PHY-NET: A Channel Autoencoder Approach for Wireless PHY Layer Design
ICC 2022 - IEEE International Conference on Communications(2022)
摘要
In this work, we present a deep learning model for creating wireless communications waveforms with tailored physical layer attributes. A multi-task learning framework based on the notion of a channel autoencoder is presented and two secondary learning goals are introduced. Data bits are the input to the deep learning model and the output is a latent representation that can be interpreted as complex baseband samples. This output representation is perturbed by a wireless channel model and distributed to the decoder, modeling a classical communications demodulator. In addition, the output representation is distributed to one or many expert-designed loss functions that serve the purpose of providing feedback to the encoder such that specific physical layer design constraints can be imposed. Synthesis of a waveform is demonstrated with example energy and cyclostationary design constraints. The bit error rate of the synthesized waveform is compared to that of a conventional BPSK modulation. When trained to optimize transmit energy, the model learned to reduce transmit power until a balance between bit error rate and transmit power is found. When trained to tune cycle features, the model learned to impose an atypical pulse shape exhibiting suppressed cycle features and a 1 dB bit error rate performance loss. These models are learned without applying expert knowledge to the waveform design process; rather, the framework uses a set of constraints to guide the development.
更多查看译文
关键词
Cognitive Radio,Deep Learning,Wireless Communications,Wireless Coexistence
AI 理解论文
溯源树
样例
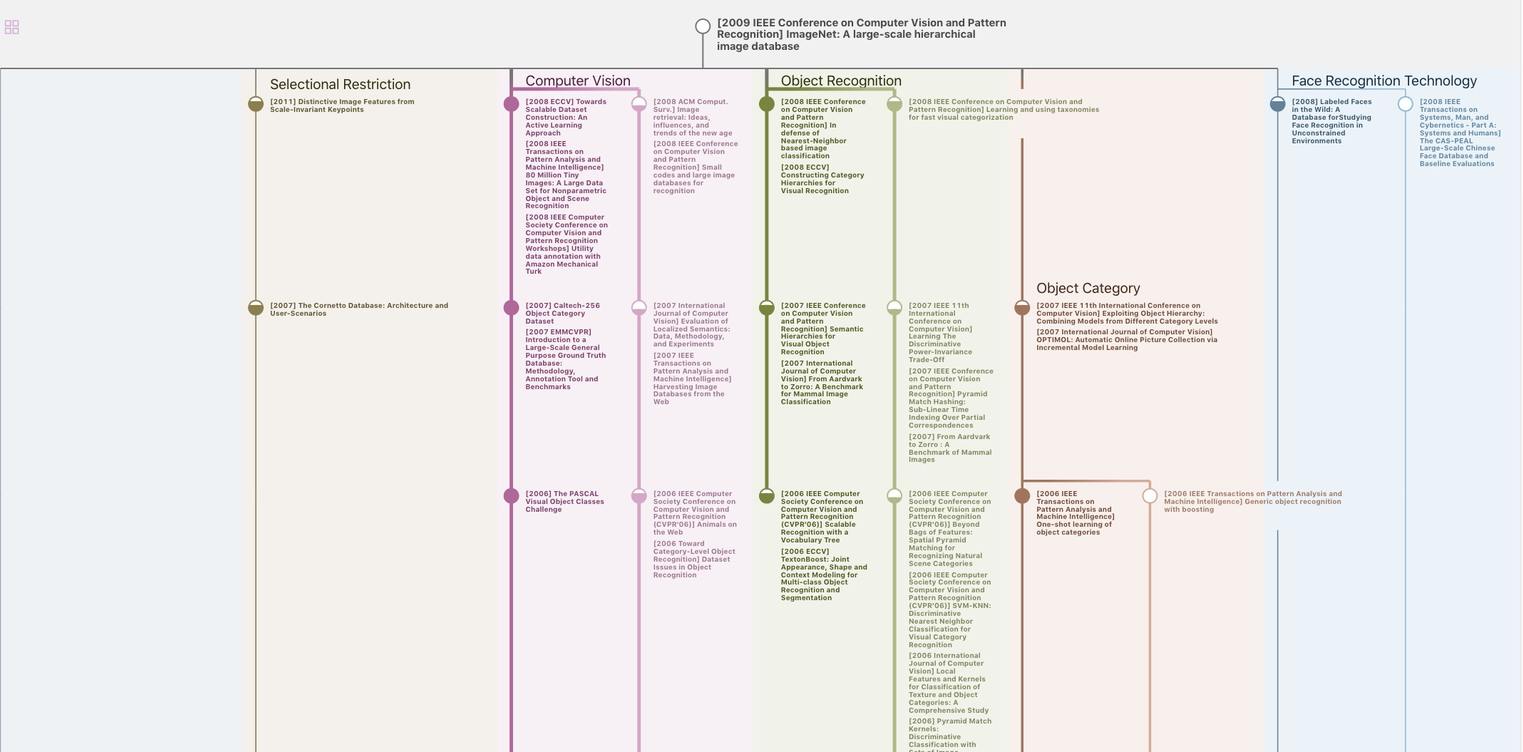
生成溯源树,研究论文发展脉络
Chat Paper
正在生成论文摘要