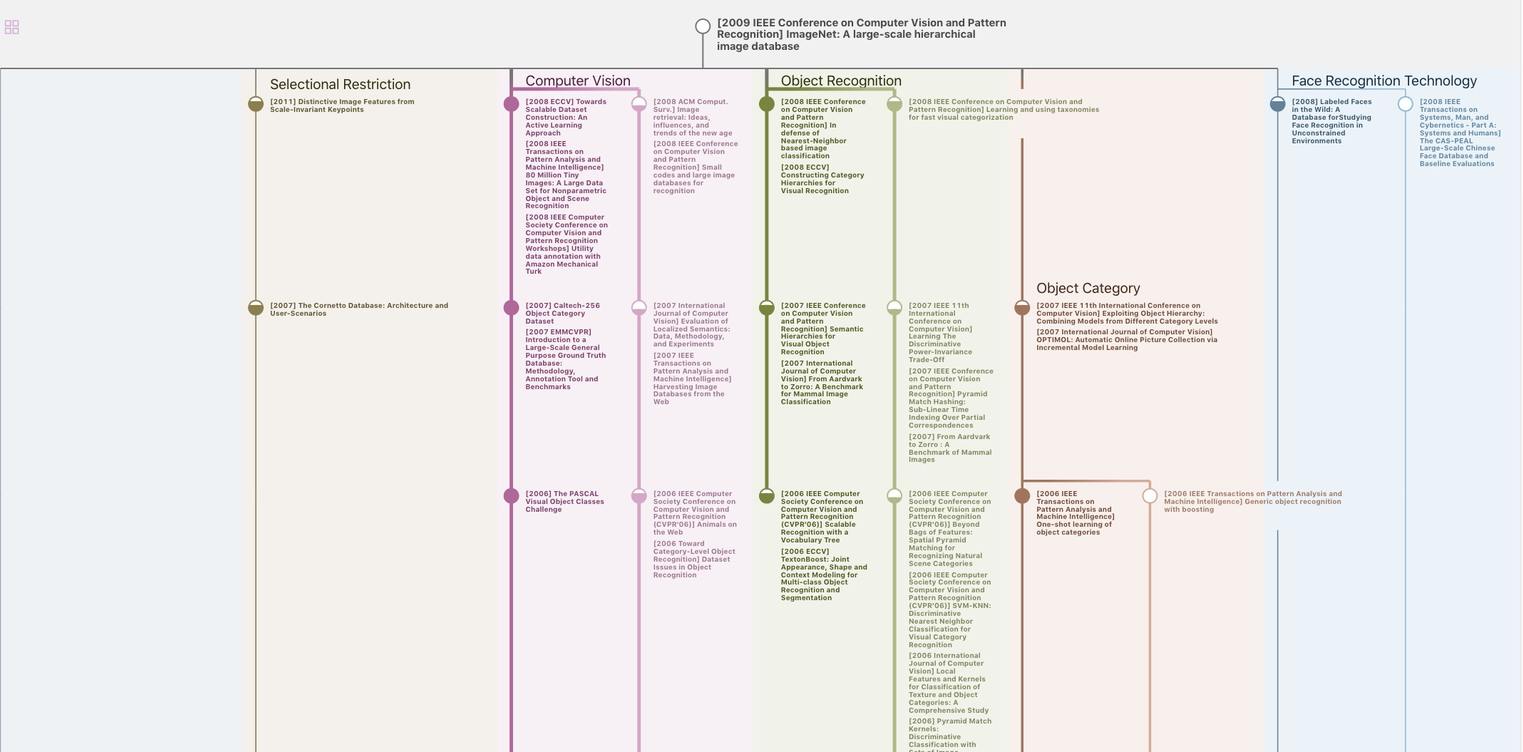
生成溯源树,研究论文发展脉络
Chat Paper
正在生成论文摘要