Dynamic Planning in Open-Ended Dialogue using Reinforcement Learning
arxiv(2022)
摘要
Despite recent advances in natural language understanding and generation, and decades of research on the development of conversational bots, building automated agents that can carry on rich open-ended conversations with humans "in the wild" remains a formidable challenge. In this work we develop a real-time, open-ended dialogue system that uses reinforcement learning (RL) to power a bot's conversational skill at scale. Our work pairs the succinct embedding of the conversation state generated using SOTA (supervised) language models with RL techniques that are particularly suited to a dynamic action space that changes as the conversation progresses. Trained using crowd-sourced data, our novel system is able to substantially exceeds the (strong) baseline supervised model with respect to several metrics of interest in a live experiment with real users of the Google Assistant.
更多查看译文
关键词
dialogue,dynamic planning,reinforcement learning,open-ended
AI 理解论文
溯源树
样例
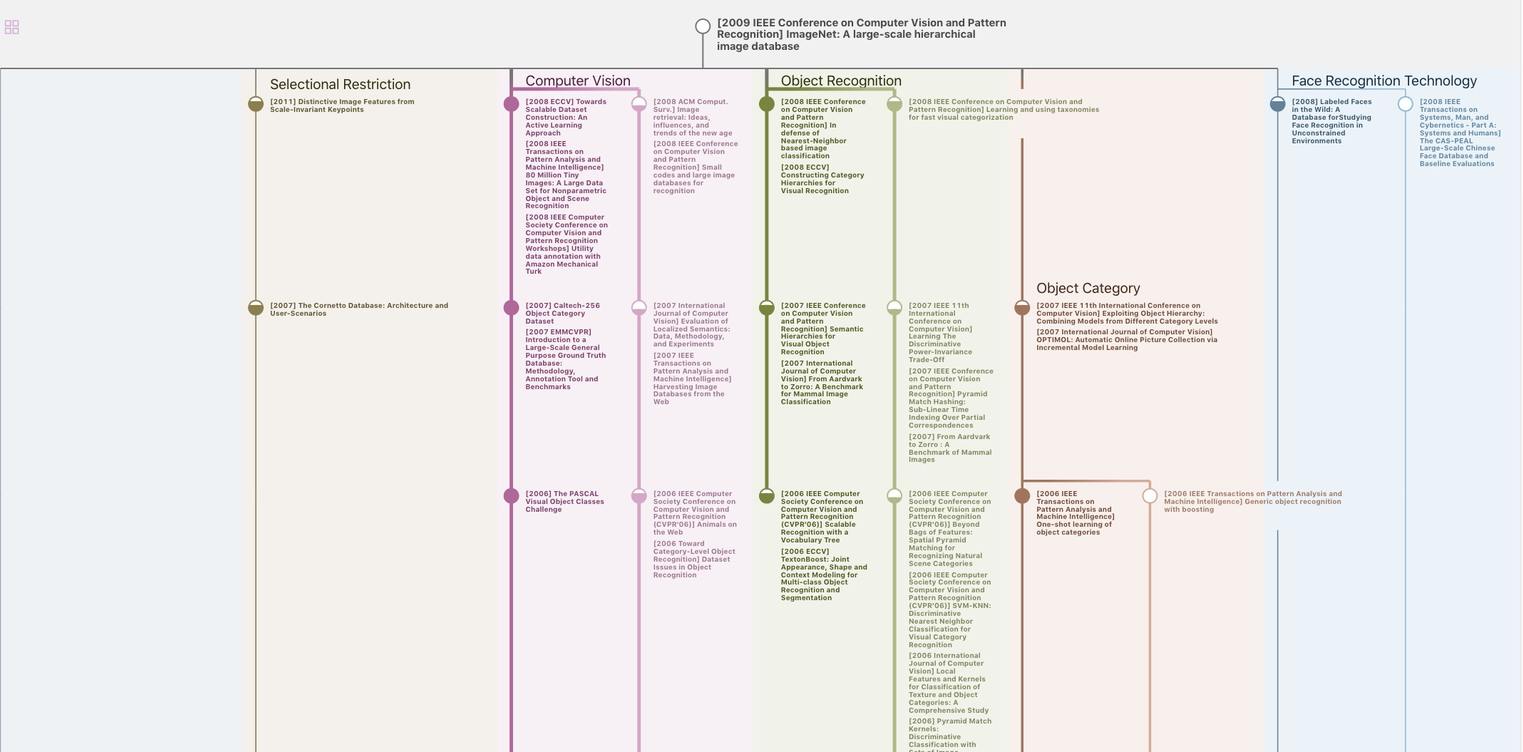
生成溯源树,研究论文发展脉络
Chat Paper
正在生成论文摘要