Salient and consensus representation learning based incomplete multiview clustering
APPLIED INTELLIGENCE(2022)
摘要
Multiview learning has a great potential to achieve a better performance than the conventional single-view based methods. However, some views of multiview data may be missing in the real-world applications. To cluster such incomplete multiview data, researchers tend to fill in the absent instances with the average vector of the available data for each view or adopt the pre-defined graphs from different views to learn a consensus representation. These approaches neither achieve the real distribution of the data nor explore the salient structure for each view influenced by noise. To address these problems, we propose a salient and consensus representation learning based method for incomplete multiview clustering (SCR_IMC). Firstly, we adaptively learn the salient structures of the collected data from different views adopting the self-representation property of the data. Then, the salient structures from different views are translated into the complete sub-spaces by a set of pre-defined projection matrices. Finally, a salient and consensus representation reflecting the common structure across different views is learned for the final clustering task. Experimental results on several real-world databases illustrate that the proposed method achieves competitive performance in comparison with the other classical and state-of-the-art methods, which proves the effectiveness of the proposed method.
更多查看译文
关键词
Incomplete multiview clustering, Salient and consensus representation, Salient structure learning, Complete structure projection
AI 理解论文
溯源树
样例
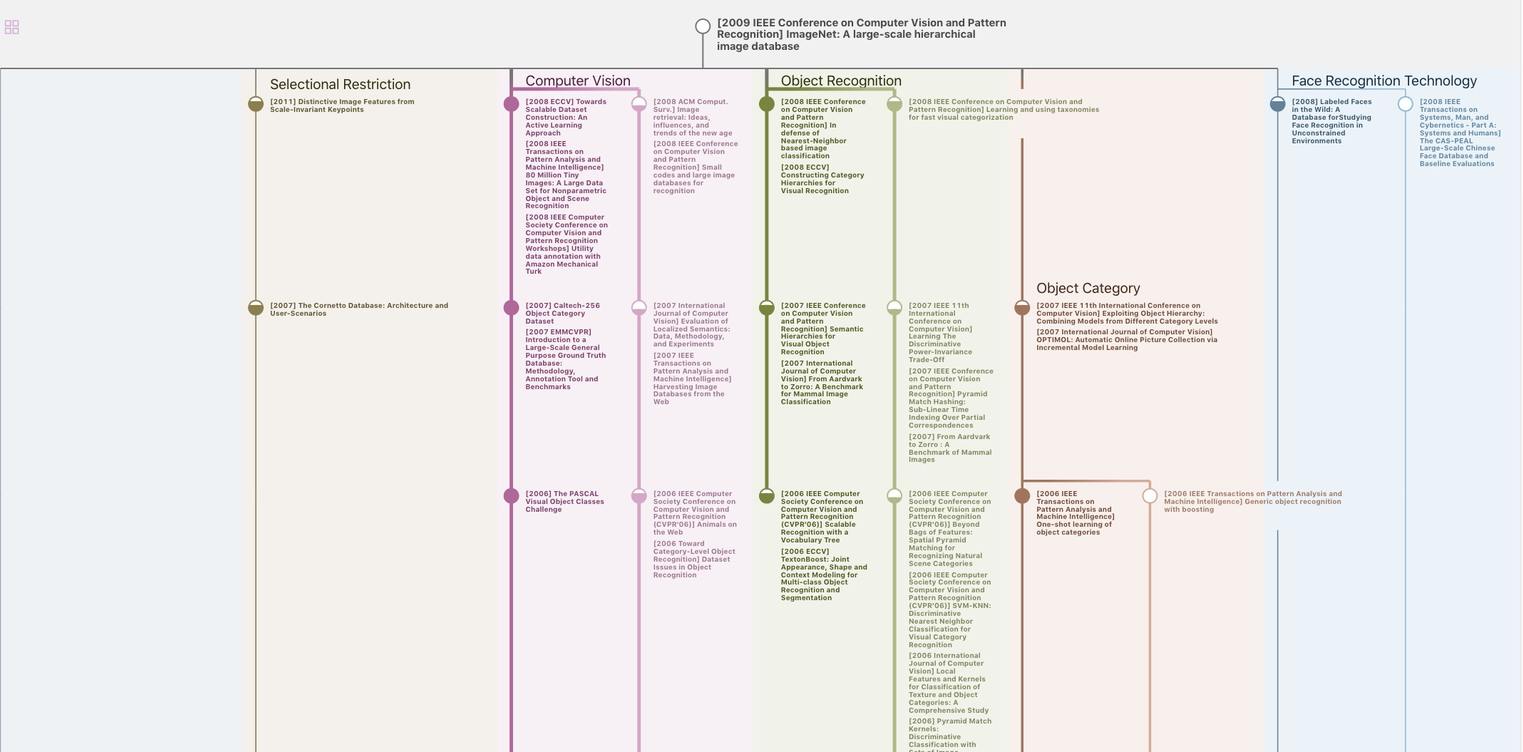
生成溯源树,研究论文发展脉络
Chat Paper
正在生成论文摘要