Quantum learning of concentrated Boolean functions
Quantum Information Processing(2022)
摘要
In this paper, we present a series of new results about learning of concentrated Boolean functions in the quantum computing model. Given a Boolean function f on n variables, its concentration refers to the dominant terms in its Fourier–Walsh spectrum. We show that a quantum probabilistically approximately correct learning model to learn a Boolean function characterized by its concentration yields improvements over the best-known classical method. All of our results are presented within the framework of query complexity , and therefore, our advantage represents asymptotic improvements in the number of queries using a quantum approach over its classical counterpart. Next, we prove a lower bound in the number of quantum queries needed to learn the function in the distribution-independent settings. Further, we examine the case of exact learning which is the learning variant without error. Here, we show that the query complexity grows as 2^βn for some 0< β < 1 and therefore remains intractable even when quantum approaches are considered. This proof is based on the quantum information theoretic approach developed by researchers for the restricted case of k -sparse functions.
更多查看译文
关键词
Quantum computing, Machine learning, PAC learning, Concentrated functions
AI 理解论文
溯源树
样例
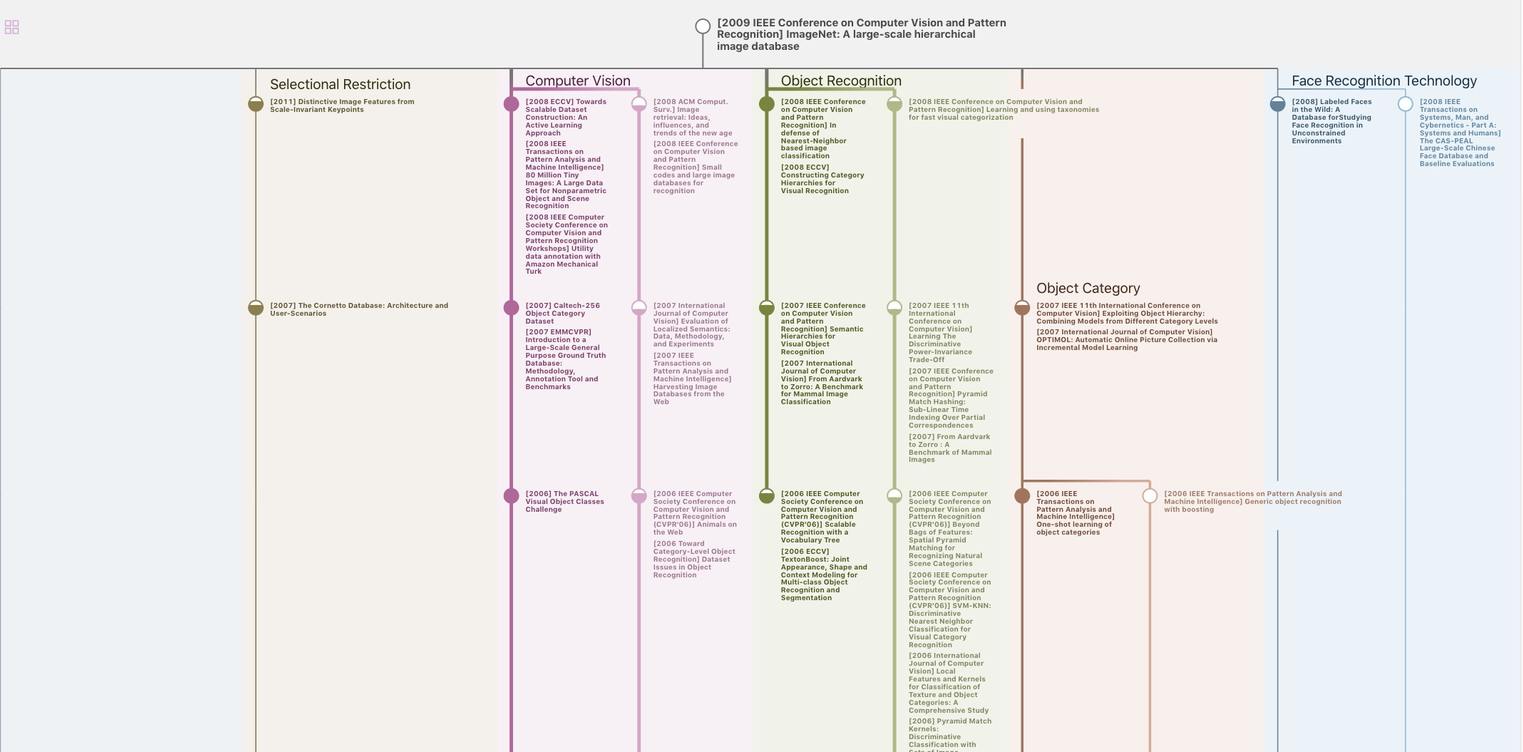
生成溯源树,研究论文发展脉络
Chat Paper
正在生成论文摘要