Design Framework for ReRAM-Based DNN Accelerators with Accuracy and Hardware Evaluation
ELECTRONICS(2022)
摘要
To achieve faster design closure, there is a need to provide a design framework for the design of ReRAM-based DNN (deep neural network) accelerator at the early design stage. In this paper, we develop a high-level ReRAM-based DNN accelerator design framework. The proposed design framework has the following three features. First, we consider ReRAM's non-linear properties, including lognormal distribution, leakage current, IR drop, and sneak path. Thus, model accuracy and circuit performance can be accurately evaluated. Second, we use SystemC with TLM modeling method to build our virtual platform. To our knowledge, the proposed design framework is the first behavior-level ReRAM deep learning accelerator simulator that can simulate real hardware behavior. Third, the proposed design framework can evaluate not only model accuracy but also hardware cost. As a result, the proposed design framework can be used for behavior-level design space exploration. In the experiments, we have deployed different DNN models on the virtual platform. Circuit performance can be easily evaluated on the proposed design framework. Furthermore, experiment results also show that the noise effects are different in different ReRAM array architectures. Based on the proposed design framework, we can easily mitigate noise effects by tuning architecture parameters.
更多查看译文
关键词
processing-in-memories, design closure, DNN accelerators, non-linear effects, simulator
AI 理解论文
溯源树
样例
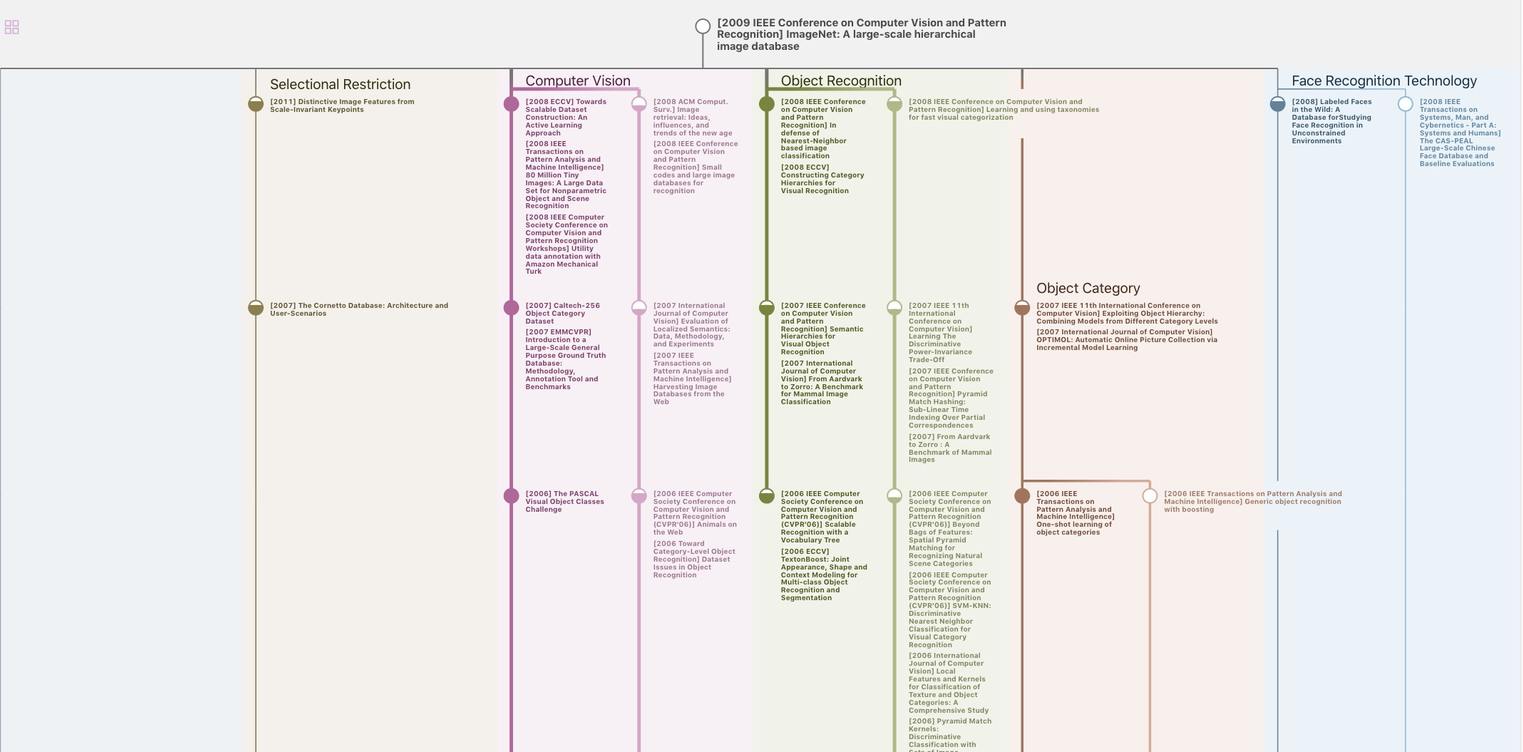
生成溯源树,研究论文发展脉络
Chat Paper
正在生成论文摘要