An Auto Encoder-based Dimensionality Reduction Technique for Efficient Entity Linking in Business Phone Conversations
SIGIR '22: Proceedings of the 45th International ACM SIGIR Conference on Research and Development in Information Retrieval(2022)
摘要
An entity linking system links named entities in a text to their corresponding entries in a knowledge base. In recent years, building an entity linking system that leverages the transformer architecture has gained lots of attention. However, deploying a transformer-based neural entity linking system in industrial production environments in a limited resource setting is a challenging task. In this work, we present an entity linking system that leverages a transformer-based BERT encoder (the BLINK model) to connect the product and organization type entities in business phone conversations to their corresponding Wikipedia entries. We propose a dimensionality reduction technique via utilizing an auto encoder that can effectively compress the dimension of the pre-trained BERT embeddings to 256 from the original size of 1024. This allows our entity linking system to significantly optimize the space requirement when deployed in a resource limited cloud machine while reducing the inference time along with retaining high accuracy.
更多查看译文
关键词
Entity Linking, BLINK, Dimensionality Reduction, Auto Encoder, BERT, Elasticsearch
AI 理解论文
溯源树
样例
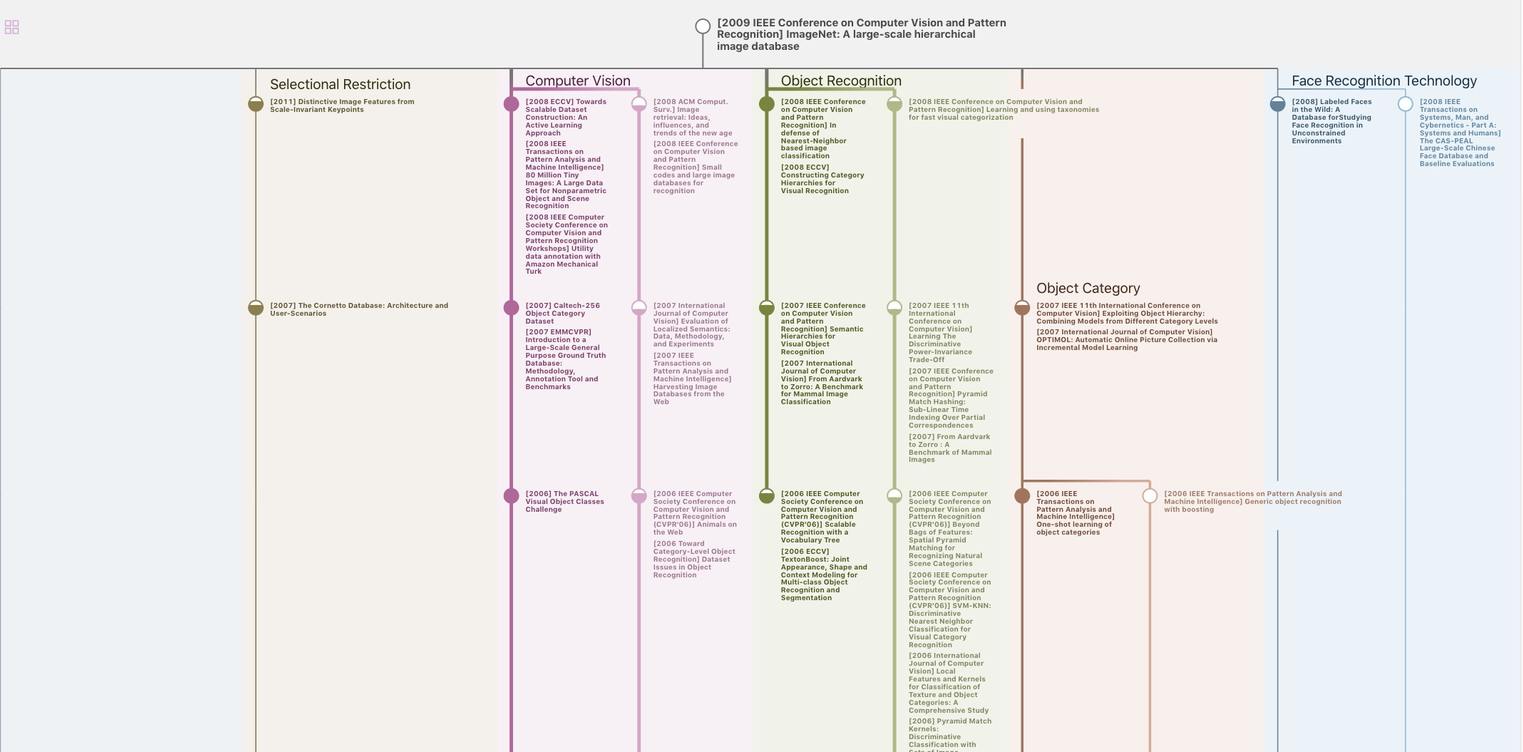
生成溯源树,研究论文发展脉络
Chat Paper
正在生成论文摘要