A New Bilinear Supervised Neighborhood Discrete Discriminant Hashing
MATHEMATICS(2022)
摘要
Feature extraction is an important part of perceptual hashing. How to compress the robust features of images into hash codes has become a hot research topic. Converting a two-dimensional image into a one-dimensional descriptor requires a higher computational cost and is not optimal. In order to maintain the internal feature structure of the original two-dimensional image, a new Bilinear Supervised Neighborhood Discrete Discriminant Hashing (BNDDH) algorithm is proposed in this paper. Firstly, the algorithm constructs two new neighborhood graphs to maintain the geometric relationship between samples and reduces the quantization loss by directly constraining the hash codes. Secondly, two small rotation matrices are used to realize the bilinear projection of the two-dimensional descriptor. Finally, the experiment verifies the performance of the BNDDH algorithm under different feature types, such as image original pixels and a Convolutional Neural Network (CNN)-based AlexConv5 feature. The experimental results and discussion clearly show that the proposed BNDDH algorithm is better than the existing traditional hashing algorithm and can represent the image more efficiently in this paper.
更多查看译文
关键词
feature extraction, perceptual hashing, discrete optimization, bilinear projection, neighborhood relationship
AI 理解论文
溯源树
样例
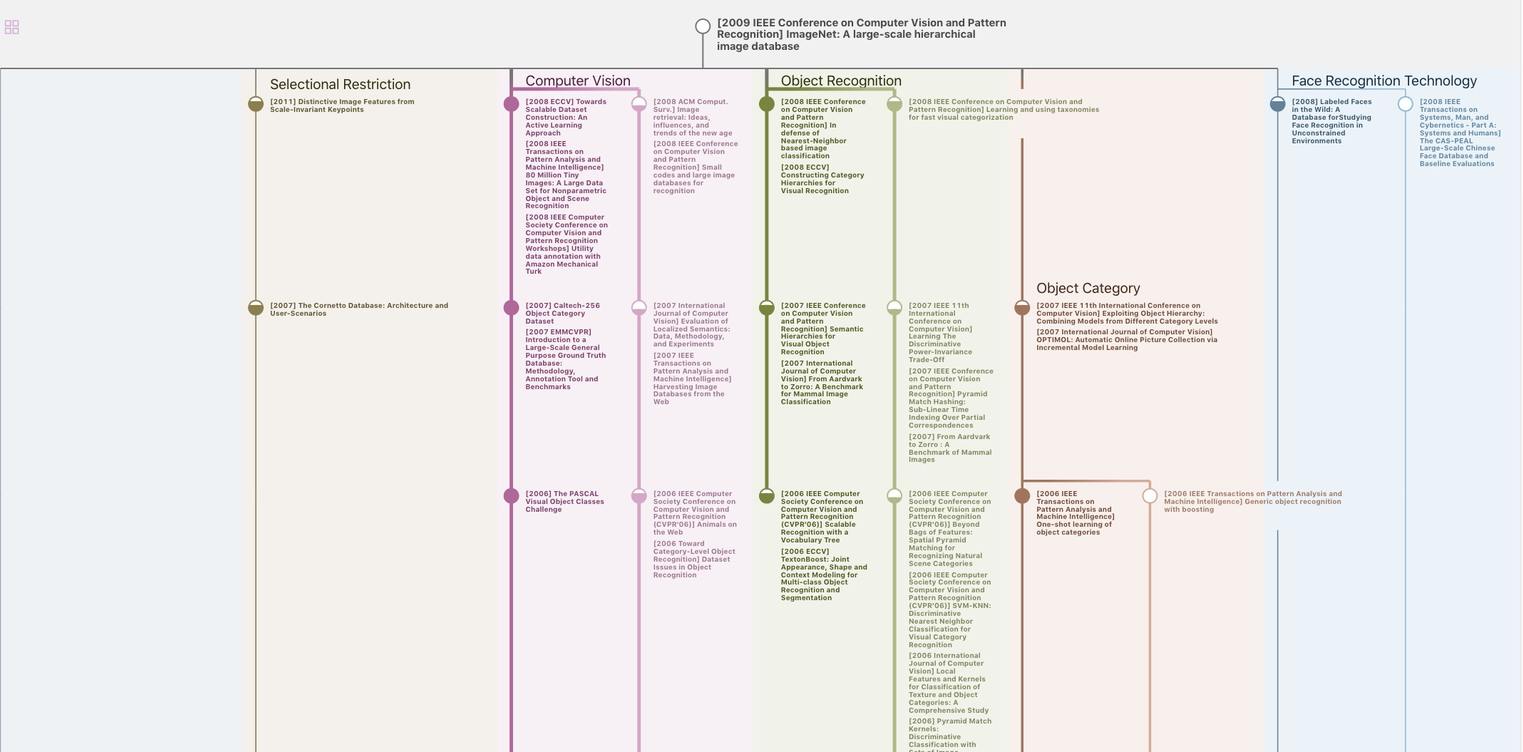
生成溯源树,研究论文发展脉络
Chat Paper
正在生成论文摘要