Power transformation of variables for post-processing precipitation forecasts: Regionally versus locally optimized parameter values
JOURNAL OF HYDROLOGY(2022)
摘要
Short-term precipitation forecasts are mainly derived from numerical weather prediction (NWP) models. Raw NWP forecasts typically require post-processing to improve their accuracy and reliability through statistical calibration. For post-processing precipitation forecasts, several well-known calibration models employ power transformation to remove the positive skewness of precipitation. The most common practice is to use a pre-fixed transformation parameter value for both observation and forecast variables. Another approach is to allow the parameter values to differ for the two variables and locally optimize them at a spatial point to ensure the best performance of a calibration model. However, when calibrating forecasts across many grid points in a region, there is a considerable advantage in keeping the transformation parameter values constant, to enable spatial consistency in the calibration model so that model information transfers from gauged to ungauged locations can be easily achieved. For this reason, a third approach is proposed here to regionally optimize the parameter values for the two variables. A question then arises: how do the three different approaches affect forecast calibration performance? This question is answered in this study by evaluating the calibration performance of precipitation forecasts across 20 locations in Australia. The pre-fixed one parameter value approach is found to lead to poor performance. The regionally optimized two parameter value approach is almost as good as the locally optimized two parameter value approach, but has the additional advantage of spatial consistency for regional applications.
更多查看译文
关键词
Data transformation, Numerical weather prediction, Post-processing, Precipitation, Forecast verification, Australia
AI 理解论文
溯源树
样例
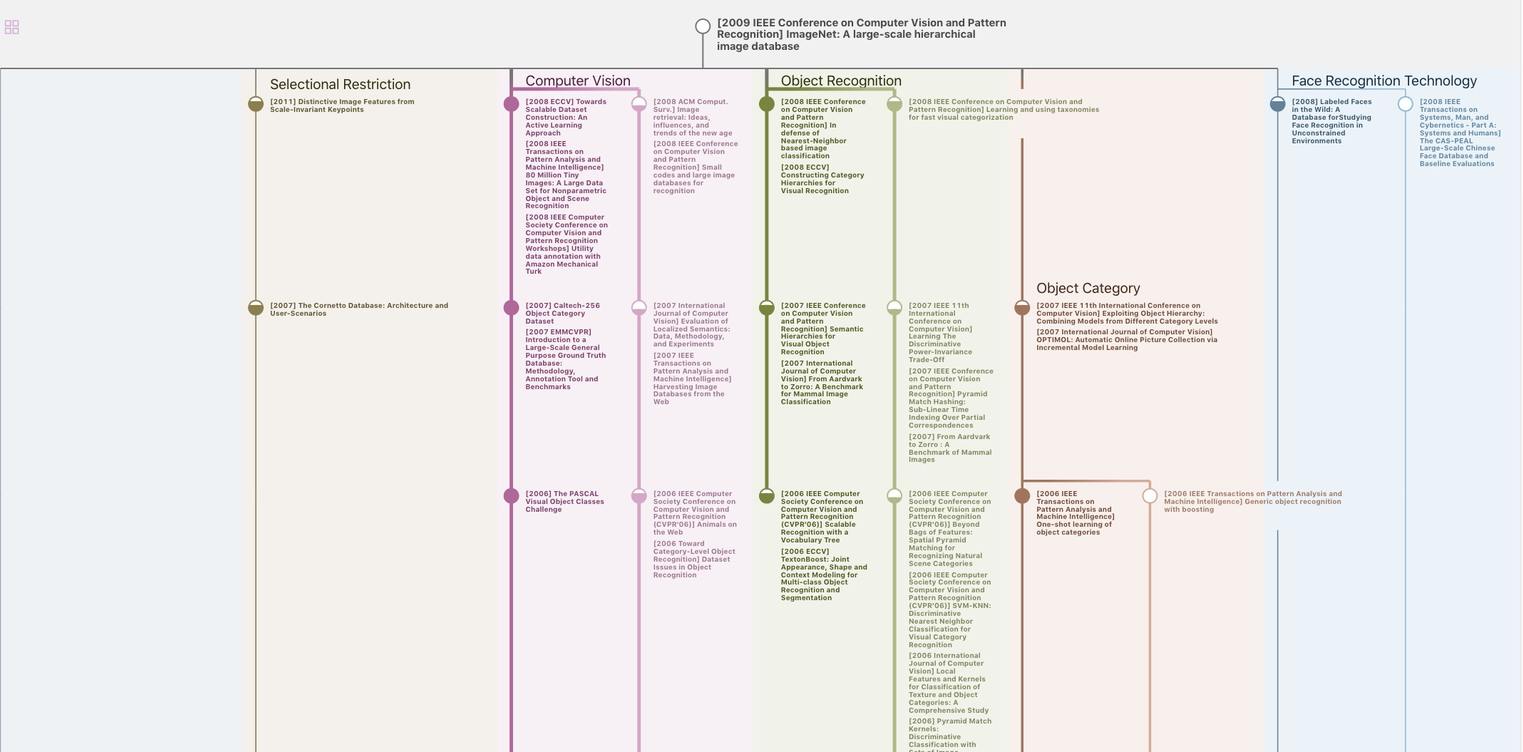
生成溯源树,研究论文发展脉络
Chat Paper
正在生成论文摘要