Impacts of the Lagrangian Data Assimilation of Surface Drifters on Estimating Ocean Circulation during the Gulf of Mexico Grand Lagrangian Deployment
MONTHLY WEATHER REVIEW(2022)
摘要
Satellite-tracked in situ surface drifters, providing measurements of near-surface ocean quantities, have become increasingly prevalent in the global ocean observation system. However, the position data from these instruments are typically not leveraged in operational ocean data assimilation (DA) systems. In this work, the impact of an augmented-state Lagrangian data assimilation (LaDA) method using the local ensemble Kalman transform filter is investigated within a realistic regional ocean DA system. Direct positioning data of surface drifters released by the Consortium for Advanced Research on Transport of Hydrocarbon in the Environment during the summer 2012 Grand Lagrangian Deployment Experiment are assimilated using a Gulf of Mexico (GoM) configuration of the Modular Ocean Model, version 6, of the Geophysical Fluid Dynamics Laboratory. Multiple cases are tested using both 1/4 degrees eddy-permitting and 1/12 degrees eddy-resolving model resolutions: 1) a free running model simulation, 2) a conventional assimilation of temperature and salinity profile observations, 3) an assimilation of profiles and Lagrangian surface drifter positions, and 4) an assimilation of the profiles and derived Eulerian velocities. LaDA generally produces more accurate estimates of all fields compared to the assimilation of derived Eulerian velocities, with estimates of surface currents notably improving, when transitioning to 1/12 degrees model resolution. In particular, LaDA produces the most accurate estimates of sea surface velocities under tropical cyclone conditions when Hurricane Isaac (2012) impacted the GoM. Further experiments applying a vertical localization while assimilating surface drifter positions improve the estimates of temperature and salinity below the mixed layer depth. Cases including the surface drifter positions in the DA show better Lagrangian predictability than the conventional DA.
更多查看译文
关键词
Lagrangian circulation, transport, In situ oceanic observations, Kalman filters, Ensembles, Data assimilation, Nonlinear models
AI 理解论文
溯源树
样例
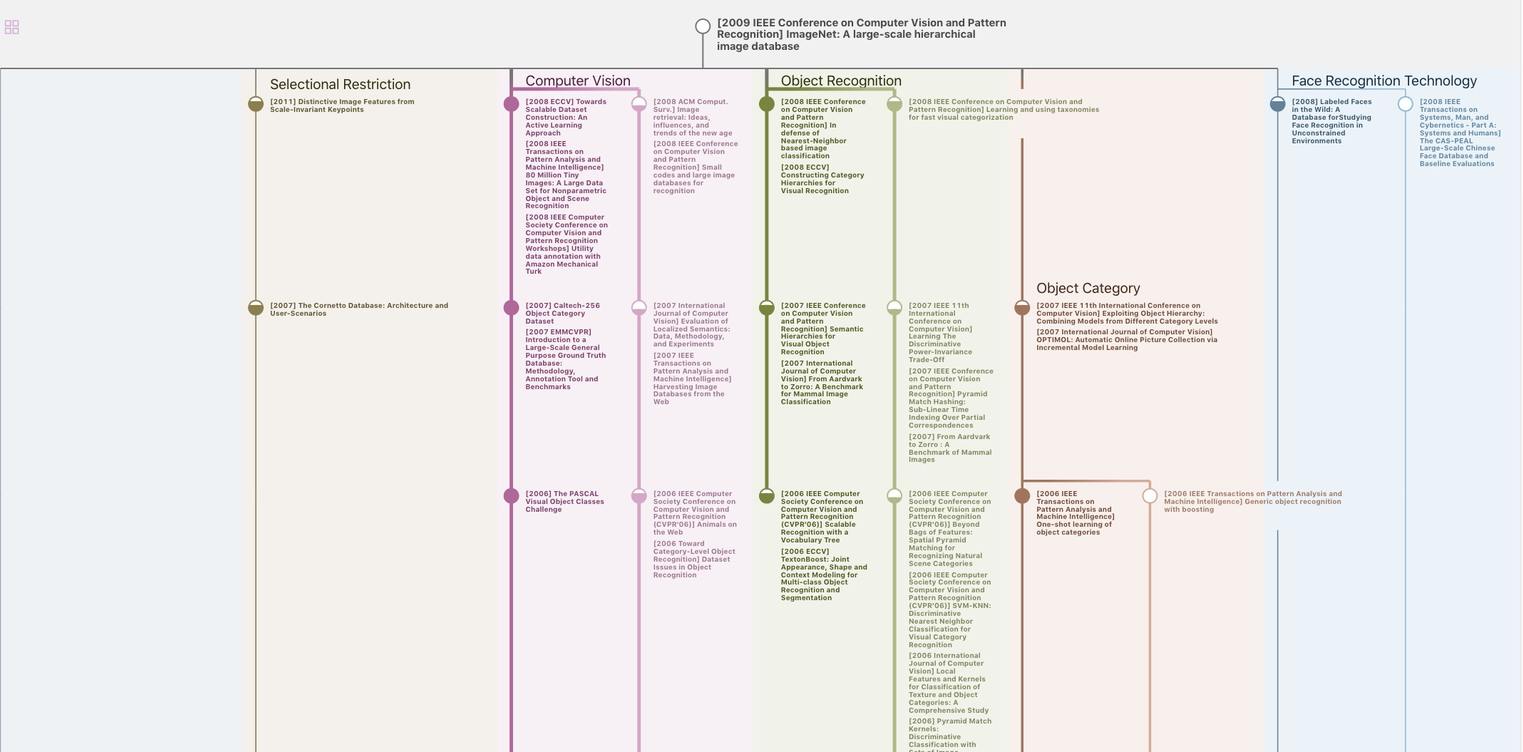
生成溯源树,研究论文发展脉络
Chat Paper
正在生成论文摘要