Application of rough set theory and machine learning algorithms in predicting accident outcomes in the Indian petroleum industry
CONCURRENCY AND COMPUTATION-PRACTICE & EXPERIENCE(2022)
摘要
Recent advancements in machine learning techniques are helping researchers to develop predictive models that assist decision-makers to get a quick, unbiased overview of the processes. But studies using machine learning approaches in analyzing and classifying the injury narratives of the petroleum industries are still in their early stages due to data unavailability and lack of trust in these models. Comparatively, other industries such as construction, manufacturing, aviation and so forth are using the findings from the predictive models but the use of machine learning techniques in analyzing petroleum industry accident data is not gaining much importance. This study aims to use available accident data from the Indian petroleum industry to develop a classification model for predicting possible outcomes of an accident. The data used in this study comprises 194 accident reports with 20 information attributes collected during the 2016-20 period. Six different machine learning algorithms are used to analyze and classify the possible outcome of the accident. It has been found that the Xgboost algorithm has achieved 95% accuracy following multilayer perceptron with 94% accuracy. The rough set theory is also used to extract the indiscernibility relationship between the given attributes causing accident occurrence. The results indicate that "skill-based error, supervisory violation, no personal protective equipment usage, and lack of standard operating procedure compliance" have contributed to the majority of the accidents. The findings of this study can be used to assist safety professionals in decision-making, mitigating the root causes of contributing factors.
更多查看译文
关键词
feature engineering,machine learning,petroleum industry,rough set theory,safety analytics,Xgboost
AI 理解论文
溯源树
样例
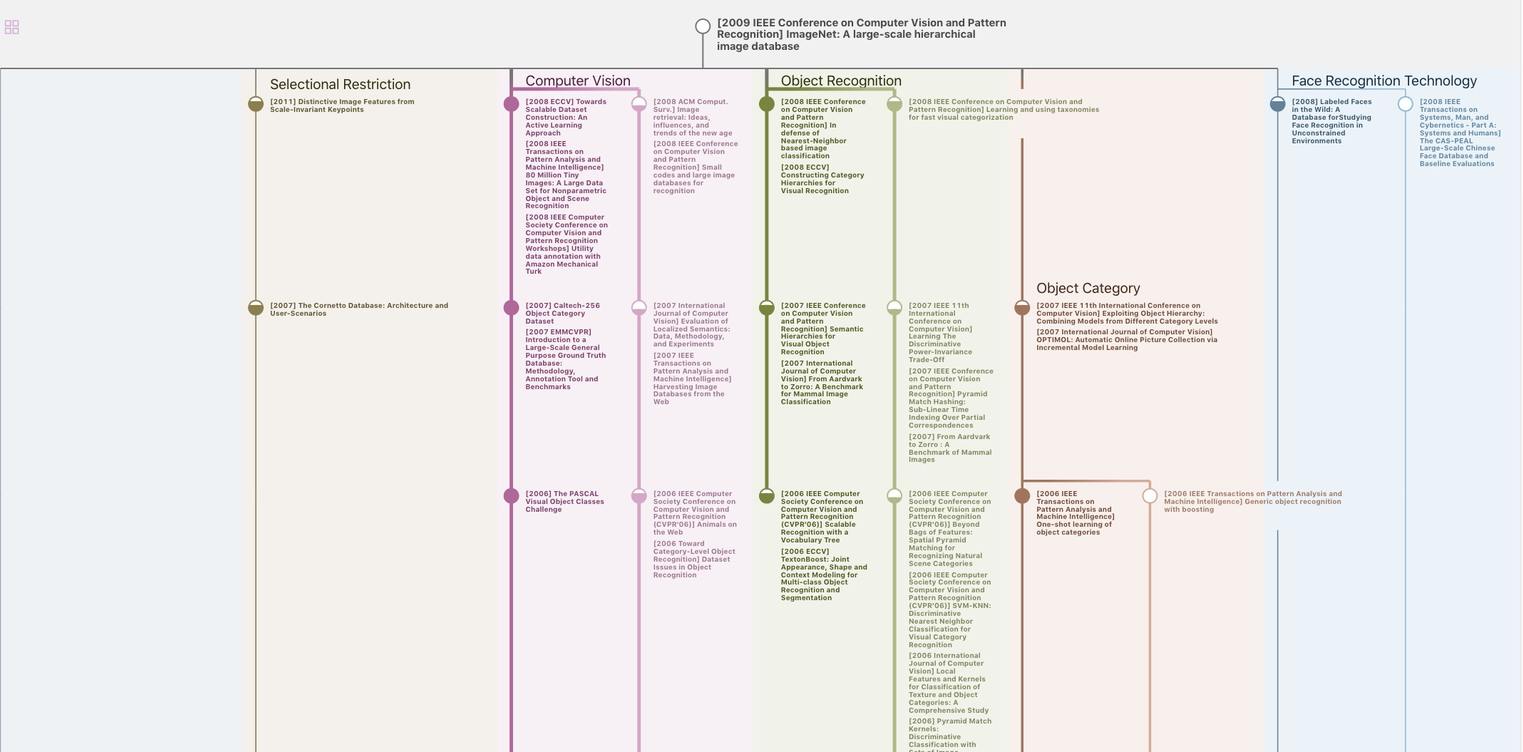
生成溯源树,研究论文发展脉络
Chat Paper
正在生成论文摘要