Don't Forget Me: Accurate Background Recovery for Text Removal via Modeling Local-Global Context.
European Conference on Computer Vision(2022)
摘要
Text removal has attracted increasingly attention due to its various applications on privacy protection, document restoration, and text editing. It has shown significant progress with deep neural network. However, most of the existing methods often generate inconsistent results for complex background. To address this issue, we propose a Contextual-guided Text Removal Network, termed as CTRNet. CTRNet explores both low-level structure and high-level discriminative context feature as prior knowledge to guide the process of background restoration. We further propose a Local-global Content Modeling (LGCM) block with CNNs and Transformer-Encoder to capture local features and establish the long-term relationship among pixels globally. Finally, we incorporate LGCM with context guidance for feature modeling and decoding. Experiments on benchmark datasets, SCUT-EnsText and SCUT-Syn show that CTRNet significantly outperforms the existing state-of-the-art methods. Furthermore, a qualitative experiment on examination papers also demonstrates the generalization ability of our method. The codes and supplement materials are available at https://github.com/lcy0604/CTRNet.
更多查看译文
关键词
GAN,Text removal,Context guidance,Transformer
AI 理解论文
溯源树
样例
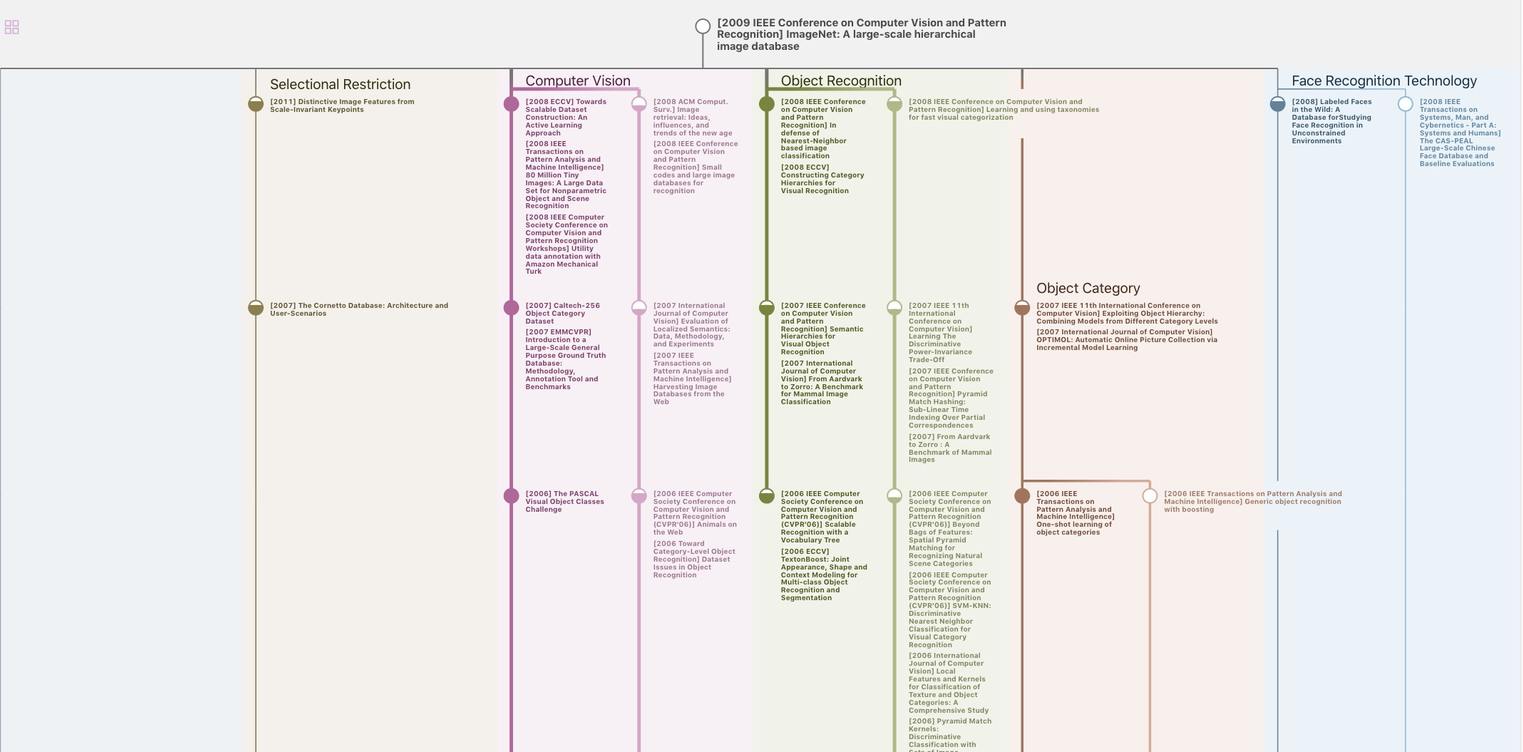
生成溯源树,研究论文发展脉络
Chat Paper
正在生成论文摘要