EDLA-EFDS: A Novel Ensemble Deep Learning Approach For Electrical Fault Detection Systems
ELECTRIC POWER SYSTEMS RESEARCH(2022)
摘要
Early detection of electrical faults is a very essential research area due to its positive influence on network stability and customer satisfaction. Despite of the electrical fault detection problem has been researched during the last decade, the existence of an intelligent fault detection system is still rare in real-world applications. Therefore, this study proposes a novel Ensemble Deep Learning Approach for Electrical Fault Detection Systems (EDLA-EFDS) that resolves the limitations of existing systems such as automation, validation, and overfitting. The proposed approach benefits from two phases prior to the training phase, namely, data preprocessing and pre training. Whereas the data preprocessing phase manages data by executing all elementary operations on the raw data, the pre-training phase selects both optimal features and hyperparameters by exploiting a double Particle Swarm Optimization (PSO) metaheuristic. Thereafter, a bagging ensemble system is deployed from three different deep learning paradigms, namely, Deep Neural Networks (DNN), Long Short-Term Memory recurrent Neural Networks (LSTM-RNN), and Deep Belief Networks (DBN). The ensemble system is followed by a majority voting engine to produce the final decision. Moreover, the performance of the ensemble system leveraged by the proposed approach is measured on the VSB dataset which is a modern and realistic dataset for power line fault detection. Finally, the analysis of the results using various scenarios and aspects such as the Receiver Operating Characteristic (ROC) curves and Friedman test is provided. The experimental results confirm the effectiveness of the proposed approach in solving the electrical fault detection problem.
更多查看译文
关键词
Electrical Fault Detection, Deep Learning, Ensemble System, Feature Extraction, Feature-Hyperparameter Selection, Particle Swarm Optimization
AI 理解论文
溯源树
样例
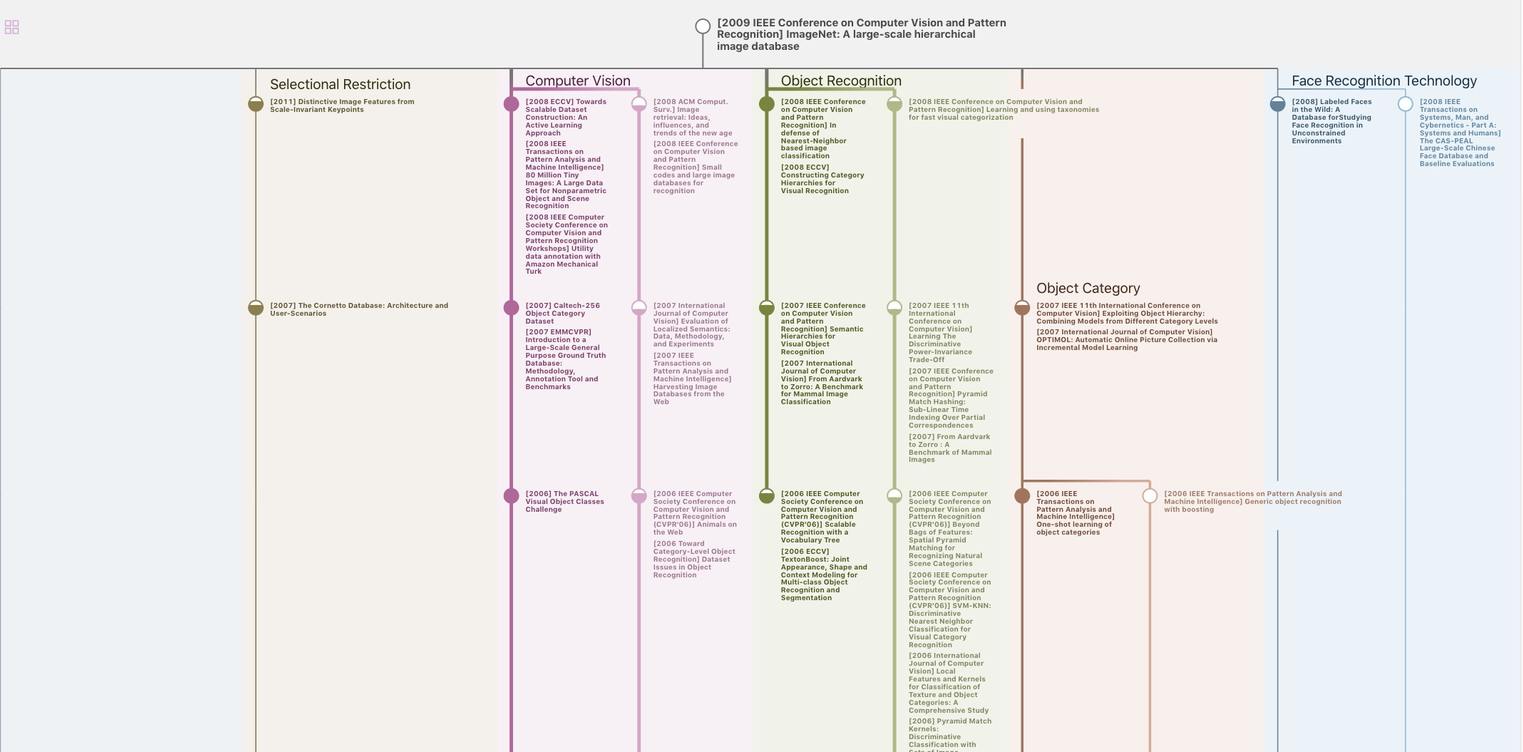
生成溯源树,研究论文发展脉络
Chat Paper
正在生成论文摘要