Hostility measure for multi-level study of data complexity
Applied Intelligence(2022)
摘要
Complexity measures aim to characterize the underlying complexity of supervised data. These measures tackle factors hindering the performance of Machine Learning ( ML ) classifiers like overlap, density, linearity, etc. The state-of-the-art has mainly focused on the dataset perspective of complexity, i.e., offering an estimation of the complexity of the whole dataset. Recently, the instance perspective has also been addressed. In this paper, the hostility measure , a complexity measure offering a multi-level (instance, class, and dataset) perspective of data complexity is proposed. The proposal is built by estimating the novel notion of hostility : the difficulty of correctly classifying a point, a class, or a whole dataset given their corresponding neighborhoods. The proposed measure is estimated at the instance level by applying the k -means algorithm in a recursive and hierarchical way, which allows to analyze how points from different classes are naturally grouped together across partitions. The instance information is aggregated to provide complexity knowledge at the class and the dataset levels. The validity of the proposal is evaluated through a variety of experiments dealing with the three perspectives and the corresponding comparative with the state-of-the-art measures. Throughout the experiments, the hostility measure has shown promising results and to be competitive, stable, and robust.
更多查看译文
关键词
Hostility measure, Complexity measures, Data complexity, Classification, Supervised problems
AI 理解论文
溯源树
样例
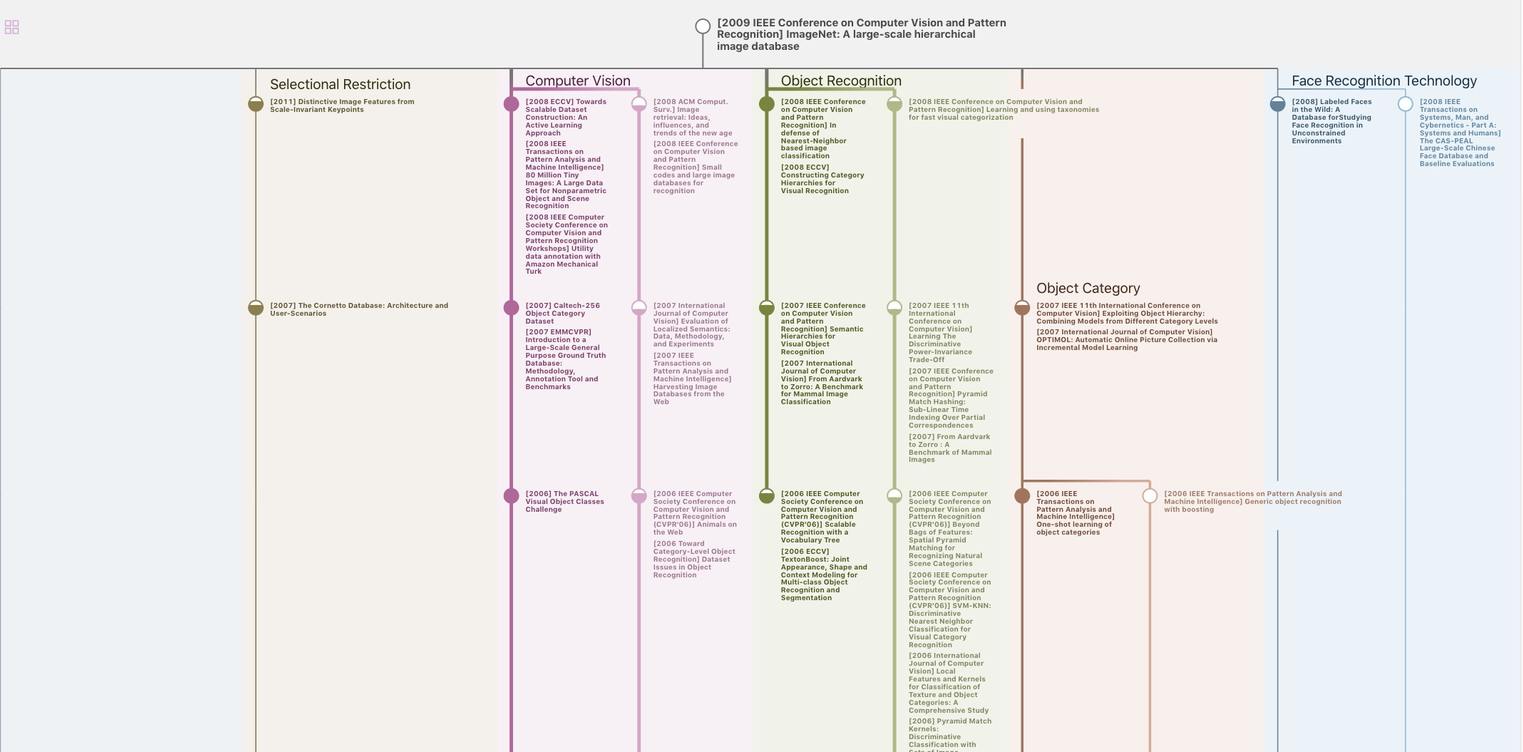
生成溯源树,研究论文发展脉络
Chat Paper
正在生成论文摘要