A Deep Embedded Clustering Algorithm for the Binning of Metagenomic Sequences
IEEE ACCESS(2022)
摘要
The study of metagenomic sequences brings a deep understanding of microbial communities. One of the crucial steps in metagenomic projects is to classify sequences into different organisms, named the binning problem. In the emerging methods for classification, deep learning is a potential technology to be applicable with high accuracy. However, it is well-known that reference databases, which are highly required by deep learning based methods, are not always available. As a result, some existing binning solutions have applied unsupervised learning processes, but utilizing the strength of deep learning in an unsupervised model is still a challenging problem. This work proposes a binning algorithm for metagenomic sequences, called MetaDEC, which applies a deep unsupervised learning approach. By following the two-phase paradigm, the algorithm firstly divides sequences into groups of overlapping sequences. The groups are then classified into clusters using an adversarial deep embedded clustering technique. Experimental results show that MetaDEC achieves competitive performance compared to existing methods on both simulated and real metagenomic data.
更多查看译文
关键词
Genomics, Clustering algorithms, Classification algorithms, Bioinformatics, Feature extraction, Sequential analysis, Organisms, Algorithm, clustering, deep learning, metagenomics, DNA sequence
AI 理解论文
溯源树
样例
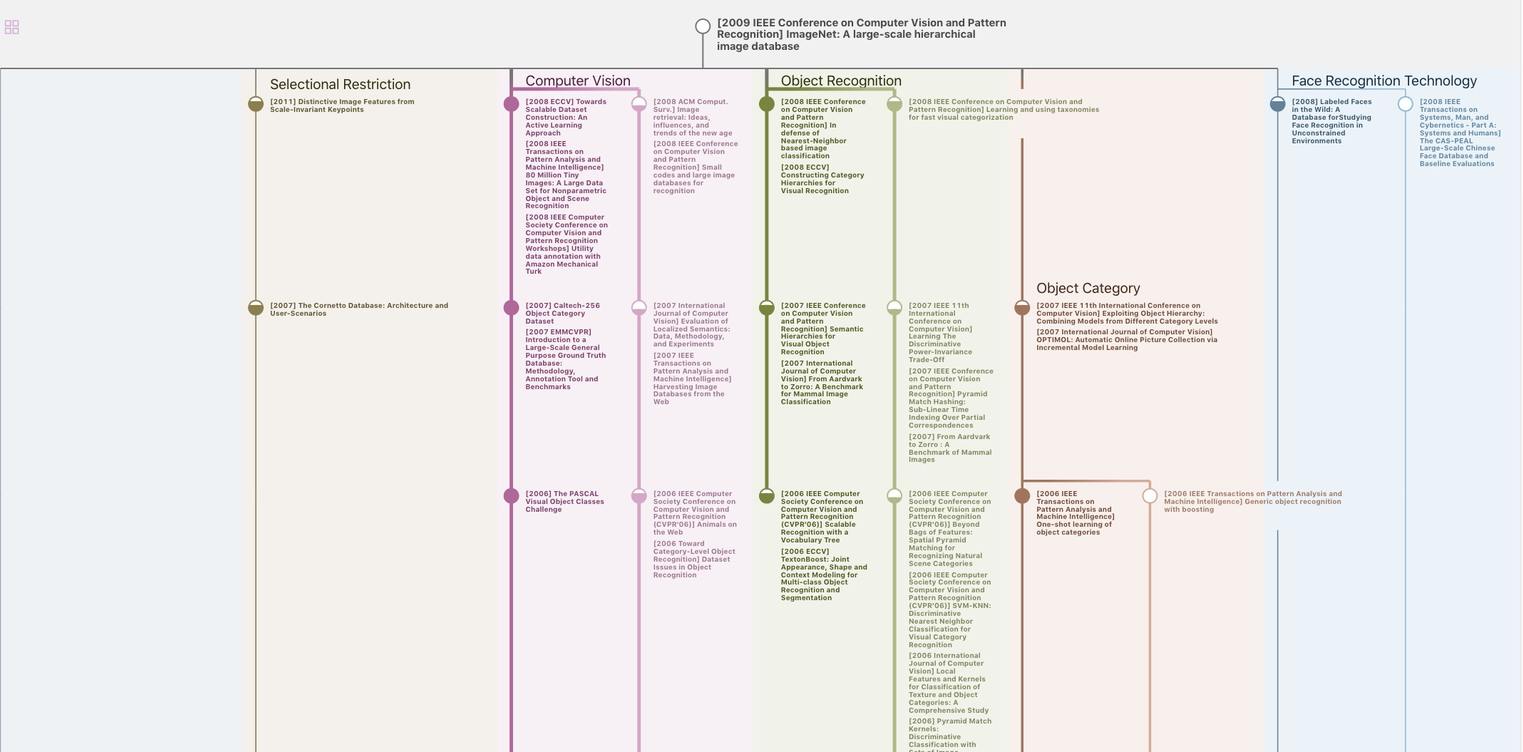
生成溯源树,研究论文发展脉络
Chat Paper
正在生成论文摘要