Data Poisoning Attacks against Autoencoder-based Anomaly Detection Models: a Robustness Analysis
ICC 2022 - IEEE International Conference on Communications(2022)
摘要
The Internet of Things (IoT) is experiencing a strong growth in both industrial and consumer scenarios. At the same time, the devices taking part in delivering IoT services-usually characterized by limited hardware and software resources-are more and more targeted by cyberattacks. This calls for designing and evaluating new approaches for protecting IoT systems, which are challenged by the limited computational capabilities of devices and by the scarce availability of reliable datasets. In line with this need, in this paper we compare three state-of-the-art machine-learning models used for Anomaly Detection based on autoencoders, i.e. shallow Autoencoder, Deep Autoencoder (DAE), and Ensemble of Autoencoders (viz. KitNET). In addition, we evaluate the robustness of such solutions when Data Poisoning Attack (DPA) occurs, to assess the detection performance when the benign traffic used for learning the legitimate behavior of devices is mixed to malicious traffic. The evaluation relies on the public Kitsune Network Attack Dataset. Results reveal that the models do not differ in performance when trained with unpoisoned benign traffic, reaching (at 1% FPR) an F1 score of approximate to 97%. However, when DPA occurs, DAE proves to be the more robust in detection, showing more than 50% of F1 Score with 10% poisoning. Instead, the other models show strong performance drops (down to approximate to 20% F1 Score) by injecting only 0.5% of the malicious traffic.
更多查看译文
关键词
anomaly detection models,robustness analysis,attacks,autoencoder-based
AI 理解论文
溯源树
样例
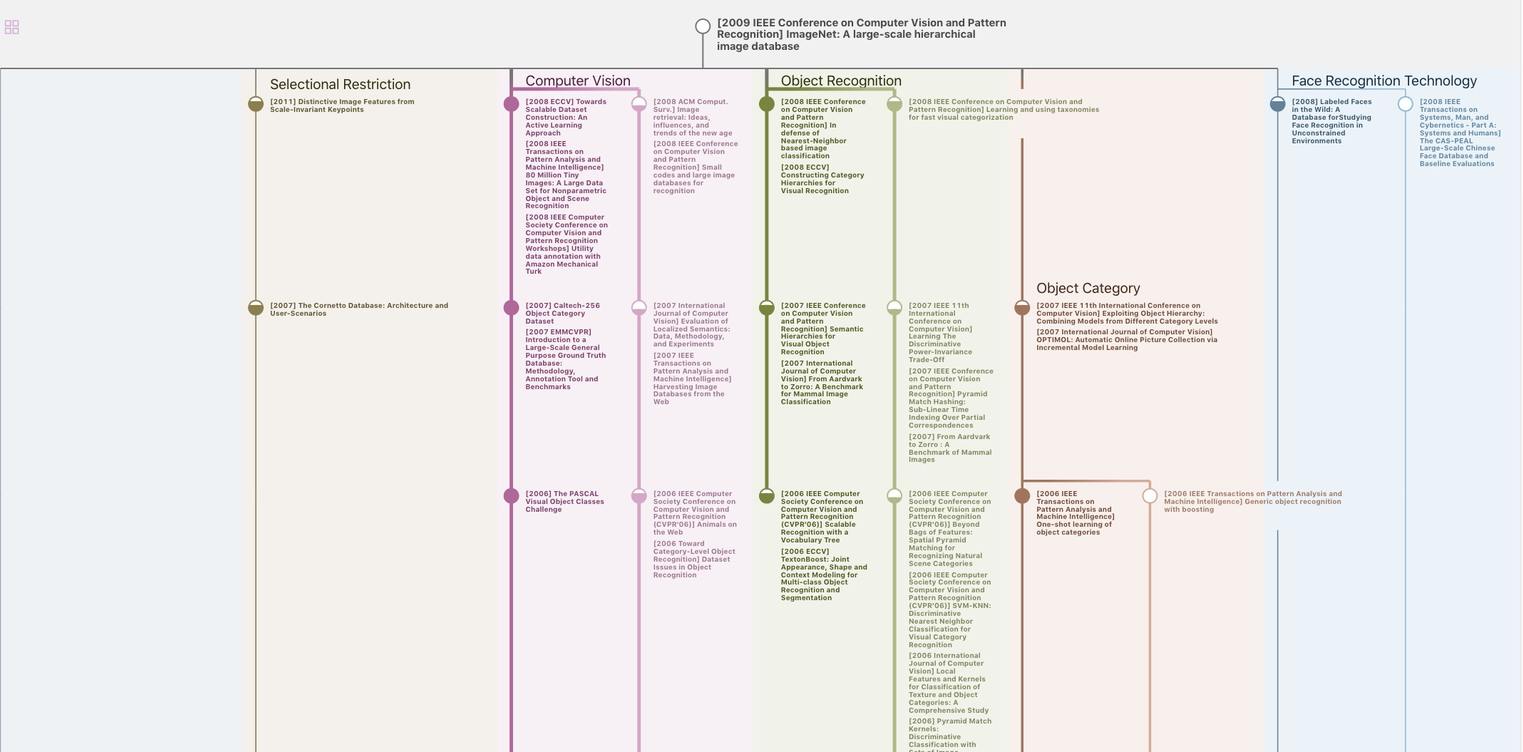
生成溯源树,研究论文发展脉络
Chat Paper
正在生成论文摘要