Multi-sphere and Multi-kernel Fuzzy Support Vector Data Description for One-class Classification
2022 7th International Conference on Intelligent Computing and Signal Processing (ICSP)(2022)
摘要
Support vector data description (SVDD) is a machine learning method for one-class classification (OCC), which is widely used in anomaly detection. However, when faced with the problem of data nonlinearity, the kernel method has difficulty in kernel parameter selection, and the lack of non-target samples completely in strict OCC tasks makes the generalization ability of the model uncertain. In addition, the nonlinearity of the data is often manifested as multi-distribution in many applications, and the data set is also at risk of being mixed with noise. Aiming at the problems above, a three-stage learning multi-sphere and multi-kernel fuzzy support vector data description (MS-MK-FSVDD) is proposed. The main idea of the method is to replace the original single spherical boundary with a set of ellipsoid boundaries that can adapt to the distribution of the data. The method first clusters the data according to the maximum posterior probability in a Gaussian mixture model (GMM). Then, for the data in each cluster, centered kernel alignment (CKA) is used to calculate the combination coefficients of the multi-kernel functions. Finally, the joint probability density function in GMM is used for weights assigning. The experiments show that MS-MK-FSVDD not only overcomes the difficulty of kernel parameter selection and enhances the anti-noise ability of the model but also improves the classification performance, especially when the data has a specific distribution.
更多查看译文
关键词
support vector data description,one-class classification,gaussian mixture model,centered kernel alignment,fuzzy weights
AI 理解论文
溯源树
样例
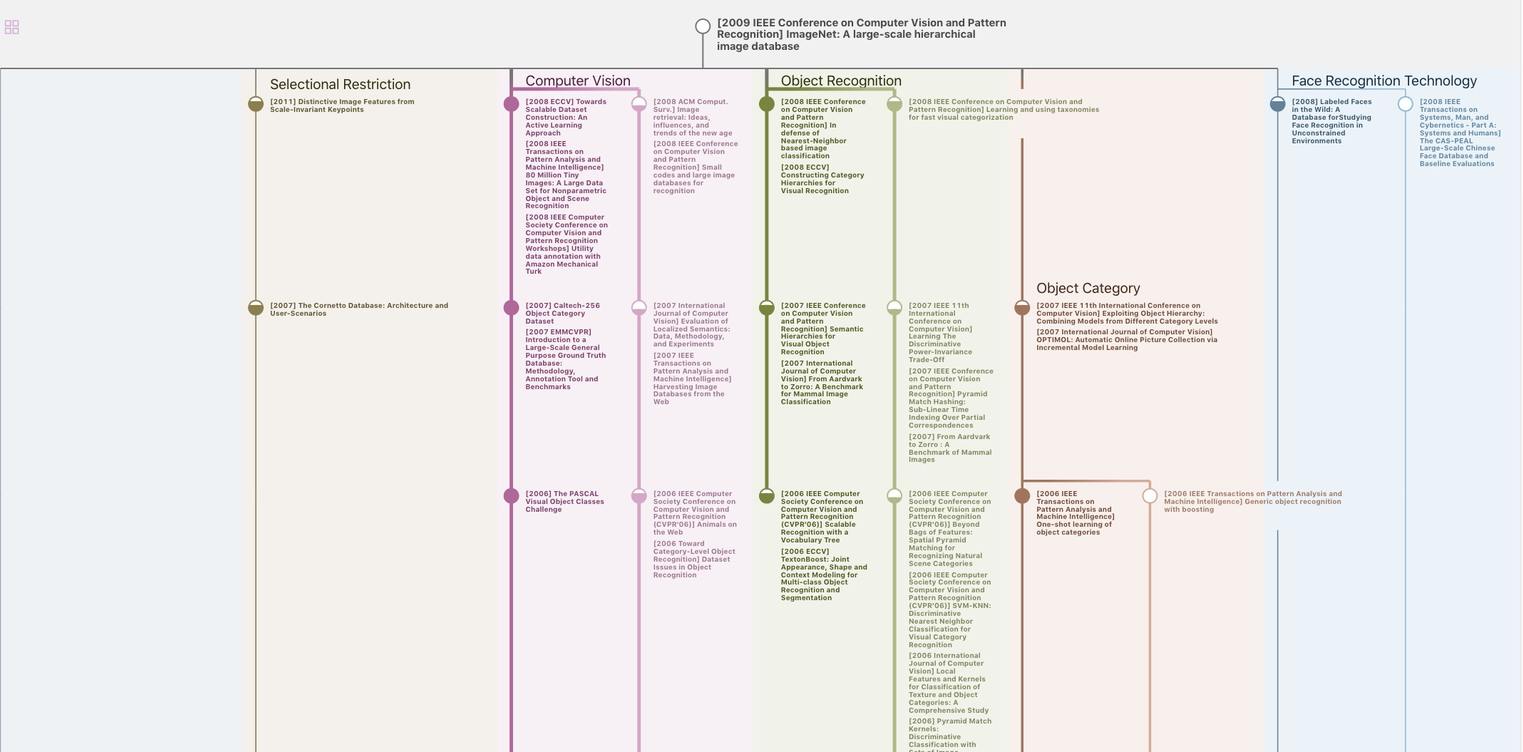
生成溯源树,研究论文发展脉络
Chat Paper
正在生成论文摘要