Mars-TRP: Classification of Mars imagery using dynamic polling betweentransferred features
ENGINEERING APPLICATIONS OF ARTIFICIAL INTELLIGENCE(2022)
摘要
Expeditions on Mars and interest in research orienting around these exploration missions have been accel-erating more than ever, recently. Due to lack of active human interference in Mars missions, processing andaccurate classification of images taken by the rovers is a very essential part of the system. Proper identificationof landforms governs the accessibility of the mobile rovers on Mars' surface. Moreover, NASA has alreadycollected over two million images from the planet, and more volumes are yet to arrive as these photographsserve as major documents for photogrammetry and studies based on remote sensing. Automatic labeling ofincoming images and also making searching of the image database easier in the public interest requireshighly accurate image classifiers. Deriving motivation from the above causes, this study intends to implementan efficient supervised multi-class image classifier for identifying Mars imagery. However, this objective isconfronted by a major bottleneck. Most datasets that are accurately labeled, portray a highly skewed natureand insufficient data to train a deep model from scratch. The MSL surface imagery labeled dataset capturedby the Curiosity rover, that has been considered for this study, is one such dataset with only 6691 imagesdistributed unevenly into 25 classes. These obstacles are less signified in the existing literature and hencethis paper addresses these challenges, outperforming the state-of-the-art metrics. Due to the absence of largedata volume, a transfer learning based methodology was considered, using very deep convolutional networkspre-trained on ImageNet dataset. But images from Mars often involve a difference in hue, contrast and claritywhen compared with images taken on Earth. Hence, the deep model was fine-tuned with our dataset and theextracted feature from the tuned neural network was used for the final classification. It was found that theresults obtained from a single pre-trained model were not optimum and that ensemble approaches could unifymany such results into a better result. Similar feature vectors were extracted from a few other pre-trainedmodels. The whole setup converges into a dynamic routing module, a novel polling algorithm, which foreach image, comes to an agreement about the best set of features while generating the output probabilityvector. The proposed approach is evaluated by several numeric metrics like accuracy, precision and recall,confusion matrices and roc curves, against the chosen individual pre-trained models and most prominentensemble methods. Mars-TRP produces a test accuracy of around 88% in the standard test set of MSL surfacedataset and an accuracy of 96% in the HiRise dataset, outperforming the individual pre-trained models, allthe ensemble baselines and other existing approaches
更多查看译文
关键词
Mars imagery,Dynamic routing,Routing by agreement,Transfer learning,Ensemble,Polling
AI 理解论文
溯源树
样例
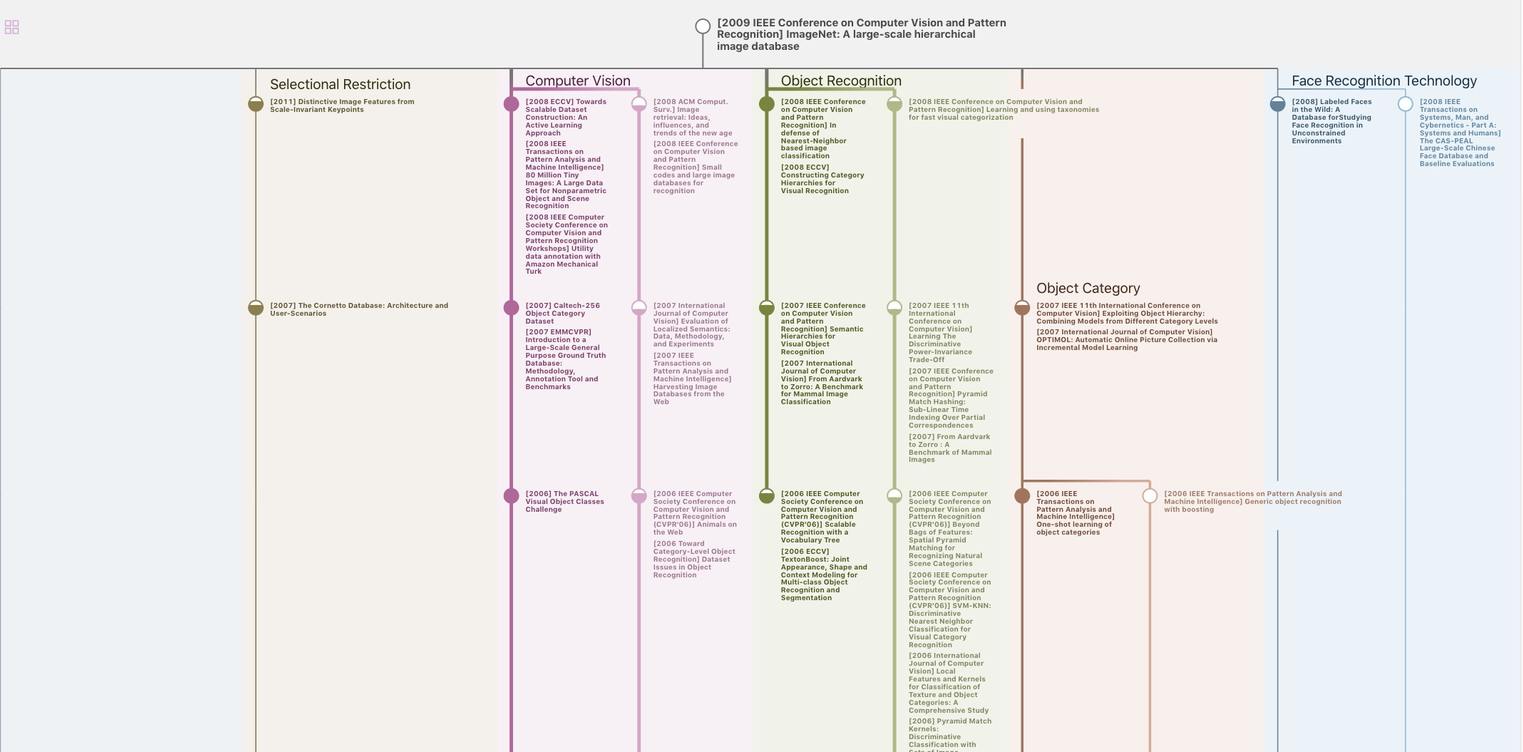
生成溯源树,研究论文发展脉络
Chat Paper
正在生成论文摘要