Dynamic Channel Selection and Transmission Scheduling for Cognitive Radio Networks
IEEE Internet of Things Journal(2022)
摘要
Cognitive radio networks (CRNs) are expected to be promising techniques for improving the spectrum efficiency of wireless network utility in the squeezed sub-6-GHz frequency bands. Nevertheless, frequency allocation and transmission scheduling for secondary users (SUs) in CRNs suffer from no prior knowledge of other SUs' network behaviors or the distribution of the amount of data generated at each SU. As a countermeasure, this article develops a protocol for the joint channel selection and transmission scheduling such that SUs with heterogeneous data transmission demands could be served with limited spectrum resources. Then, we formulate the dynamic optimization of the protocol as mutually embedded Markov decision processes (MDPs). To address the intractable MDPs, Q-learning-based channel selection and transmission scheduling based on reinforcement learning with basis function approximation are, respectively, proposed. It is shown that compared with various baselines, the proposed channel selection algorithm enables each SU to select the best frequency-domain channel that does not interfere with other SUs. In particular, the proposed transmission scheduling algorithm outperforms algorithms based on off-the-shelf approaches, such as Q-learning and Lyapunov optimization, in terms of both energy efficiency and long-term accumulative amount of bits at each SU.
更多查看译文
关键词
Dynamic scheduling,Resource management,Optimization,Approximation algorithms,Time-frequency analysis,Data communication,Sensors,Basis function approximation (BFA),cognitive radio network (CRN),mutually embedded Markov decision processes (MDPs),Q-learning,reinforcement learning (RL),resource allocation
AI 理解论文
溯源树
样例
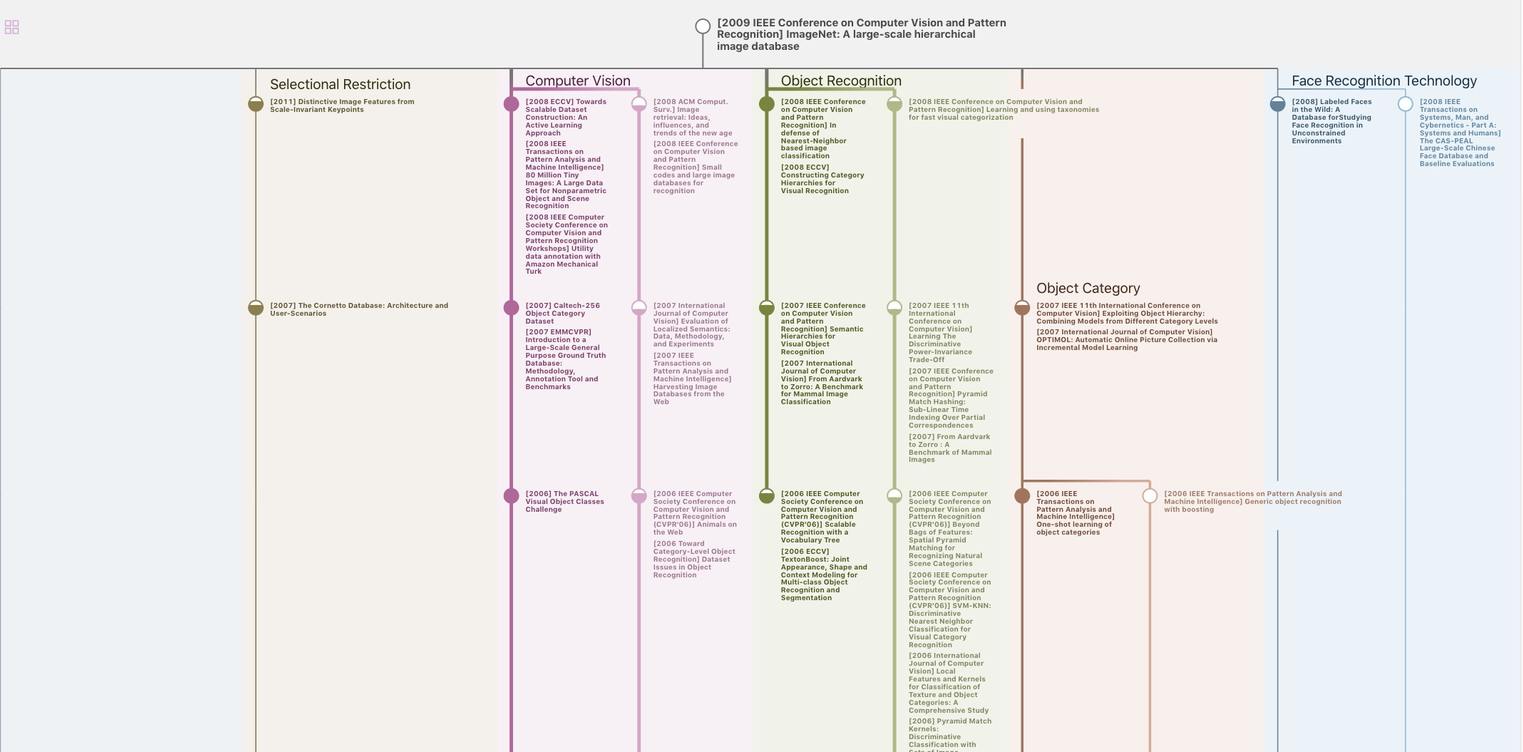
生成溯源树,研究论文发展脉络
Chat Paper
正在生成论文摘要