Intrusion Detection System: An Automatic Machine Learning Algorithms Using Auto- WEKA
2022 IEEE 13th Control and System Graduate Research Colloquium (ICSGRC)(2022)
摘要
Network Intrusion Detection Systems (NIDS) are essential to maintaining network security. This study is concerned with developing an Intrusion Detection System (IDS) based on Automated Machine Learning (AutoML) to reduce false alarms and provide accurate NIDS. The proposed model is developed using two machine learning (ML) software tools (Weka and RapidMiner). To study the performance of various ML algorithms, four different classifiers are applied to the intrusion detection dataset, namely Naïve Bayes (NB), Multilayer Perceptron (MLP), Random Forest (RF), and Sequential Minimal Optimization (SMO). Auto-WEKA is used to select the best classifier with its appropriate hyperparameters automatically. Auto-model implements the best classifier resulting from Auto- Wekain RapidMiner to answer the question, which tool among them achieves the best accuracy with minimal effort (i.e., best-suited to non-expert developers)? Finally, the performance of the classifiers (resulting from Weka, Auto-WEKA, and the Auto Model in RapidMiner) is evaluated utilizing the NLS-KDD dataset. The experimental results show that RF outperforms the other classifiers in terms of accuracy with adequate time consumption. It was also found that Auto-WEKA is the preferable one since it automatically selects the best classifier (in terms of accuracy) with its appropriate hyperparameters with minimal effort.
更多查看译文
关键词
Auto Machine Learning,Intrusion Detection,WEKA,Auto-WEKA,RapidMiner,Machine Learning
AI 理解论文
溯源树
样例
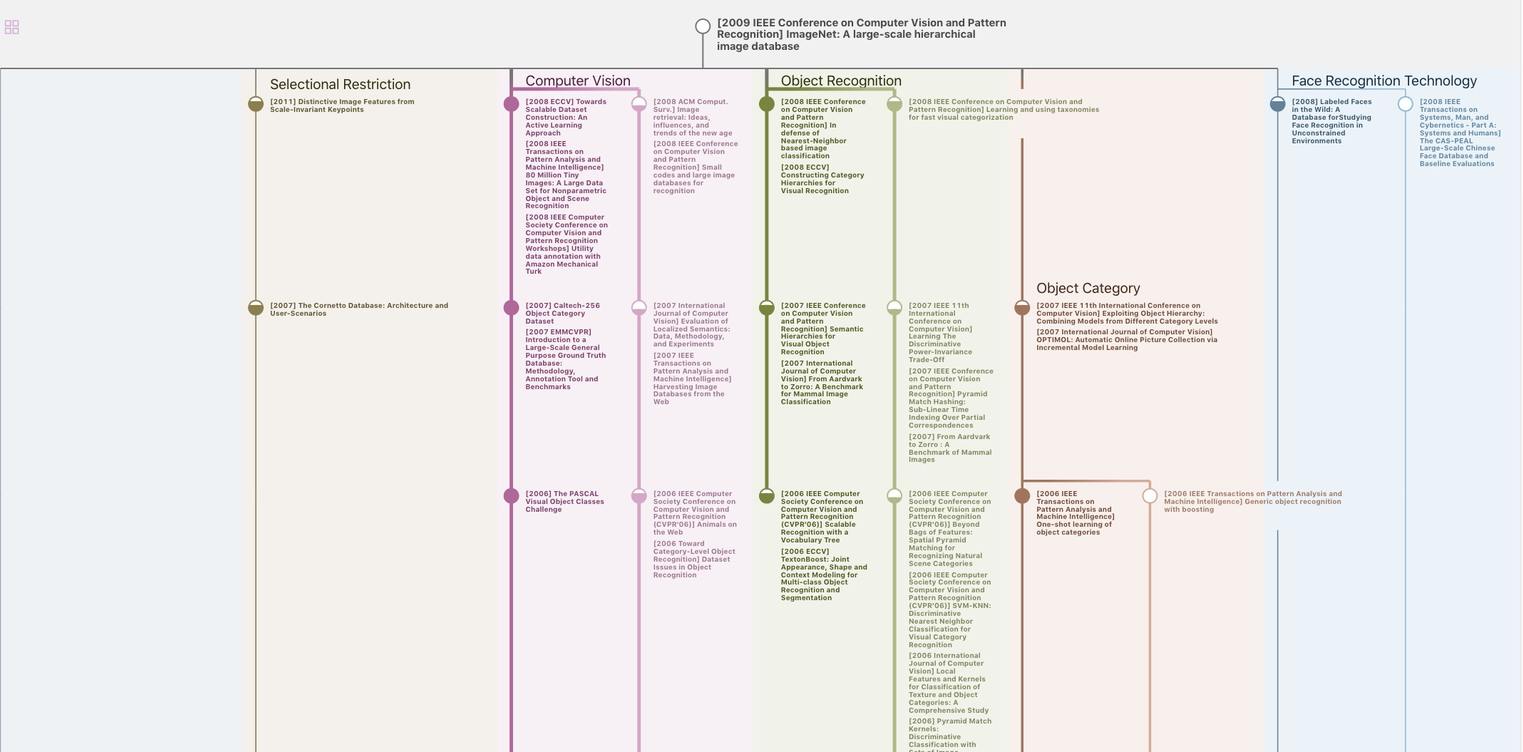
生成溯源树,研究论文发展脉络
Chat Paper
正在生成论文摘要