A novel invasive plant detection approach using time series images from unmanned aerial systems based on convolutional and recurrent neural networks
NEURAL COMPUTING & APPLICATIONS(2022)
摘要
Accurate identification of invasive plants (IPs) is critical for the preservation of natural ecosystems. Unmanned Aerial Systems (UAS) offer an efficient method of monitoring IPs in conservation areas. However, the process of finding IPs in UAS is labor-intensive and error-prone. Deep learning techniques have the potential for object detection and increase the efficiency and accuracy of IP identification. This study develops a novel deep learning model using a UAS and associated geographic information system (GIS) to detect and identify invasive Phragmites australis (PA) in its early growth stage. This novel network, integrating a convolutional neural network (CNN) and a recurrent neural network (RNN), proves to be an efficient and accurate method to detect PA in time series UAS images. CNN is good at extracting spatial features on images, and RNN excels in processing temporal features. Temporal features of the time series UAS images are extracted and employed with spatial features for the classification by an RNN model. Experiments are conducted in Nature Conservancy’s Emiquon Preserve. The proposed network achieves the highest performance compared with other IP detection models. With its high generalization ability, the proposed model might find more applications in precision agriculture and land conservation areas.
更多查看译文
关键词
Invasive plant,Unmanned aerial system,Convolutional neural network,Recurrent neural network
AI 理解论文
溯源树
样例
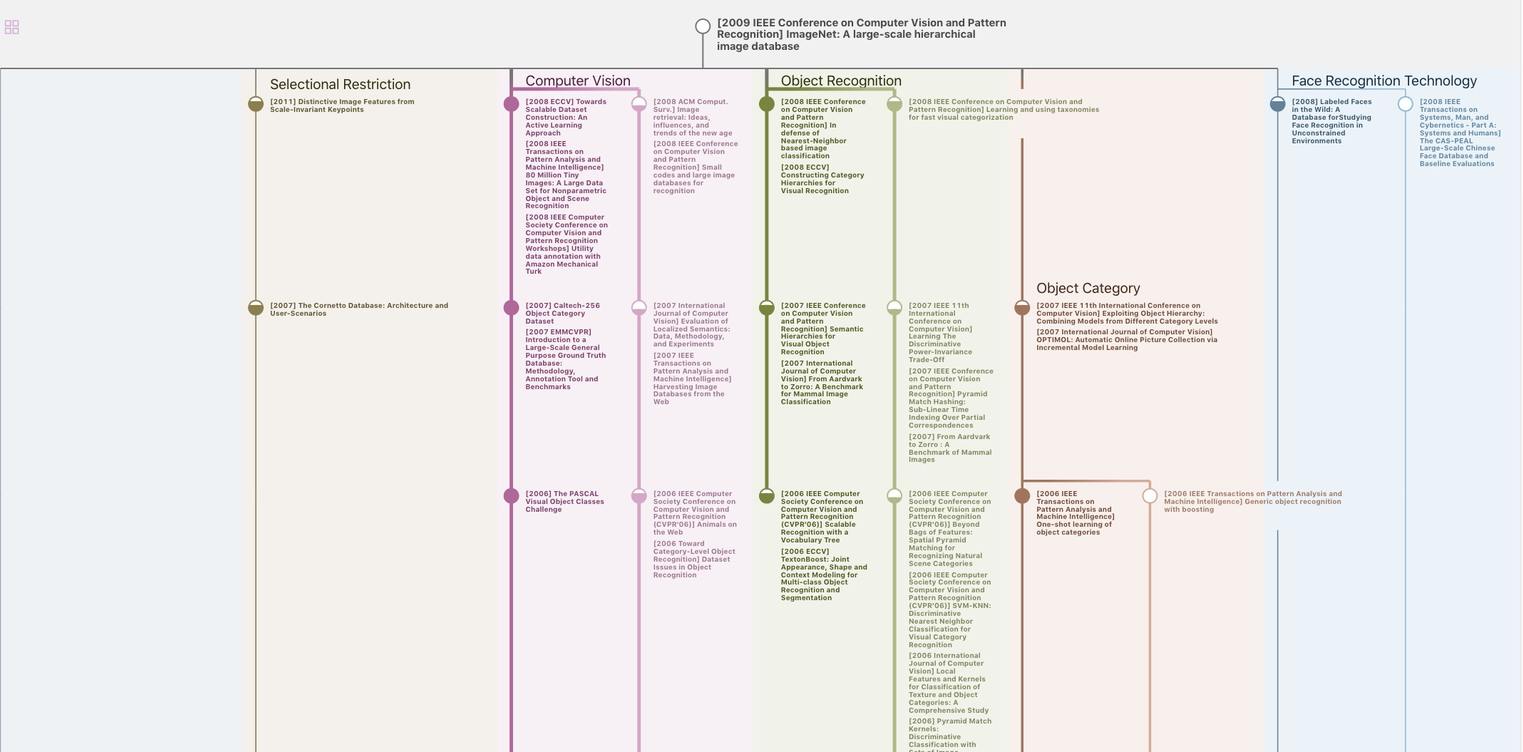
生成溯源树,研究论文发展脉络
Chat Paper
正在生成论文摘要