Towards Understanding Mixture of Experts in Deep Learning
arxiv(2022)
摘要
The Mixture-of-Experts (MoE) layer, a sparsely-activated model controlled by a router, has achieved great success in deep learning. However, the understanding of such architecture remains elusive. In this paper, we formally study how the MoE layer improves the performance of neural network learning and why the mixture model will not collapse into a single model. Our empirical results suggest that the cluster structure of the underlying problem and the non-linearity of the expert are pivotal to the success of MoE. To further understand this, we consider a challenging classification problem with intrinsic cluster structures, which is hard to learn using a single expert. Yet with the MoE layer, by choosing the experts as two-layer nonlinear convolutional neural networks (CNNs), we show that the problem can be learned successfully. Furthermore, our theory shows that the router can learn the cluster-center features, which helps divide the input complex problem into simpler linear classification sub-problems that individual experts can conquer. To our knowledge, this is the first result towards formally understanding the mechanism of the MoE layer for deep learning.
更多查看译文
关键词
deep learning,experts,mixture,understanding
AI 理解论文
溯源树
样例
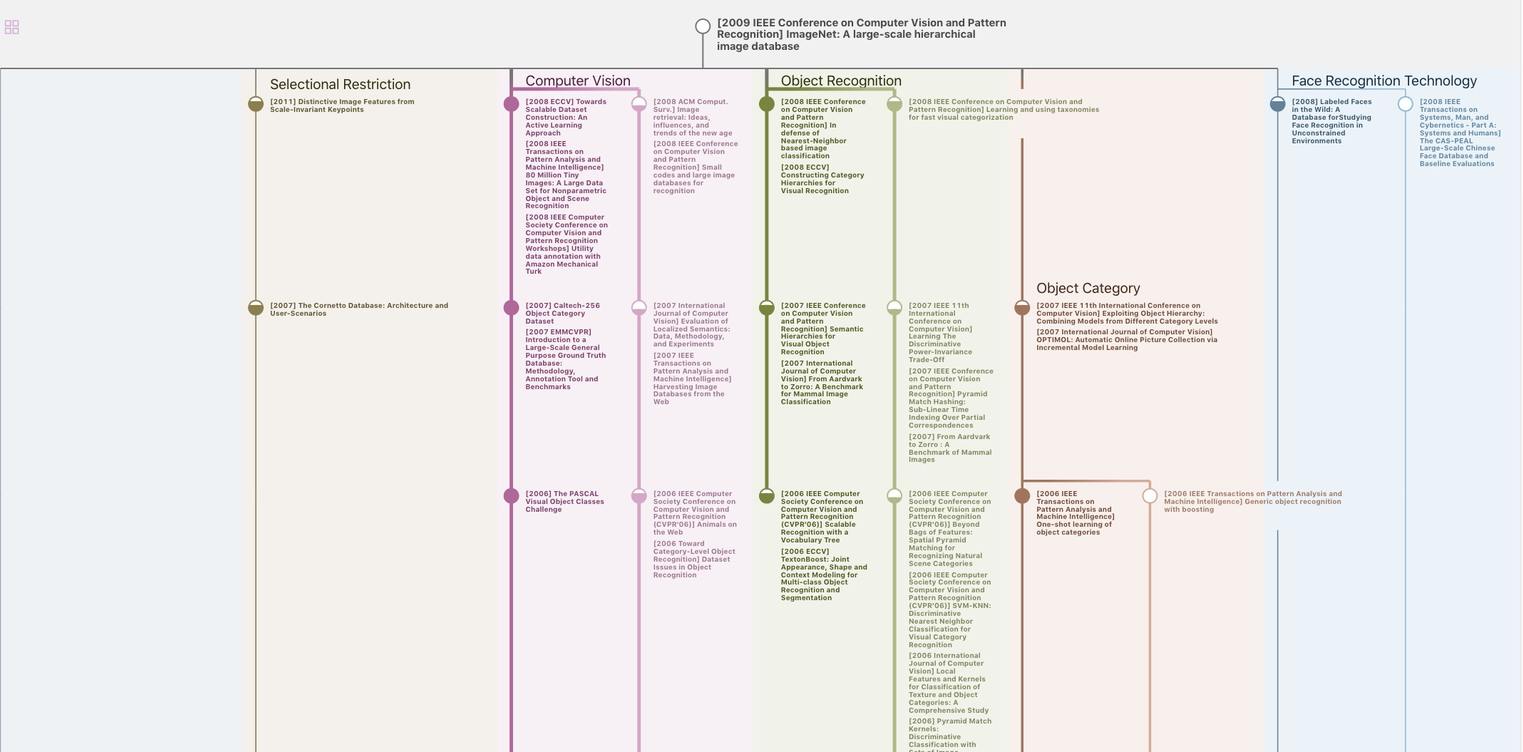
生成溯源树,研究论文发展脉络
Chat Paper
正在生成论文摘要