Semantic Compression Embedding for Generative Zero-Shot Learning
Proceedings of the Thirty-First International Joint Conference on Artificial Intelligence(2022)
摘要
Generative methods have been successfully applied in zero-shot learning (ZSL) by learning an implicit mapping to alleviate the visual-semantic domain gaps and synthesizing unseen samples to handle the data imbalance between seen and unseen classes. However, existing generative methods simply use visual features extracted by the pre-trained CNN backbone. These visual features lack attribute-level semantic information. Consequently, seen classes are indistinguishable, and the knowledge transfer from seen to unseen classes is limited. To tackle this issue, we propose a novel Semantic Compression Embedding Guided Generation (SC-EGG) model, which cascades a semantic compression embedding network (SCEN) and an embedding guided generative network (EGGN). The SCEN extracts a group of attribute-level local features for each sample and further compresses them into the new low-dimension visual feature. Thus, a dense-semantic visual space is obtained. The EGGN learns a mapping from the class-level semantic space to the dense-semantic visual space, thus improving the discriminability of the synthesized dense-semantic unseen visual features. Extensive experiments on three benchmark datasets, i.e., CUB, SUN and AWA2, demonstrate the significant performance gains of SC-EGG over current state-of-the-art methods and its baselines.
更多查看译文
AI 理解论文
溯源树
样例
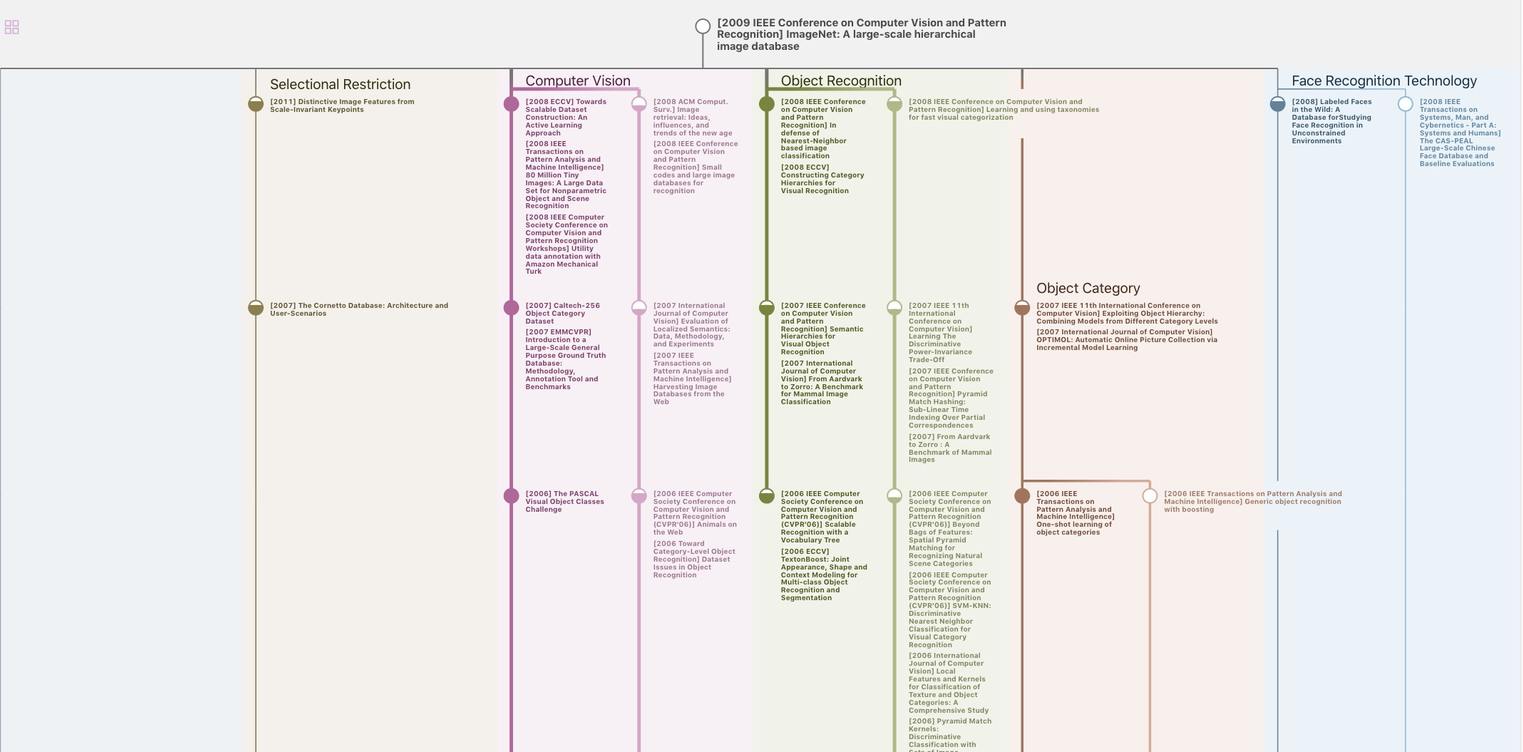
生成溯源树,研究论文发展脉络
Chat Paper
正在生成论文摘要