Underwater image super-resolution and enhancement via progressive frequency-interleaved network
Journal of Visual Communication and Image Representation(2022)
摘要
Underwater images usually contain severely blurred details, color distortion, and low contrast, warranting efficient methods to obtain clean images. However, most convolutional neural network-based approaches involve high computational cost, numerous model parameters, and even poor performance. Besides, the mapping from input to output is learned using a single path, ignoring the frequency domain information. To solve these challenges, we propose a novel progressive frequency-interleaved network (PFIN) for underwater imagery super-resolution and enhancement. Specifically, progressive frequency-domain module (PFDM) and convolution-guided module (CGM) constitute PFIN for effective color deviation correction and detail enhancement. PFDM that possesses global spatial attention, multi-scale residual, and frequency information modulation blocks gradually learn frequency features and explicitly compensate for detail loss. Furthermore, CGM comprising a series of convolution blocks generates discriminative characteristics to modulate in PFDM for better accommodating degraded representations. Extensive experiments demonstrate the superiority of our PFIN regarding quantitative evaluations and visual quality.
更多查看译文
关键词
Underwater image,Super-resolution,Enhancement,Deep learning,Frequency domain
AI 理解论文
溯源树
样例
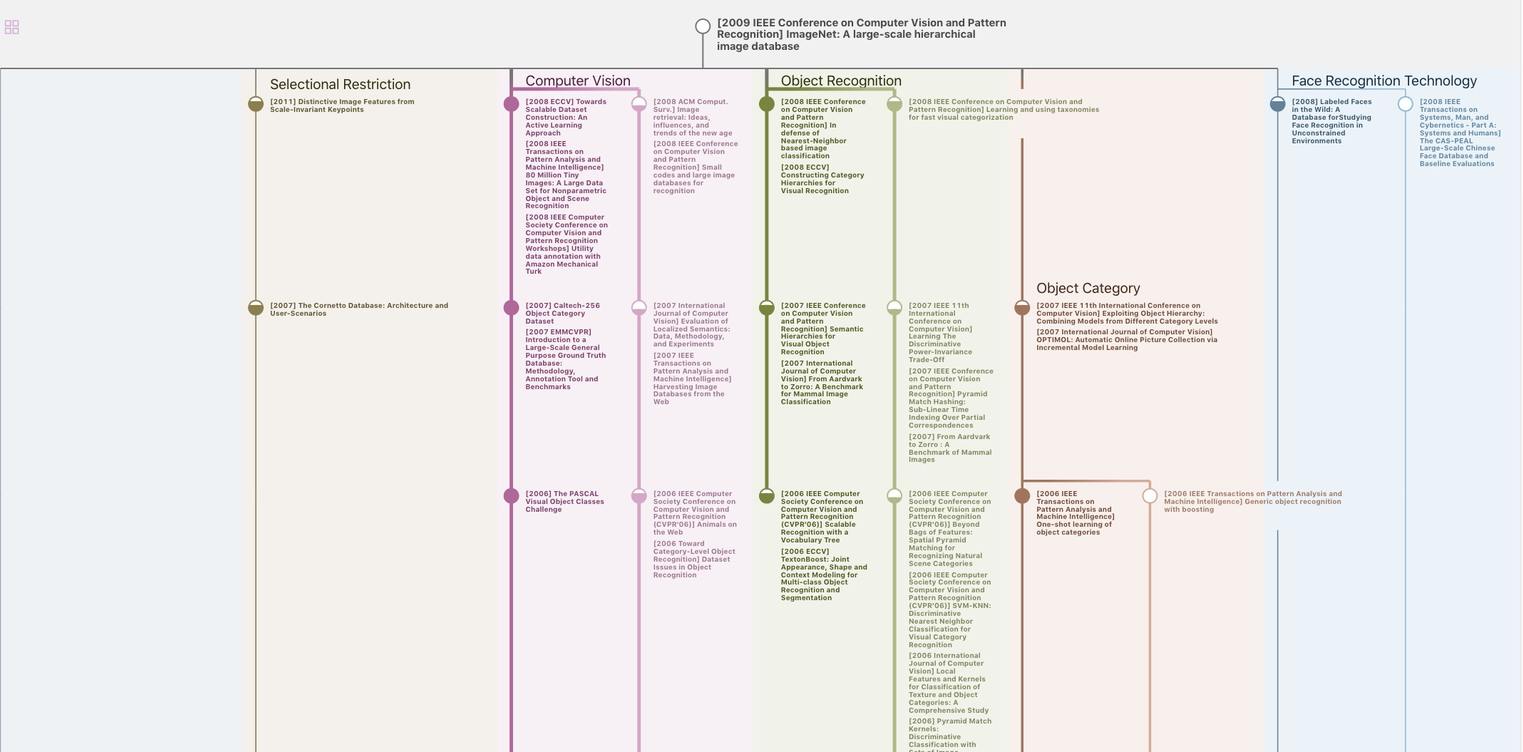
生成溯源树,研究论文发展脉络
Chat Paper
正在生成论文摘要