Deep Learning Approach for Detecting Work-Related Stress Using Multimodal Signals
IEEE Sensors Journal(2022)
摘要
Work-related stress causes serious negative physiological and socioeconomic effects on employees. Detecting stress levels in a timely manner is important for appropriate stress management; therefore, this study proposes a deep learning (DL) approach that accurately detects work-related stress by using multimodal signals. We designed a protocol that simulates stressful situations and recruited 24 subjects for the experiments. Then, we collected electrocardiogram (ECG), respiration (RESP), and video data. The datasets were pre-processed and 10-s ECG and RESP signals and a sequence of facial features were fed into our deep neural network. Sixty-eight facial landmarks’ coordinates were extracted, and facial textures were extracted from a pre-trained network based on facial expression recognition. Each signal was processed by each of its network branch, and data were fused at two different levels: 1) feature-level and 2) decision-level. The feature-level fusion that used RESP and facial landmarks’ coordinates showed average accuracy of 73.3%, AUC of 0.822, and F1 score of 0.700 in two-level stress classification, and the feature-level fusion that used ECG, RESP, and the coordinates showed average accuracy of 54.4%, AUC of 0.727, and F1 score of 0.508 in three-level stress classification. When analyzing the weights in the decision-level fusion, we found that the importance of each information item varied according to the stress classification problem. When comparing t-stochastic neighbor embedding results, we observed that overlapped samples of different classes caused performance degradation in both classifications. Our findings suggest that the proposed DL approach fusing multimodal and heterogeneous signals can enhance stress detection.
更多查看译文
关键词
Work-related stress,mental stress detection,deep learning approach,multimodality,data fusion
AI 理解论文
溯源树
样例
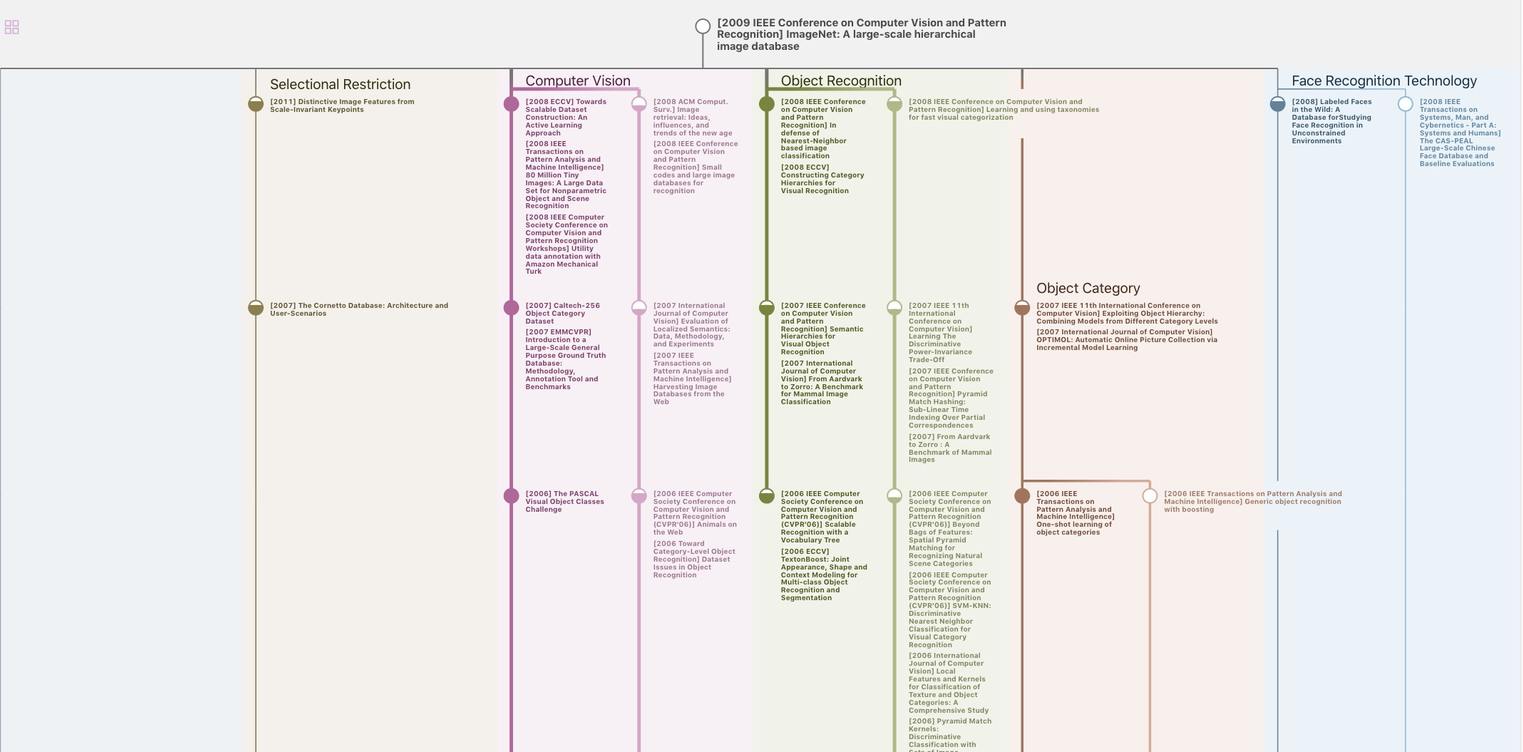
生成溯源树,研究论文发展脉络
Chat Paper
正在生成论文摘要