Deep Attention Relation Network: A Zero-Shot Learning Method for Bearing Fault Diagnosis Under Unknown Domains
IEEE Transactions on Reliability(2023)
摘要
Deep learning (DL) method are extensively used for bearing fault diagnosis (BFD). Due to severe data distribution difference under variable working conditions, they have unsatisfactory performance of the BFD. Although the existing transfer learning (TL) methods might improve the diagnostic performance in different data distributions, fault data from these different domains in training have to be obtained. When a given bearing operates in a new working condition and fault data are not available, the TL methods might be invalid, and the BFD would be postponed. To solve the above problem, a novel zero-shot learning method named deep attention relation network (DARN) is proposed for the BFD under multiple unknown domains. The built DARN only trained by the data from a known domain might be used to diagnose fault types from unknown, but related domains without prior data input. In this method, a feature extraction module is constructed to generate representations of input samples, and a relation module is designed to calculate the relation score between the sample pairs to determine their categories. Meanwhile, a parallel attention mechanism is introduced into the DARN so as to enhance the representative ability of the built model. The results of experimental study indicate that the proposed method can make use of fault knowledge learnt from the single known domain for the BFD in the several unknown domains. The proposed DARN significantly outperforms the existing popular TL methods in diagnostic performance.
更多查看译文
关键词
Bearing fault diagnosis (BFD),parallel attention mechanism (PAM),relation network,transfer learning (TL),zero-shot learning
AI 理解论文
溯源树
样例
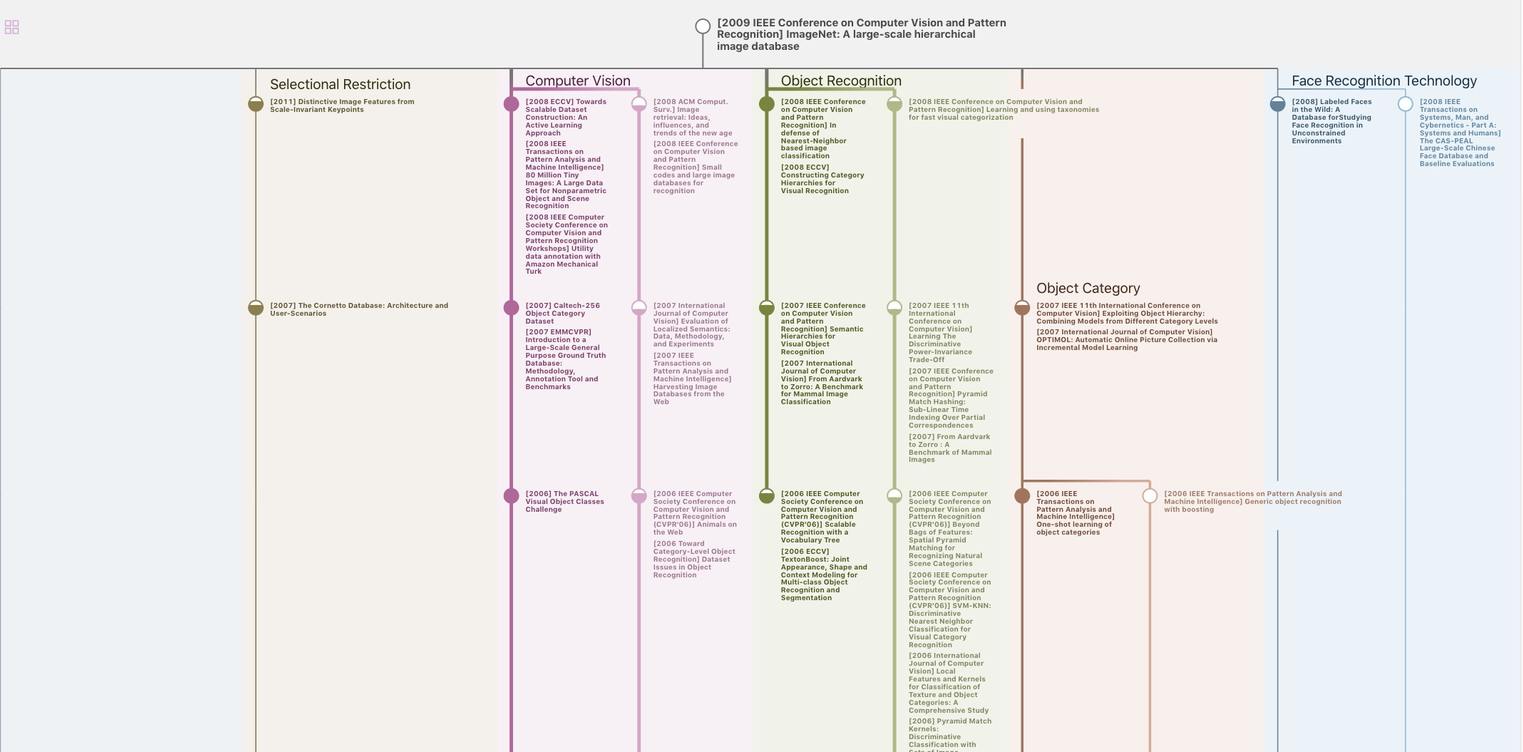
生成溯源树,研究论文发展脉络
Chat Paper
正在生成论文摘要