Divide-and-Conquer Predictor for Unbiased Scene Graph Generation
IEEE Transactions on Circuits and Systems for Video Technology(2022)
摘要
Scene Graph Generation (SGG) aims to detect the objects and their pairwise predicates in an image. Existing SGG methods mainly fulfil the challenging predicate prediction task that involves severe long-tailed data distribution with a single classifier. However, we argue that this may be enough to differentiate predicates that present obvious differences (e.g.,
$on$
and
$near$
), but not sufficient to distinguish similar predicates that only have subtle differences (e.g.,
$on$
and
$standing~on$
). Towards this end, we divide the predicate prediction into a few sub-tasks with a Divide-and-Conquer Predictor (DC-Predictor). Specifically, we first develop an offline pattern-predicate correlation mining algorithm to discover the similar predicates that share the same object interaction pattern. Based on that, we devise a general pattern classifier and a set of specific predicate classifiers for DC-Predictor. The former works on recognizing the pattern of a given object pair and routing it to the corresponding specific predicate classifier, while the latter aims to differentiate similar predicates in each specific pattern. In addition, we introduce the Bayesian Personalized Ranking loss in each specific predicate classifier to enhance the pairwise differentiation between head predicates and their similar ones. Experiments on VG150 and GQA datasets show the superiority of our model over state-of-the-art methods.
更多查看译文
关键词
Scene graph generation,vision and language,divide-and-conquer,Bayesian personalized ranking
AI 理解论文
溯源树
样例
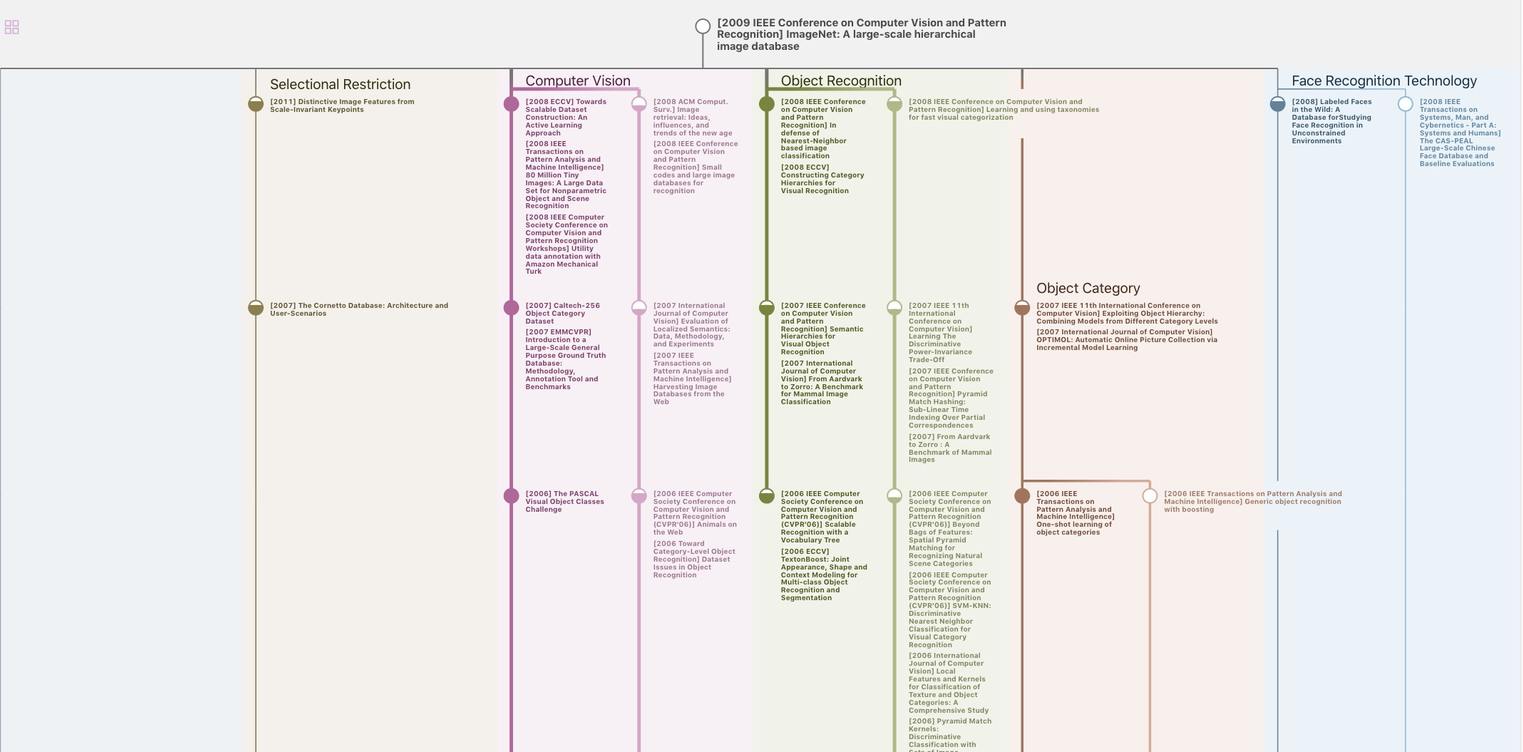
生成溯源树,研究论文发展脉络
Chat Paper
正在生成论文摘要